Smart Sensors, Smarter Living: How AI-Powered Sleep Strategies are Revolutionizing Healthcare
"Discover how Wireless Body Area Networks (WBANs) and data compression are paving the way for energy-efficient and intelligent healthcare solutions."
Imagine a world where healthcare is proactive, personalized, and seamlessly integrated into your daily life. Wireless Body Area Networks (WBANs) are making this vision a reality, utilizing small, wearable sensors to monitor vital physiological data. However, the challenge lies in powering these devices efficiently and ensuring long-lasting performance.
WBAN nodes typically rely on batteries, which are often limited in energy capacity and difficult to replace frequently. To address this issue, researchers have developed innovative data compression and energy-saving methods. One such approach involves using Sparse Representation Classification (SRC) algorithms to identify normal signals and Compressed Sensing (CS) theory for signal compression.
This groundbreaking method allows WBAN nodes to minimize energy consumption by entering a sleep state when normal signals are detected, reducing the need for continuous data transmission. By focusing on transmitting only essential data, this approach significantly extends the lifespan of WBAN devices, paving the way for more sustainable and efficient healthcare monitoring.
How Does Data Compression Optimize WBAN Energy Efficiency?
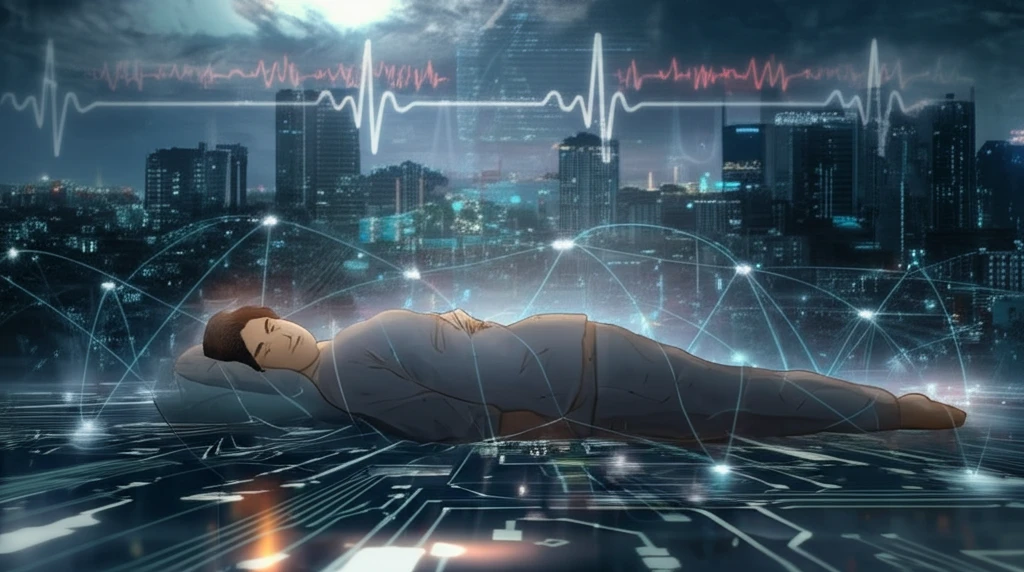
The core of this energy-saving method lies in leveraging Sparse Representation Classification (SRC) and Compressed Sensing (CS) techniques. SRC algorithms are employed to differentiate between normal and abnormal physiological signals. When the system identifies normal signals, the WBAN node enters a low-power sleep state, conserving energy.
- SRC Algorithm: Identifies normal signals, triggering the sleep state.
- CS Theory: Compresses signals to reduce data transmission volume.
- Signal Reconstruction: Ensures accurate data analysis at the base station.
The Future of WBAN Technology: Personalized and Energy-Efficient Healthcare
The integration of SRC and CS techniques in WBAN technology marks a significant step forward in creating more sustainable and efficient healthcare solutions. By enabling nodes to intelligently manage their energy consumption, these advancements pave the way for long-lasting, wearable health monitoring devices. This means more continuous and reliable data collection, leading to better patient care and proactive health management. As technology evolves, the potential for AI-driven, energy-efficient healthcare solutions will only continue to grow, transforming how we approach personal health and well-being.