Speak Up! How AI Face Analysis Can Boost Your Public Speaking Game
"Discover how researchers are using AI to bridge the gap between facial recognition and voice analysis, improving speaker performance in unexpected ways."
In an era where effective communication is more critical than ever, researchers are constantly seeking new ways to enhance how we speak and engage with one another. A groundbreaking study introduces a novel approach to improve speaker modeling by leveraging knowledge transferred from facial representation. This interdisciplinary method aims to create a more discriminative metric, allowing speaker turns to be compared directly, which is beneficial for tasks such as diarization and dialogue analysis.
The study focuses on enhancing the embedding space of speaker turns by applying maximum mean discrepancy loss. This technique minimizes the disparity between the distributions of facial and acoustic embedded features. By uncovering the shared underlying structure of these two seemingly distinct embedded spaces, the approach enables the transfer of knowledge from richer face representations to their speech counterparts. This method promises to refine how machines understand and process human interaction, offering potential improvements across various applications.
The implications of this research extend beyond academic circles. Imagine AI systems that can better discern who is speaking in a crowded room, understand the nuances of a conversation, or even help individuals improve their public speaking skills by analyzing their facial expressions and vocal tones in tandem. As AI continues to evolve, the integration of multimodal data—like faces and voices—will become increasingly important. This study marks a significant step forward in achieving more natural and intuitive human-computer interaction.
Decoding the Science: How Face Data Can Help Your Voice
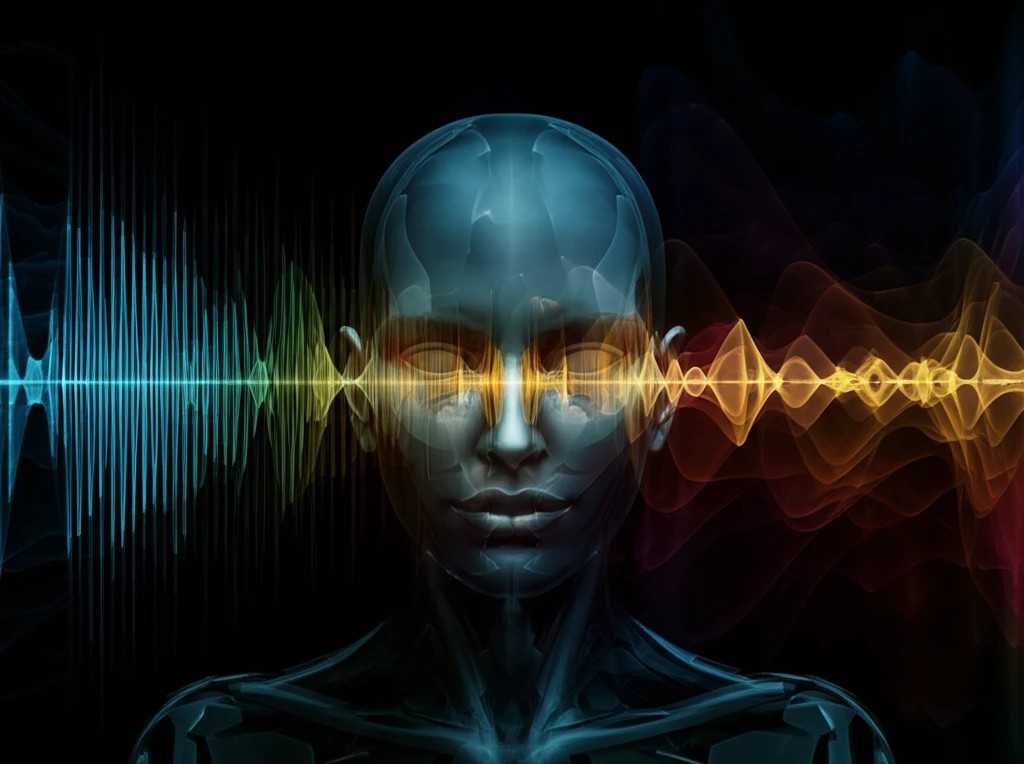
At its core, the research addresses a significant challenge in speech technology: accurately modeling and differentiating between different speakers, especially in noisy or complex environments. Traditional methods often struggle with short speaker turns or limited training data. To overcome these hurdles, researchers have turned to facial recognition technology, which has seen remarkable advancements in recent years. The idea is that by analyzing facial expressions and movements, AI can glean additional information that complements and enhances voice analysis.
- Improved Accuracy: By integrating facial data, the system can more accurately identify and differentiate between speakers, even when speech segments are short or noisy.
- Better Performance with Limited Data: The approach is particularly effective when training data is scarce, leveraging the wealth of information available from face recognition models.
- Enhanced Generalization: The AI can better generalize across different environments and speaking styles, leading to more robust and reliable performance in real-world scenarios.
The Future is Multimodal: What This Means for You
This research underscores the growing importance of multimodal learning in AI. By combining different sources of information, such as faces and voices, AI systems can achieve a more comprehensive understanding of human behavior and communication. For end-users, this translates to more intuitive and effective technologies. Whether it's improving the accuracy of voice assistants, enhancing video conferencing experiences, or developing new tools for language learning, the possibilities are endless. As AI continues to evolve, expect to see more innovations that bridge the gap between different modalities, creating a more seamless and natural interaction between humans and machines.