Smart Water: How AI Could Be Fixing Leaks Before They Start
"AI-powered iterative learning control offers a new approach to managing water pressure, minimizing leaks, and conserving resources in distribution networks."
Water scarcity is a growing global concern, exacerbated by significant water loss due to leaks in distribution networks. Traditional methods of pressure management have proven to be effective in reducing leaks and bursts, but a new approach is needed to integrate advanced technologies for smart and efficient water resource management. With pressure too low, end-users don't get the water they expect, in turn leading to pollutants entering the network.
Research into water distribution systems has largely focused on optimal pump scheduling, leakage detection, and contamination prevention. One promising area is the use of iterative learning control (ILC), a technique that leverages past data to refine control actions and improve system performance over time. By applying ILC to pressure control, we can meet pressure requirements at critical points in the network while minimizing unnecessary pressure levels.
Iterative Learning Control (ILC) offers a dynamic approach to refining control inputs. This technique is beneficial for managing systems such as mechanical robots or production lines and is now being applied to enhance smart pumping station control. Iterative learning uses logged pressure data to calculate the most efficient pressure, reducing reliance on internal models. It's particularly effective because water usage often follows predictable patterns.
The AI Model: Reducing Water Loss
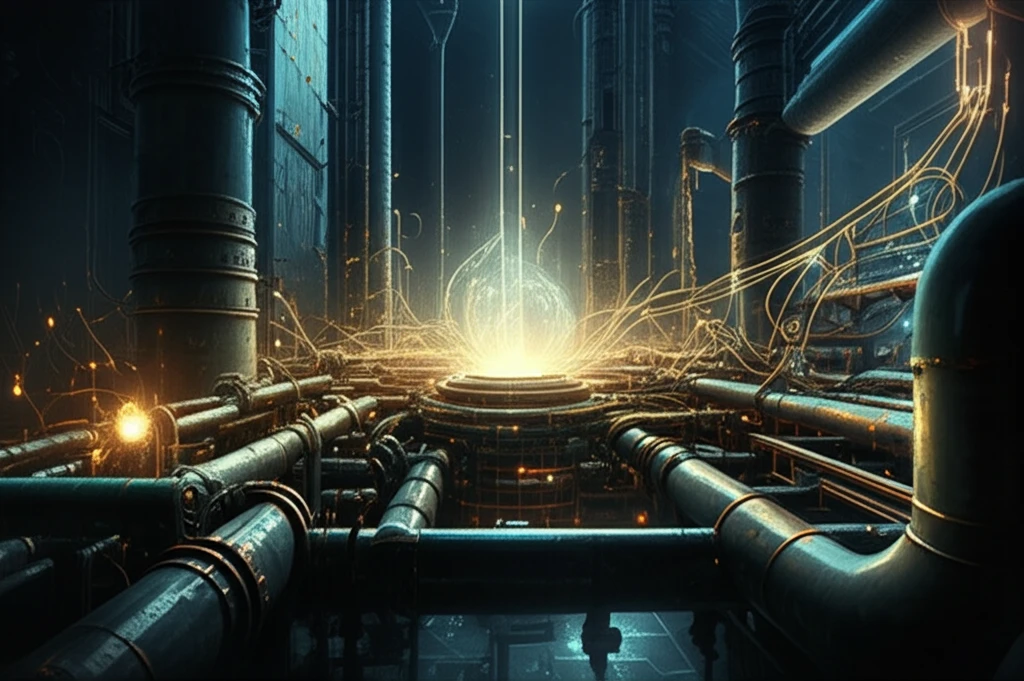
Water distribution networks can be modeled as a graph, with vertices representing pipe connections and edges representing the pipes themselves. Each vertex is associated with pressure, demand, and geodesic level, while each edge is characterized by pressure drop due to hydraulic resistance. This model allows us to analyze the network's behavior and develop control strategies to optimize pressure levels.
- Reduced Leaks: By maintaining optimal pressure, the likelihood of leaks due to excessive pressure is significantly reduced.
- Energy Savings: Lowering the amount of energy used by pumps.
- Improved Comfort: Stable pressure for all consumers.
- Avoiding increased risks of pollutants.
The Future of Smart Water Networks
The ILC-type control structure offers a promising approach to pressure control in water distribution networks, enabling reduced leaks, energy savings, and improved system performance. By leveraging AI and machine learning, we can create smarter and more resilient water systems that are better equipped to meet the challenges of water scarcity and environmental sustainability. Future research should focus on estimating inlet node elevation, handling sensor dropouts, and extending the approach to networks with elevated storage to push the boundaries of innovation in smart water management.