Unlock Efficiency: How Conic Optimization is Revolutionizing Problem-Solving
"Discover the power of conic optimization (CO) – a sophisticated yet accessible method transforming industries from finance to logistics."
In today's fast-paced world, businesses and organizations are constantly seeking innovative tools to optimize their operations, streamline decision-making, and solve complex problems. Linear programming (LP) has long been a staple, but a more versatile and powerful approach is gaining traction: conic optimization (CO). Although it sounds technical, conic optimization offers practical solutions across diverse fields, from managing investment portfolios to optimizing supply chains.
Conic optimization is a mathematical method that generalizes linear programming, making it suitable for problems that can't be accurately represented with simple linear equations. It provides a framework for dealing with non-linear relationships and complex constraints, reflecting the real-world scenarios that businesses often encounter. Think of it as upgrading from a basic calculator to a scientific one – it handles more complex calculations with greater precision.
This article serves as a user-friendly guide to conic optimization, breaking down its key concepts, showcasing its applications, and explaining why it's becoming an essential tool for professionals in operations research, data science, and beyond. Whether you're an analyst, a manager, or simply someone curious about advanced problem-solving techniques, this guide will provide valuable insights into the world of conic optimization.
What Exactly is Conic Optimization?
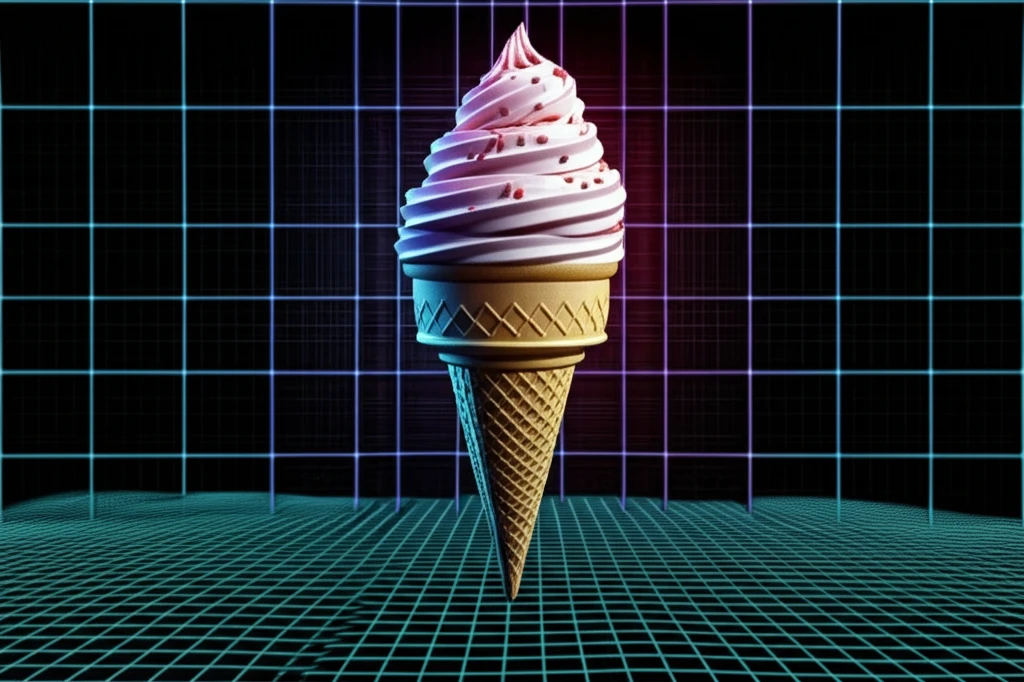
At its core, conic optimization involves optimizing a linear function over the intersection of a hyperplane and a 'proper cone'. Don't let the jargon intimidate you! In simpler terms, imagine you're trying to find the best possible solution (like maximizing profit or minimizing cost) within a set of constraints. These constraints define a feasible region, and instead of being limited to straight lines and flat surfaces (as in linear programming), conic optimization allows for curved boundaries and more complex shapes.
- Risk management in finance: Optimizing investment portfolios while considering various risk factors.
- Engineering design: Ensuring structural stability and performance.
- Logistics and supply chain management: Minimizing transportation costs and optimizing delivery routes.
The Future of Optimization
Conic optimization is more than just a mathematical technique; it's a powerful framework for addressing real-world challenges with greater precision and efficiency. As software and algorithms continue to improve, CO is poised to become an indispensable tool for businesses and organizations seeking a competitive edge. Embracing CO means unlocking new possibilities for optimization, innovation, and data-driven decision-making.