Taming Uncertainty: How Stochastic Programming Optimizes a World in Flux
"Navigate the complexities of real-world decisions with stochastic programming, a powerful method for handling uncertainty and optimizing outcomes."
The modern world is characterized by constant change and pervasive uncertainty. From fluctuating market conditions and unpredictable consumer behavior to supply chain disruptions and geopolitical instability, businesses and organizations across all sectors face a barrage of unknowns that can significantly impact their operations and strategic goals. Traditional optimization methods, which rely on fixed parameters and deterministic assumptions, often fall short in such dynamic environments, leading to suboptimal decisions and increased risks.
A powerful and versatile approach for navigating this complexity is stochastic programming (SP). Unlike deterministic optimization, which seeks the best solution under a single, predefined scenario, SP explicitly incorporates uncertainty into the decision-making process. It achieves this by modeling uncertain parameters as random variables with associated probability distributions, allowing for a range of possible future outcomes to be considered.
This framework enables decision-makers to identify robust strategies that perform well across a spectrum of scenarios, rather than being optimized for just one particular outcome. This is particularly valuable in situations where the cost of making a wrong decision is high, or when adaptability and resilience are paramount.
The Essence of Stochastic Programming
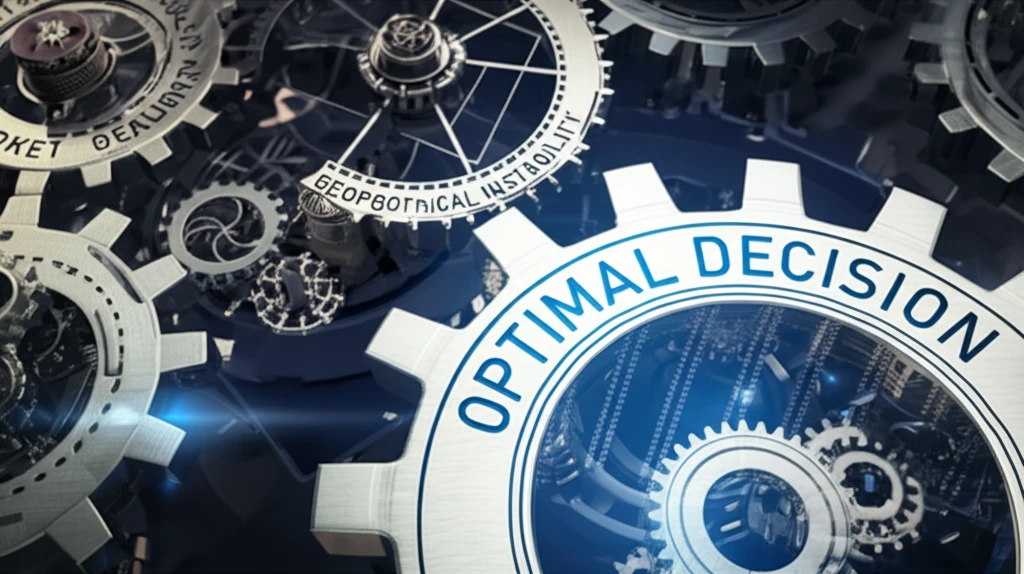
At its core, stochastic programming is a blend of optimization techniques and probability theory. It begins by identifying the key sources of uncertainty in a given problem, such as future demand, resource availability, or market prices. These uncertain parameters are then modeled as random variables, characterized by probability distributions that reflect their possible range of values and likelihoods. This step requires careful analysis and data gathering, potentially involving statistical modeling and expert elicitation.
The Future of Decision-Making
As the world becomes increasingly interconnected and unpredictable, the need for robust and adaptable decision-making tools will only intensify. Stochastic programming offers a powerful framework for navigating uncertainty and optimizing outcomes in a wide range of applications. By embracing this approach, organizations can enhance their resilience, improve their performance, and gain a competitive edge in a dynamic and ever-changing world.