Smart Sensors, Safer Systems: How AI is Revolutionizing Fault Detection
"Discover how data-driven fault detection methods are enhancing safety and reliability in everything from aerospace to everyday electronics using AI, reducing errors and improving maintenance."
Imagine a world where your devices not only tell you when something is wrong but also predict potential problems before they even happen. This isn't science fiction; it's the reality that advanced fault detection and isolation (FDI) technologies are bringing to various industries. Since the concept of autonomous fault diagnosis emerged, interest in this field has exploded, with new methods promising to make our systems safer and more reliable.
Traditional fault diagnosis falls into two main categories: model-based and data-driven approaches. Model-based methods rely on detailed mathematical descriptions of systems, which can be challenging to develop and maintain for complex engineering systems. Advances in sensing and data acquisition now provide huge volumes of raw data, making data-driven methods more attractive. This shift allows engineers to harness the power of artificial intelligence and machine learning to identify and address potential faults.
Data-driven techniques use a variety of tools, including neural networks and fuzzy logic, to extend and improve traditional model-based FDI schemes. One straightforward solution involves creating a dynamical mathematical model from available data and using this model to design conventional FDI systems. However, this approach can suffer from errors introduced during system identification, ultimately undermining the reliability of the fault diagnosis scheme.
The Rise of Data-Driven Fault Detection
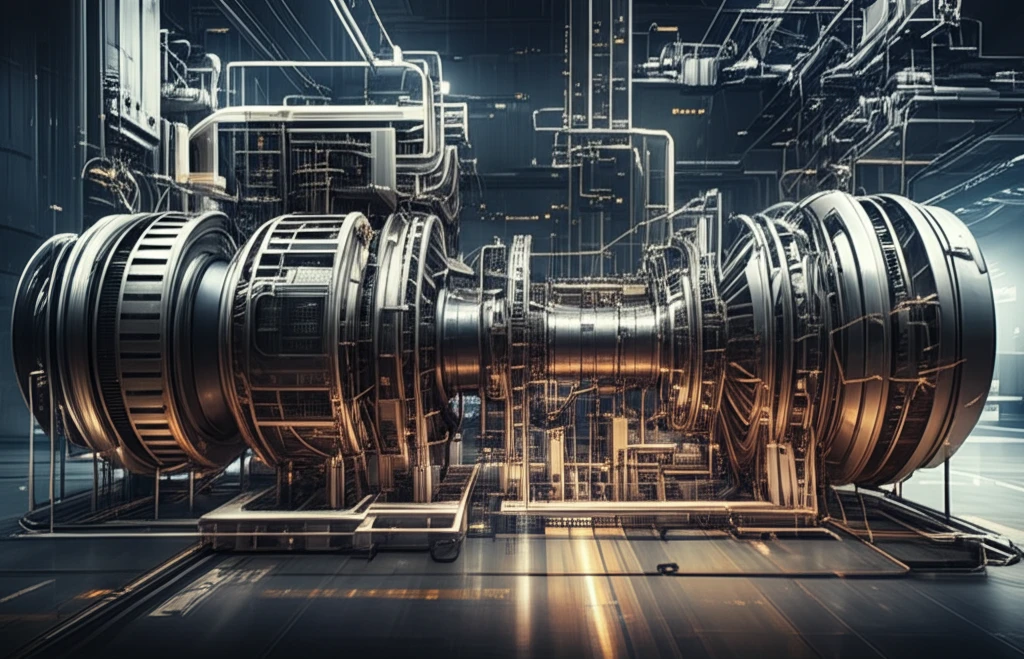
In recent years, a new approach has emerged, focusing on directly constructing FDI schemes from system input-output (I/O) data. These methods, known as subspace-based data-driven fault detection and isolation, identify the system's left null space using I/O data. This process typically involves a reduction step, where the system order is estimated via Singular Value Decomposition (SVD). However, this step can be problematic because choosing a truncation point for 'small' singular values is subjective and can lead to errors.
- Adaptability: AI algorithms can adapt to changes in the system over time, ensuring that the fault detection system remains effective even as the system evolves.
- Comprehensive Analysis: AI algorithms can analyze vast amounts of data to identify patterns and anomalies that might be missed by traditional methods.
- Predictive Maintenance: AI can help predict when maintenance will be needed, reducing downtime and improving efficiency.
The Future of Fault Detection
As technology advances, the ability to quickly and accurately detect and isolate faults will become even more critical. Data-driven fault detection methodologies, particularly those enhanced by AI, offer a promising path toward creating safer, more reliable systems across industries. By overcoming the limitations of traditional model-based approaches, these innovative techniques are paving the way for a future where potential failures are identified and addressed before they can cause significant disruptions.