The Unexpected Twist in Risk Management: Why Diversification Might Not Always Save You
"Pareto distributions, infinite means, and the surprising pitfalls of diversification in a volatile world."
In the complex world of finance and risk management, the Pareto distribution stands as a crucial model, especially when dealing with heavy-tailed loss distributions. Its connection to regularly varying tails, Extreme Value Theory (EVT), and power laws makes it indispensable for understanding phenomena across economics and social networks. But what happens when the conventional wisdom of diversification faces a statistical anomaly?
A recent study uncovers a surprising inequality: the weighted average of independent and identically distributed Pareto random variables with infinite mean is actually larger than a single such random variable when assessed using first-order stochastic dominance. This counter-intuitive result challenges our fundamental understanding of risk management and diversification, with far-reaching implications for financial institutions, insurance companies, and anyone dealing with potential high-impact losses.
Pareto distributions help us model various real-world events, from catastrophic losses like earthquakes and hurricanes to wealth distribution and operational risks in finance. Understanding the nuances of these distributions, especially in cases where traditional measures like the mean become infinite, is critical for making informed decisions. This article explores this unexpected behavior, its underlying mechanisms, and its practical consequences.
What Makes Pareto Distributions So Important in Understanding Risk?
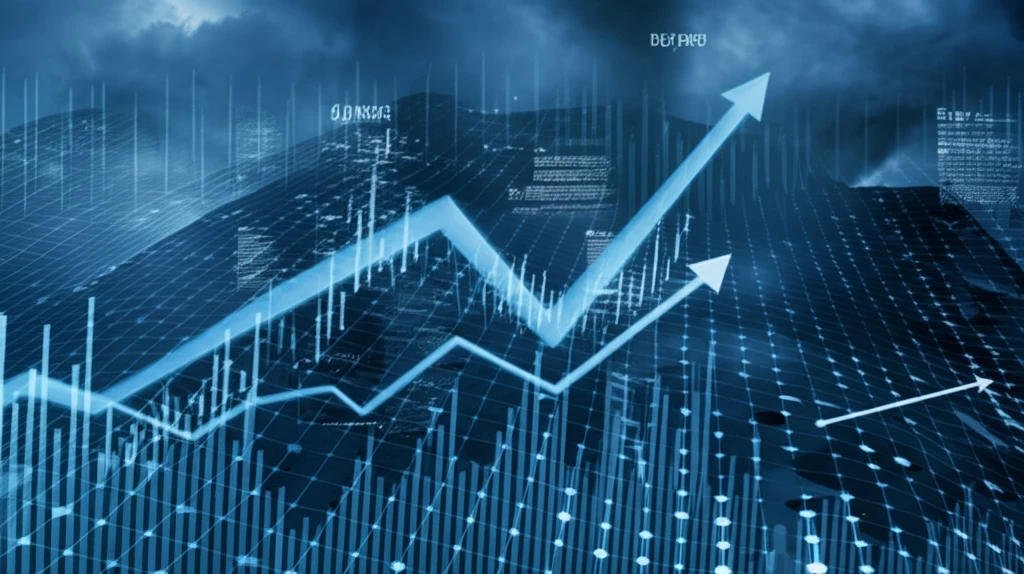
Pareto distributions stand out because they effectively capture extreme events and heavy tails, which are common in many real-world scenarios. Unlike normal distributions, which assume events cluster around an average, Pareto distributions acknowledge that significant outliers can occur with surprising frequency. This is particularly relevant in:
- Catastrophic Risks: Modeling losses from earthquakes, hurricanes, and wildfires where the impact can be devastatingly large.
- Financial Markets: Representing wealth distribution, financial asset losses, and operational risks.
- Technological Innovation: Analyzing financial returns from technological advancements that can disrupt markets.
- Insurance: Evaluating potential losses in insurance contexts.
The Future of Risk Assessment: Adapting to the Unexpected
The findings presented in this research highlight the need for a more nuanced approach to risk management, especially when dealing with heavy-tailed distributions and infinite mean scenarios. Traditional diversification strategies may not always provide the protection expected, urging financial professionals and policymakers to reconsider their models and assumptions. As we navigate an increasingly uncertain world, understanding these statistical subtleties is essential for building more resilient and robust financial systems.