Stress Less, Classify More: How AI Feature Selection Can Help You Relax
"A Comparative Study Reveals the Best Methods for Stress Hotspot Classification in Materials, Making Engineering Simpler"
In the fast-evolving world of materials science, accurately identifying stress hotspots is crucial for ensuring the durability and safety of various products, from aircraft wings to medical implants. Traditional methods of stress analysis can be time-consuming and computationally intensive. Fortunately, the rise of artificial intelligence (AI) offers a promising avenue for improving these processes.
AI-driven feature selection methods are changing how engineers classify stress hotspots in materials. By automating the identification of the most critical features, these methods reduce analysis time and improve accuracy. A recent comparative study has shed light on the effectiveness of different AI techniques, providing practical guidance for engineers and materials scientists.
This article delves into the findings of this groundbreaking study, explaining how AI is transforming stress analysis. We'll explore the best feature selection methods, how they work, and their real-world applications. Whether you're an engineer, a student, or simply curious about the future of materials science, this guide will equip you with valuable insights.
Why Feature Selection Matters for Stress Hotspot Classification
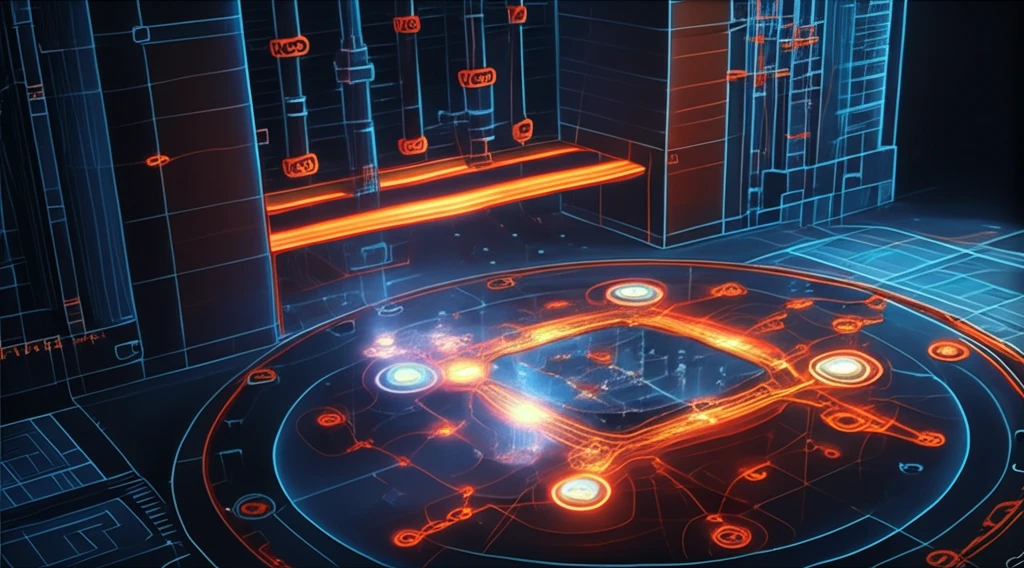
Stress hotspots are areas within a material that experience significantly higher stress concentrations than their surroundings. Identifying these hotspots is essential because they are often the points where failures initiate. Traditional methods involve extensive simulations and experimental testing, which can be both costly and time-consuming. The goal of feature selection is to pinpoint the most relevant characteristics or parameters that contribute to stress concentration, reducing the complexity of the analysis.
- Reduced Complexity: Simplifies the analysis by focusing on the most important variables.
- Improved Accuracy: Enhances the precision of stress predictions by eliminating irrelevant data.
- Cost Savings: Reduces the need for extensive simulations and experimental testing.
- Time Efficiency: Accelerates the identification of stress hotspots.
The Future of Stress Analysis: Embracing AI
As AI technology continues to advance, its role in materials science and engineering will only grow. By embracing AI-driven feature selection methods, engineers can unlock new levels of efficiency, accuracy, and innovation in stress analysis. This not only leads to safer and more durable products but also paves the way for groundbreaking discoveries in materials science. The journey toward smarter, more reliable stress analysis is just beginning, and the possibilities are endless.