Taming Randomness: How Particle Methods Revolutionize Stochastic Equations
"Explore the power of particle representations in solving stochastic partial differential equations and uncover their applications in various scientific fields."
In numerous scientific fields, understanding systems influenced by randomness is crucial. These systems are often described by stochastic partial differential equations (SPDEs), which can be incredibly challenging to solve. However, recent advancements in particle representations offer a powerful new approach.
SPDEs are central to modeling diverse phenomena, including weather patterns, financial markets, and material science. The Allen-Cahn equation, for example, is a well-known SPDE that models phase separation in alloys. Another SPDE governs the stochastic quantization of Euclidean quantum field theory. The common thread is that these SPDEs all include randomness, requiring sophisticated methods to analyze their behavior.
This article explores how particle representations offer a way to tame the randomness in SPDEs. We will break down the complex research and showcase how these techniques are opening new possibilities for modeling and simulating complex systems. We'll explore the theoretical underpinnings and practical applications of this exciting area of research.
What Are Particle Representations and Why Do They Matter?
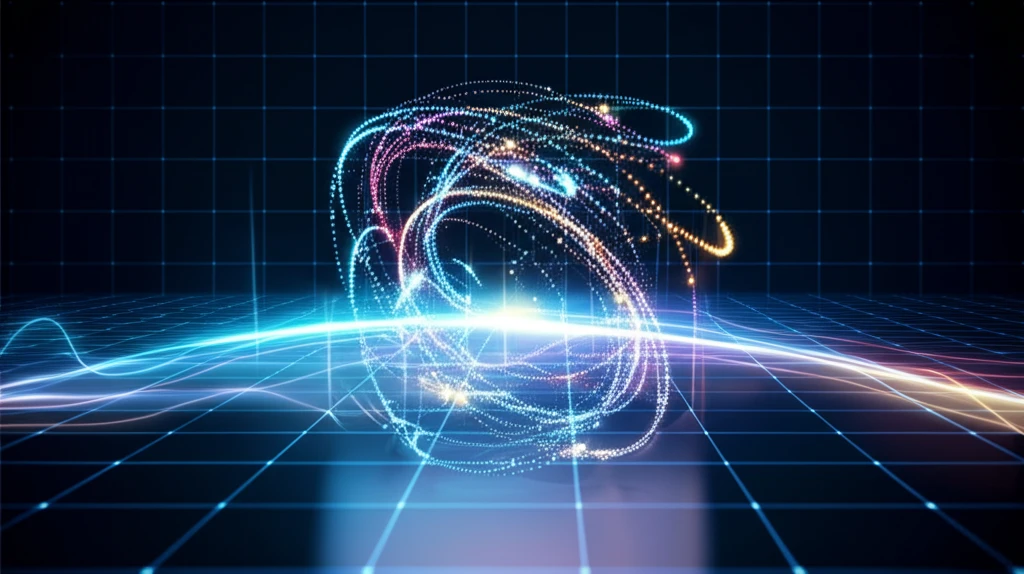
The essence of particle representations lies in approximating the solution of an SPDE using a collection of individual "particles." Think of these particles as individual agents, each following its own probabilistic rules. Crucially, these particles interact with each other, and their collective behavior provides an approximation to the solution of the SPDE.
- Key Advantages:
- Computational Efficiency: Particle methods can handle high-dimensional problems more efficiently than traditional methods.
- Flexibility: They adapt well to different types of SPDEs and boundary conditions.
- Intuitive Interpretation: The particle-based approach offers a clear, visual way to understand complex system behavior.
The Future of Stochastic Modeling
Particle representations are poised to play an increasingly important role in solving complex stochastic problems. As computational power continues to grow and new theoretical insights emerge, these methods will unlock a deeper understanding of systems governed by randomness.