Smarter Nuclear Data: How Adaptive Monte Carlo Could Revolutionize Safety Simulations
"Traditional nuclear simulations face limitations; adaptive methods offer a path to more accurate and reliable safety assessments"
Computer simulations are vital for guiding the development of nuclear facilities. These simulations estimate critical factors like atomic element production and neutron multiplication rates, optimizing design for both efficiency and safety. High-quality, evaluated nuclear data, typically in the form of an ENDF file, is essential for these simulations. When the ENDF file includes covariance matrices, it allows for uncertainty propagation through perturbation theory, providing valuable insights into the reliability of simulation results.
However, perturbation theory has a fundamental limitation: it assumes that both evaluated nuclear data and simulation results have Gaussian-shaped uncertainties. This assumption may not hold true due to the non-linear nature of nuclear models and simulations. These non-linearities can lead to skewed distributions with multiple peaks, making Gaussian approximations inadequate. Relying on Gaussian distributions in such cases can underestimate the probability of rare events or misrepresent the likelihood of certain parameter ranges.
Monte Carlo methods offer an alternative by allowing the use of more realistic, non-Gaussian distributions. These methods use samples of model parameter sets and corresponding model predictions to extract mean values, uncertainties, and other important quantities. Despite the practical success, these methods are computationally intensive.
Adaptive Monte Carlo: A Faster Route to Precision
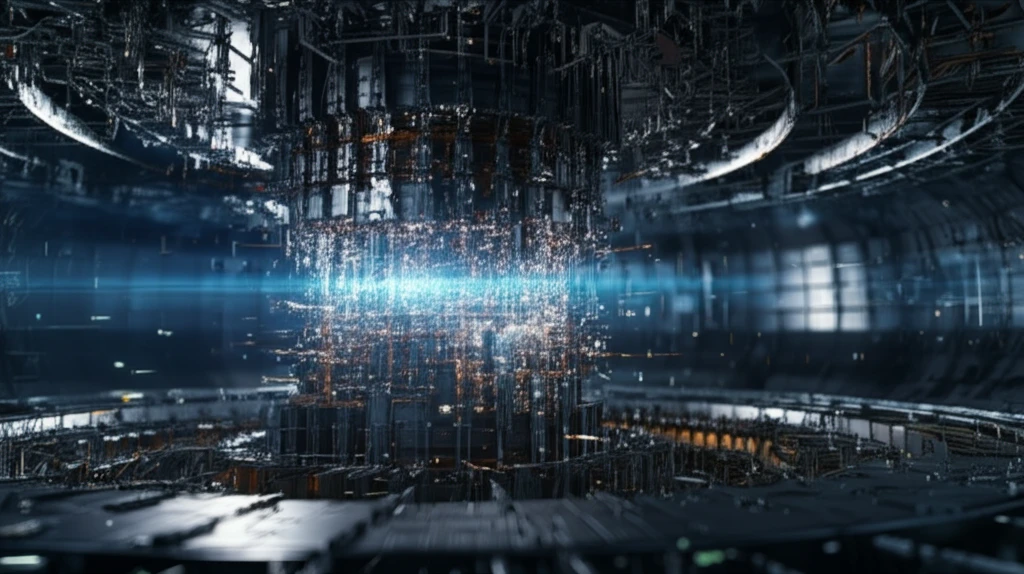
Recognizing the computational demands of traditional Monte Carlo methods, researchers are exploring adaptive Monte Carlo schemes. These schemes incorporate fast evaluation techniques that linearize nuclear models. This helps adapt the sampling distribution to better approximate the posterior distribution. This is key to accelerate convergence and reduce execution time, making the simulations more practical.
- Initialization: Set up an initial sampling distribution, often based on prior knowledge.
- Sampling and Weighting: Generate parameter vectors and calculate weights based on the posterior distribution.
- Learning Step: Evaluate the effective sample size and, if it's below a threshold, update the sampling distribution.
- Iteration: Repeat the sampling and learning steps until the effective sample size reaches a target value.
The Future of Nuclear Simulations
The adaptive Monte Carlo method shows promise for enhancing nuclear data evaluation. By efficiently adapting the sampling distribution, it can provide more accurate and reliable uncertainty estimates. This can be crucial for the safety and efficiency of nuclear facilities. Future research will focus on evaluating the method with more complex models and a larger number of parameters. Applications may include the Total Monte Carlo method, treatment of model defects, and constraining model parameters using both differential and integral observables. This has the potential to significantly improve the accuracy and reliability of nuclear simulations, leading to safer and more efficient nuclear technologies.