Spine Surgery Tech: Which Guided Method Reigns Supreme?
"A breakdown of robotic, navigation template, O-arm, and fluoroscopy-guided approaches to pedicle screw insertion."
Spine surgery has undergone a tech revolution, with robotic assistance emerging as a promising tool. While it offers benefits like enhanced precision, questions remain about how it compares to other guided methods in treating lumbar degenerative disease.
A recent study published in "Medical Science Monitor" dives into this comparison, evaluating the accuracy of pedicle screw insertion using four different guided technologies: spine robots, navigational templates, O-arm-based navigation, and fluoroscopy-guided assistance.
This article breaks down the study's findings, exploring the advantages and disadvantages of each approach to help you understand the latest advancements in spine surgery tech.
Robotic vs. Traditional: Understanding Spine Surgery Methods
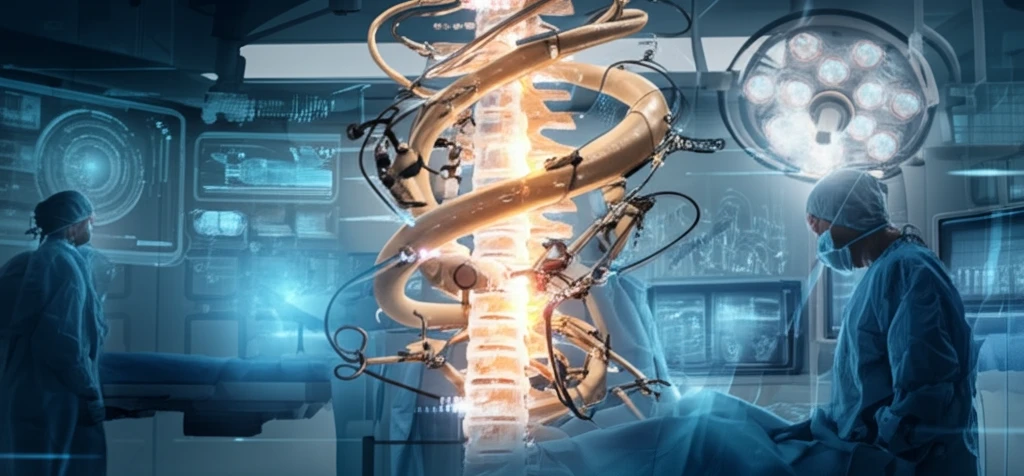
The study meticulously analyzed 176 pedicle screws inserted into 39 patients using a spine robot, comparing them against screws placed with navigational templates (134 screws in 28 patients), O-arm-based navigation (234 screws in 51 patients), and fluoroscopy-guided assistance (346 screws in 72 patients).
- Spine Robot (Group 1): Relies on preoperative CT scans and intraoperative imaging for precise screw placement.
- Navigational Template (Group 2): Uses a patient-specific template created from CT data to guide screw insertion.
- O-arm-Based Navigation (Group 3): Employs real-time imaging during surgery to track instruments and guide screw placement.
- Fluoroscopy-Guided Assistance (Group 4): Utilizes X-ray imaging to visualize the spine during surgery, guiding screw insertion.
The Future of Guided Spine Surgery
While robotic assistance shows promise in specific areas like reducing complications and fluoroscopy time, the study suggests that navigational templates and O-arm-based navigation offer comparable accuracy for pedicle screw insertion. As technology evolves, the choice of guided method will likely depend on individual patient needs, surgeon expertise, and the specific goals of the surgery.