Stratified Experiments: How to Boost Accuracy in Your Research
"Discover the power of covariate adjustment in stratified experiments. Learn how to refine your research and achieve more reliable results."
In the world of research, accuracy is everything. Whether you're studying the effects of a new drug, analyzing consumer behavior, or evaluating the impact of a social program, you need to be confident that your findings are reliable and precise. That's where stratified experiments and covariate adjustment come into play. These techniques can help you refine your research and achieve more accurate results, but they can also be complex and challenging to implement correctly.
Imagine you're conducting a study to determine the effectiveness of a new educational program. You randomly assign students to either participate in the program or continue with their regular curriculum. However, you know that students come from diverse backgrounds and have varying levels of prior academic achievement. If you don't account for these differences, they could skew your results and lead you to draw inaccurate conclusions.
This guide demystifies the process of covariate adjustment in stratified experiments, providing you with practical insights and actionable strategies to enhance the precision of your research. We'll explore common pitfalls and offer solutions that ensure your data analysis is both robust and reliable. Get ready to take your research to the next level!
Why Stratification and Covariate Adjustment Matter
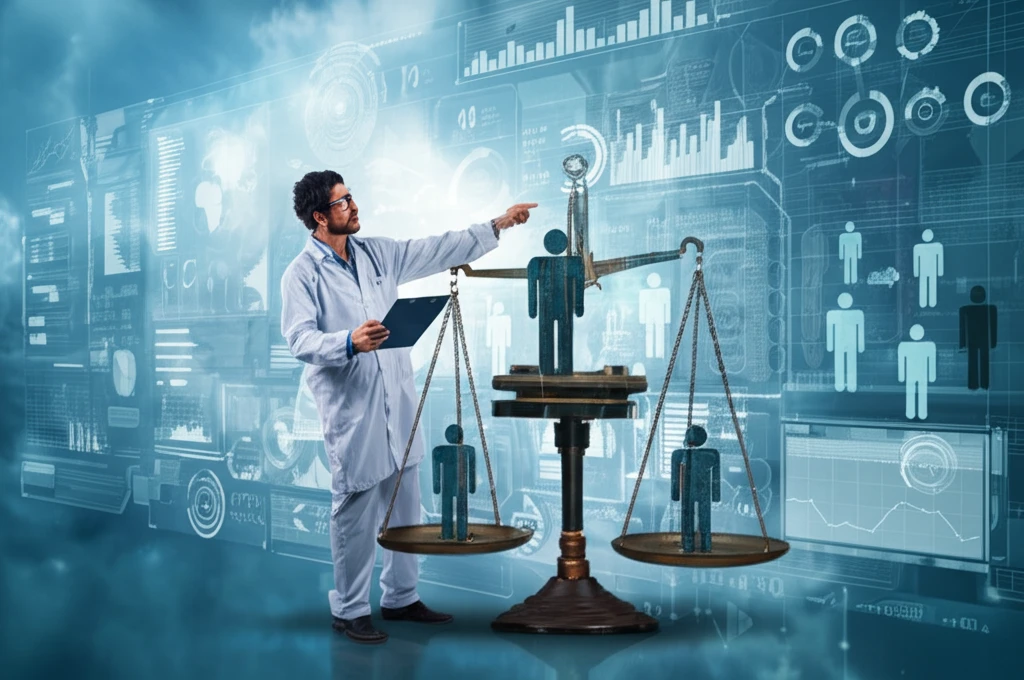
Stratified experiments involve dividing your study population into subgroups, or strata, based on shared characteristics. This is a powerful way to ensure that your sample is representative of the broader population and that you can account for key differences between groups. For instance, in our education program example, you might stratify your sample by socioeconomic status, prior academic performance, or geographic location.
- Improved Precision: By accounting for differences between subgroups, you can reduce the amount of variability in your results and obtain more precise estimates of the effects you're studying.
- Reduced Bias: Controlling for confounding variables helps to eliminate bias and ensure that your findings are accurate and reliable.
- Increased Statistical Power: By reducing noise in your data, you can increase the statistical power of your study, making it more likely that you'll detect meaningful effects.
- Generalizability: If your sample is properly stratified, the results are more generalizable to a wide audience.
Optimizing Your Research
By mastering the techniques of covariate adjustment within stratified experiments, you are setting yourself up for more reliable and impactful research outcomes. Be thoughtful in design, diligent in execution, and always ready to adapt. Your journey toward precision begins with a single, well-adjusted experiment. The insights gleaned can be transformative, impacting not only your immediate field of study, but potentially offering broader applications across various disciplines.