Smart Savings: How AI is Revolutionizing Home Energy Forecasting
"Discover how cutting-edge hybrid deep learning strategies are making multi-step short-term power consumption forecasting more accurate and accessible, paving the way for smarter energy use and significant savings."
In an era where smart homes and sustainable living are becoming increasingly important, accurately forecasting household power consumption is a game-changer. Artificial intelligence (AI) is at the forefront of this revolution, offering tools to predict energy usage with unprecedented precision. This technology not only promises to cut down on energy waste but also plays a crucial role in the planning of smart grids, promoting sustainable energy practices, and designing more efficient electricity market bidding systems.
Traditional methods of forecasting, such as basic statistical models and conventional machine learning techniques, often fall short when faced with the personalized and variable energy consumption patterns of individual households. This is where advanced AI, particularly deep learning neural networks, steps in to bridge the gap, offering more reliable and accurate predictions.
Recent research highlights the potential of Long Short-Term Memory (LSTM) neural networks, a type of recurrent neural network, in outperforming traditional methods for power consumption forecasting. However, challenges remain, particularly in achieving the level of accuracy required for real-world applications and in forecasting energy use over extended periods.
The Power of Hybrid Deep Learning: CNNs and LSTMs
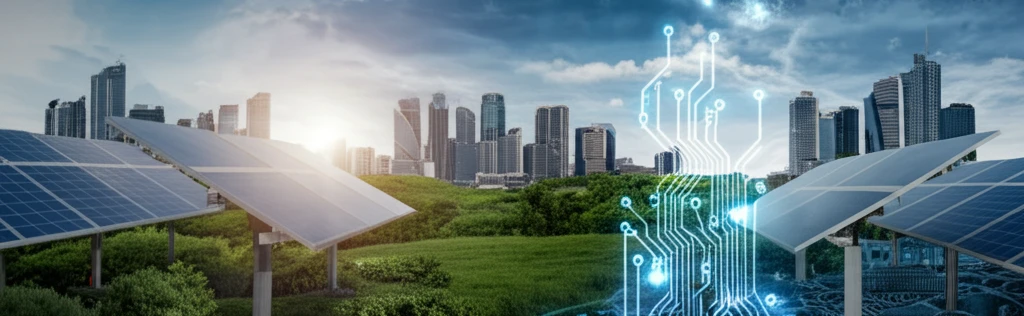
To tackle these challenges, a novel approach combines Convolutional Neural Networks (CNNs) with LSTM networks. This hybrid deep-learning strategy leverages the strengths of both architectures to enhance the accuracy of power consumption forecasts. CNNs are excellent at extracting complex features from data, while LSTMs excel at learning from sequences, making them ideal for time-series forecasting.
- Improved Accuracy: Hybrid models outperform traditional methods.
- Extended Forecasting: Multi-step forecasting offers longer prediction windows.
- Real-World Application: Enhances energy market bidding and smart grid management.
- Data Flexibility: Effective with single-source power consumption data.
The Future of Energy Forecasting
As AI technology continues to evolve, the potential for even more sophisticated energy forecasting models is vast. The CNN-LSTM framework represents a significant step forward, offering a pathway to more sustainable energy consumption and savings. By providing accurate, multi-step forecasts, this technology not only helps individual households manage their energy use but also contributes to the development of smarter, more resilient energy grids. The intersection of AI and energy management holds the key to a future where energy is used more efficiently, costs are reduced, and environmental impact is minimized.