Trim the Fat: How Halfspace Depth Can Help You Find the Core of Your Data
"Uncover the power of halfspace depth trimmed means in multivariate analysis, making complex data more understandable and manageable."
In a world awash with data, finding meaningful insights can feel like searching for a needle in a haystack. Traditional statistical methods often fall prey to the distorting influence of outliers – those pesky data points that lie far from the norm, skewing results and leading to misguided conclusions. But what if there were a way to 'trim the fat,' focusing on the core of your data to reveal the underlying truth? That's where trimmed means come in, offering a more robust and reliable approach to data analysis.
Imagine you're calculating the average income in a neighborhood, and one resident is a billionaire. Their income would drastically inflate the average, misrepresenting the financial reality for the majority. A trimmed mean solves this by discarding a certain percentage of the highest and lowest values before calculating the average. This way, extreme values have less impact, providing a more accurate reflection of the typical income.
While trimmed means are a familiar concept in one-dimensional data, extending them to multiple dimensions presents a unique challenge. Enter the 'halfspace depth trimmed mean,' a sophisticated technique that brings the benefits of trimming to complex, multivariate datasets. This method, rooted in the concept of 'halfspace depth,' provides a way to order data points from the center outwards, allowing us to identify and remove outliers in a meaningful way.
Understanding Halfspace Depth and Trimmed Means
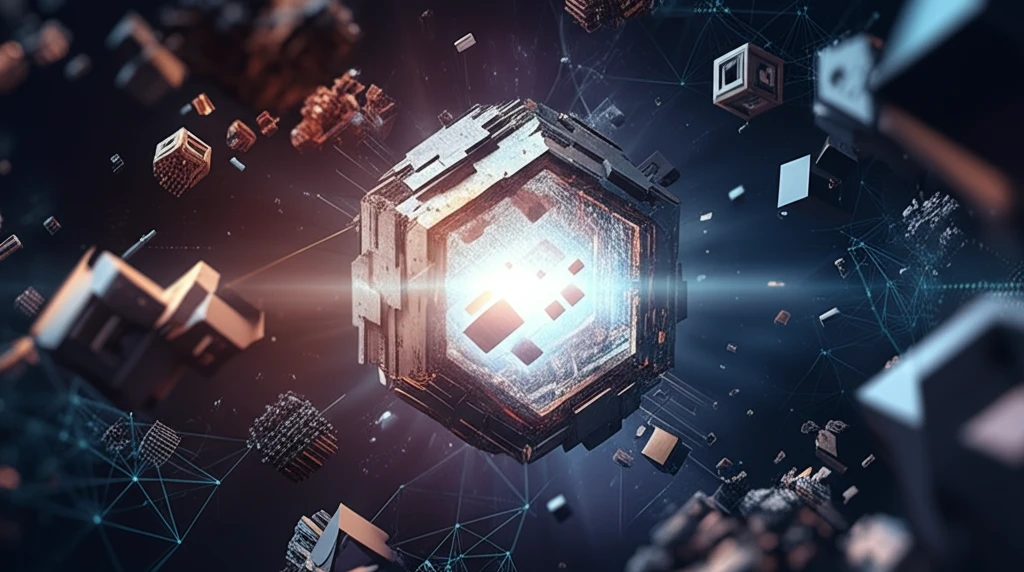
At its heart, the halfspace depth function is a measure of how 'central' a point is within a dataset. Imagine drawing a line through your data – the halfspace depth of a point is related to the smallest proportion of data points that lie on one side of any such line. Points deep within the data cloud have high halfspace depth, while outliers clinging to the edges have low depth. This creates a natural ordering, allowing us to define a 'depth trimmed region' – a subset of the data containing only the most central points.
- Resilience to Outliers: By discarding points with low halfspace depth, trimmed means minimize the impact of extreme values, leading to more stable and reliable results.
- Improved Accuracy: Focusing on the core of the data reduces noise and distortion, revealing underlying trends with greater clarity.
- Flexibility: The 'trimming percentage' can be adjusted to balance robustness and efficiency, allowing you to tailor the method to your specific data and analysis goals.
- Applicability to Multivariate Data: Unlike many traditional methods, halfspace depth trimmed means can be applied to datasets with multiple variables, making them ideal for complex real-world problems.
The Future of Robust Data Analysis
As data continues to grow in volume and complexity, robust statistical methods like halfspace depth trimmed means will become increasingly important. By providing a way to filter out noise and focus on the essential signal, these techniques empower us to make better decisions, uncover hidden patterns, and gain a deeper understanding of the world around us. So, embrace the power of trimming – it might just be the key to unlocking the truth hidden within your data.