Unlock Economic Insights: A Beginner's Guide to Vector Autoregression (VAR) Models
"Demystifying complex economic relationships with VAR models, understand how these models predict and analyze the interplay of economic indicators, and gain practical knowledge to interpret macroeconomic data with confidence."
In today's data-driven world, understanding how different economic factors interact is more important than ever. Whether you're an investor, a policy maker, or simply a curious citizen, the ability to interpret macroeconomic data and predict economic trends can provide a significant edge. Enter Vector Autoregression (VAR) models: a statistical technique used to capture the complex interdependencies between multiple time series.
VAR models are particularly useful in macroeconomics because they allow economists to analyze the dynamic relationships between key economic indicators. Unlike traditional methods that require pre-defined causal structures, VAR models treat all variables as endogenous, meaning that they are all mutually influenced by each other. This makes VAR models ideal for exploring the intricate feedback loops that characterize the economy.
This article aims to demystify VAR models, providing you with a comprehensive yet accessible guide to their underlying principles, applications, and interpretation. We'll break down complex concepts into easy-to-understand terms, equipping you with the knowledge and confidence to navigate the world of macroeconomic analysis.
What are Vector Autoregression (VAR) Models?
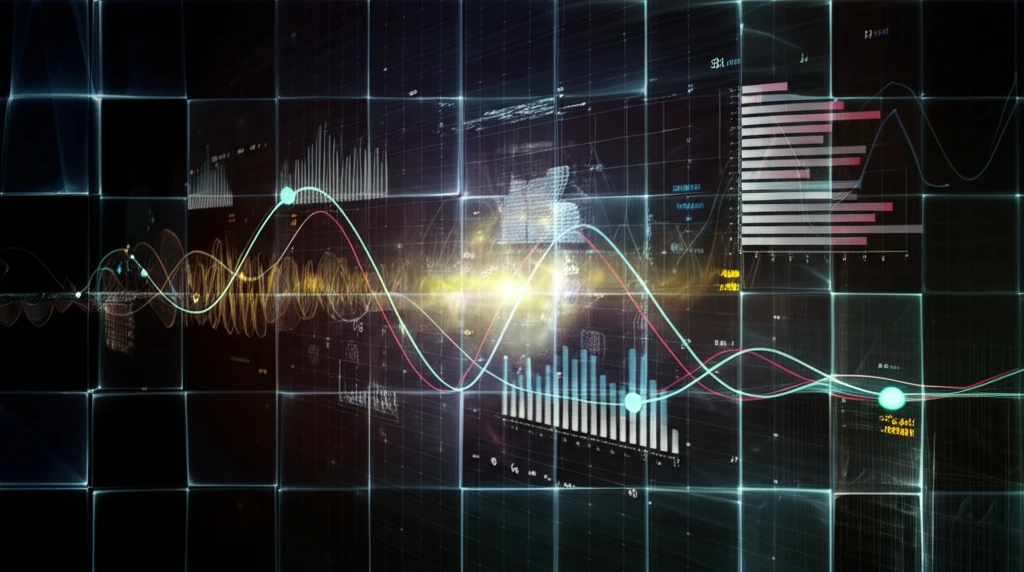
At its core, a VAR model is a statistical model used to capture the relationships between multiple time series by analyzing how each variable evolves based on its own past values and the past values of other variables in the system. Think of it as a way to model how different economic indicators “talk” to each other over time.
- Multiple Time Series: VAR models involve several variables that are measured over time, such as GDP growth, inflation rates, unemployment figures, and interest rates.
- Endogenous Variables: Unlike some other statistical models, VAR models treat all variables as endogenous, meaning they are all mutually influenced by each other. There is no need to specify in advance which variables are independent and which are dependent.
- Lagged Values: The model uses lagged values of each variable to predict its future values. The number of lags included is a key parameter of the model.
- System of Equations: A VAR model consists of a system of equations, with each equation representing the evolution of one of the variables in the system.
Next Steps: Diving Deeper into VAR Model Analysis
Vector Autoregression models provide a powerful framework for analyzing and forecasting macroeconomic variables. By understanding the core principles, applications, and limitations of VAR models, you can gain a competitive edge in interpreting economic data, predicting market trends, and evaluating policy implications. As you continue to explore the world of VAR models, remember to stay curious, embrace the complexity, and never stop learning.