Small Steps, Big Impact: How Prioritizing Safety Can Revolutionize Medical Trials
"Discover how new statistical approaches are making medical experiments safer and more effective, especially when starting with limited data."
Imagine you’ve developed a promising new medical intervention. Before rolling it out widely, you need to know if it truly works and, crucially, if it’s safe. So, you begin with a small experiment, perhaps just two participants. Your primary concern? Safety. It’s far worse to administer an unsafe treatment than to withhold one that could be beneficial. The experiment concludes, and both participants—one receiving the intervention, the other a placebo—are alive and well. Now what? Should you administer the treatment to more people, or should you scrap the idea?
Questions like these are at the heart of causal inference, a field that grapples with determining cause-and-effect relationships. Traditionally, researchers might rely on intuition or simple statistical tests. However, a groundbreaking study is proposing a more rigorous, data-driven approach, especially tailored for those crucial early stages of medical trials where every data point matters.
This article delves into how a novel approach combines the exact finite sample likelihood—a precise way of calculating probabilities in small samples—with statistical decision theory. This powerful combination provides a framework for making informed decisions that prioritize patient safety, even when data is scarce. Let's explore how this can revolutionize the way we approach medical experiments.
Why Prioritizing Safety is a Game-Changer in Medical Trials
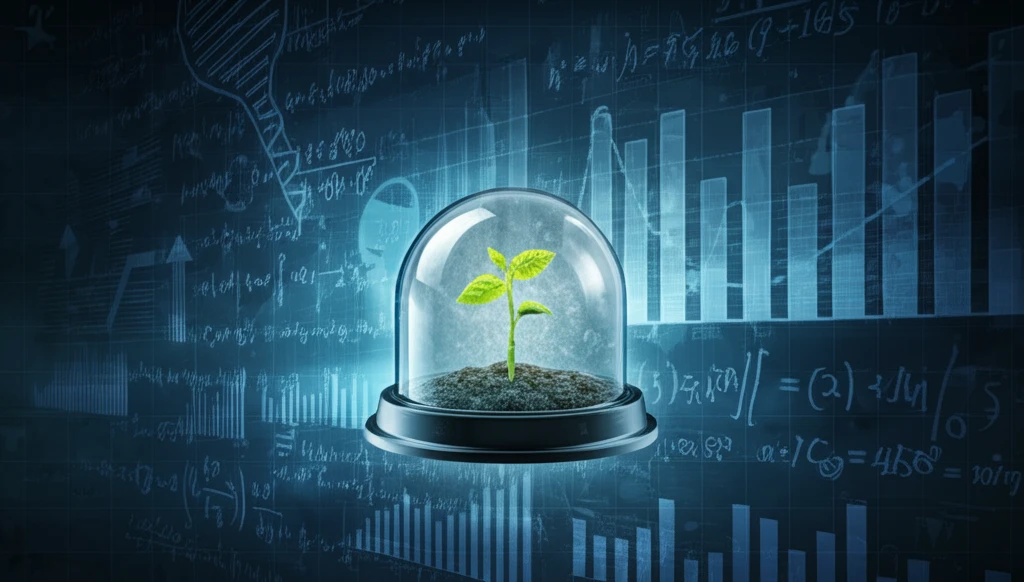
Traditional approaches to medical trials often focus on efficacy—how well a treatment works. However, in the initial phases, safety should be paramount. Administering a potentially harmful treatment can have devastating consequences, making a safety-first approach ethically and practically essential. This new statistical method acknowledges this asymmetry, incorporating it directly into the decision-making process.
- More Informed Decisions: The approach uses all available data to make informed decisions, even when the sample size is small.
- Prioritization of Safety: The utility function is designed to penalize unsafe interventions more heavily than missed opportunities for effective treatments.
- Improved Performance: The research demonstrates that, in many cases, these new decision rules outperform traditional methods, especially in adhering to maximin and maximum regret criteria.
Looking Ahead: The Future of Safer Medical Innovations
This innovative statistical approach represents a significant step forward in making early-stage medical trials safer and more effective. By prioritizing safety and using precise calculations tailored for small samples, researchers can make more informed decisions about whether to proceed with further testing. As the field evolves, we can expect even more sophisticated methods that further refine our ability to evaluate new medical interventions responsibly and ethically, ensuring that patient well-being remains at the forefront of medical innovation.