Simplify Your Time Series Data: How Memetic Algorithms are Revolutionizing Data Reduction
"Discover how a novel memetic coral reefs optimization algorithm (MCRO) is setting new standards in time series approximation and data reduction, making complex data more manageable and insightful."
In an era defined by unprecedented data volumes, the ability to efficiently manage and analyze temporal data has become a critical challenge across various sectors. The exponential growth of available time series data—from financial markets to climate patterns—demands innovative techniques that can distill valuable insights without being overwhelmed by the sheer size and complexity of the datasets. As a result, researchers and practitioners are continuously seeking automated methods to reduce the number of data points in time series, making analysis faster, more accurate, and more accessible.
Traditional approaches to time series analysis often struggle with the computational demands and storage requirements of large datasets. However, a new study introduces a promising solution: a novel modification of the coral reefs optimization algorithm (CRO), enhanced with memetic strategies to minimize approximation errors and optimize data reduction. This approach, known as memetic CRO (MCRO), represents a significant step forward in the field of data optimization, offering a more efficient way to handle the increasing flood of temporal data. Memetic algorithms, which combine evolutionary strategies with local search techniques, have proven to be exceptionally effective in solving complex optimization problems.
The MCRO algorithm refines solutions through local optimization and reintegration into the evolutionary process, leveraging two well-established algorithms—Bottom-Up and Top-Down—to enhance its performance. By comparing MCRO against standard CRO and its statistically driven and hybrid variants, the study demonstrates MCRO's superior ability to reduce time series size while preserving critical information. Tested across 15 diverse time series, MCRO consistently delivers the best results, marking a significant advancement in data reduction methodologies.
The Power of Memetic Algorithms in Time Series Analysis
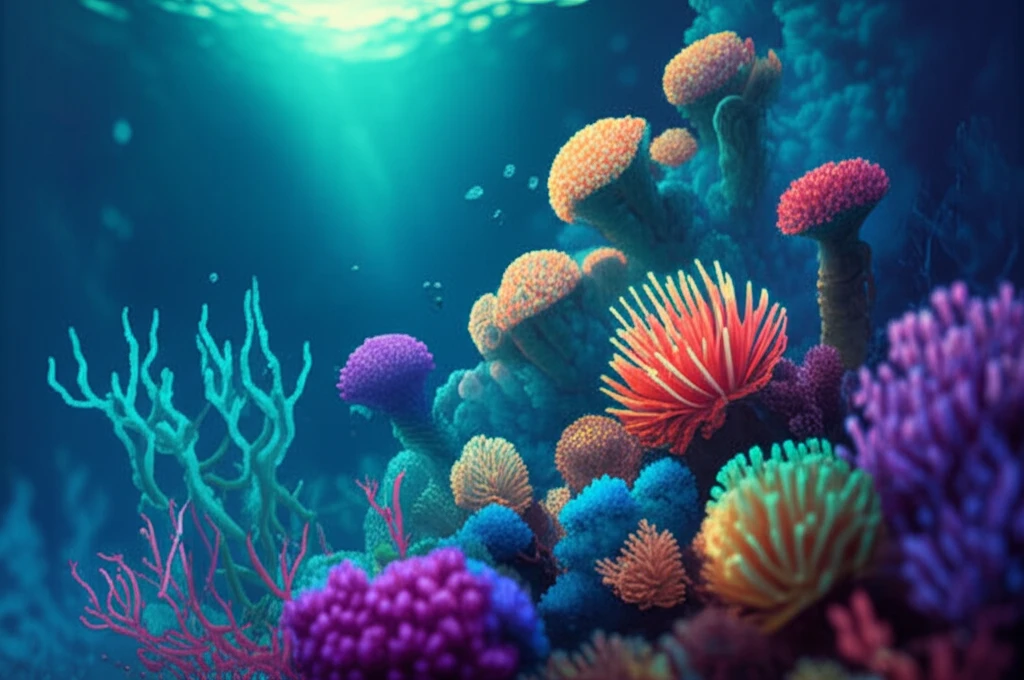
At its core, the MCRO algorithm addresses the challenge of time series size reduction by optimizing a trade-off between data volume and approximation accuracy. The primary goal is to minimize the error introduced when reducing the number of data points, ensuring that the simplified time series remains a faithful representation of the original data. This is particularly important in applications where subtle patterns and trends within the data carry significant meaning. The algorithm’s efficiency is rooted in its unique approach to balancing global exploration with local refinement, a hallmark of memetic algorithms.
- Hybridization: Combines global exploration with local exploitation for enhanced optimization.
- Local Optimization: Refines solutions using Bottom-Up and Top-Down algorithms.
- Reintegration: Reintroduces optimized solutions into the population to guide further evolution.
- Adaptability: Proven effective across diverse time series data.
The Future of Time Series Data Management
The development of the MCRO algorithm represents a significant step forward in time series data management. As data continues to grow in volume and complexity, the ability to efficiently reduce and analyze time series data will become increasingly critical. The MCRO algorithm offers a powerful tool for researchers and practitioners seeking to extract valuable insights from temporal data while minimizing computational costs. Future research will likely focus on adapting the MCRO algorithm to other tasks, such as numerical or real functions minimization, further expanding its applicability and impact.