Unlock Fairer Outcomes: How Composite Heaviside Optimization is Changing Machine Learning
"Discover the innovative approach using Heaviside functions to tackle bias and constraints in AI decision-making, leading to more equitable results."
In an era where algorithms increasingly dictate decisions ranging from loan approvals to medical treatments, the need for fairness and ethical considerations in machine learning has never been more critical. Traditional machine learning models, while powerful, often lack the ability to incorporate real-world constraints and can perpetuate or even amplify existing biases, leading to discriminatory outcomes.
Enter Composite Heaviside Optimization, a groundbreaking approach that provides a new framework for building fairness and constraints directly into machine learning models. This innovative technique uses Heaviside functions—mathematical tools that act like on-off switches—to create models that are not only accurate but also adhere to specific rules and limitations, ensuring more equitable and just outcomes.
This article delves into the world of Composite Heaviside Optimization, explaining how it works, why it's important, and how it's being applied to solve some of the most pressing challenges in AI ethics. Whether you're a data scientist, a policy maker, or simply someone curious about the future of AI, this is your guide to understanding a technology that's poised to reshape the way we think about machine learning and fairness.
What is Composite Heaviside Optimization and Why Does It Matter?
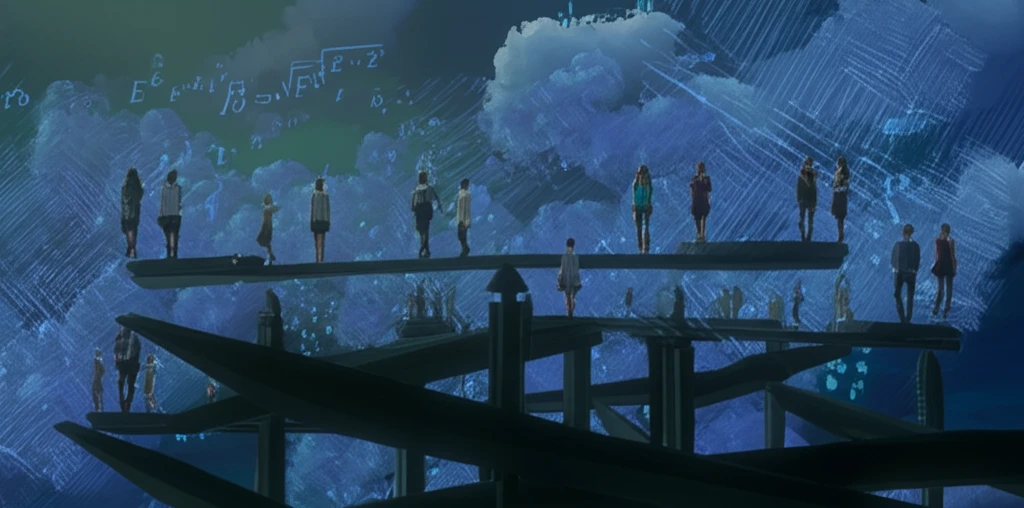
At its core, Composite Heaviside Optimization is a mathematical technique used to solve complex optimization problems where constraints and specific conditions must be met. It's particularly useful in scenarios where decisions need to be made based on multiple factors, and where certain rules or limitations must be strictly enforced.
- Fairness in Lending: Ensuring that loan applications are evaluated without bias based on race, gender, or other protected characteristics.
- Equitable Healthcare: Allocating medical resources and treatments in a way that prioritizes need and fairness.
- Impartial Criminal Justice: Developing algorithms that reduce bias in predicting recidivism rates and sentencing.
- Ethical AI in Hiring: Creating recruitment tools that evaluate candidates based on skills and qualifications, not on demographic factors.
The Future of Fairer Algorithms
Composite Heaviside Optimization represents a significant step forward in the quest for fairer and more ethical AI systems. By providing a way to directly incorporate constraints and address bias in machine learning models, this technique has the potential to transform a wide range of applications, from finance and healthcare to criminal justice and education. As AI continues to play an increasingly important role in our lives, tools like Composite Heaviside Optimization will be essential for ensuring that these technologies are used in a way that promotes equity, justice, and the well-being of all members of society. The journey toward truly fair and ethical AI is just beginning, but Composite Heaviside Optimization offers a promising path forward.