Real-Time Reliability: How Trans-Layer Model Learning is Revolutionizing Complex Systems
"Discover how a hierarchical modeling strategy enhances the safety and efficiency of everything from aircraft engines to industrial plants, ensuring systems operate at their peak."
In today's fast-paced world, ensuring the reliability of complex systems is more critical than ever. From aircraft engines to large-scale industrial plants, these systems are the backbone of our modern infrastructure. Proactive maintenance, a strategy championed by the Prognostics and Health Management (PHM) society, is key to improving system availability, enhancing safety, and keeping maintenance costs in check. At the heart of proactive maintenance lies the ability to accurately estimate the Remaining Useful Life (RUL) of system components using real-time data.
Estimating RUL involves a significant challenge: understanding the loading conditions and performance of components within complex systems. These systems often exhibit multiple, simultaneous degradation processes that are hidden, time-varying, and nonlinear. While numerous methods have been proposed to address this issue, including state space models, Kalman filters, and Markovian models, they often fall short in providing direct insights into the hidden degradation details of components.
This is where Trans-Layer Model Learning (TLML) comes into play. TLML is a hierarchical modeling strategy designed to bridge the gap between system-level observations and component-level behaviors. TLML offers a promising solution for enhancing the real-time RUL estimation of system parts. This strategy involves building system models that mirror the hierarchical topologies of real-world implementations, allowing for a more accurate and comprehensive understanding of system dynamics.
What is Trans-Layer Model Learning (TLML)?
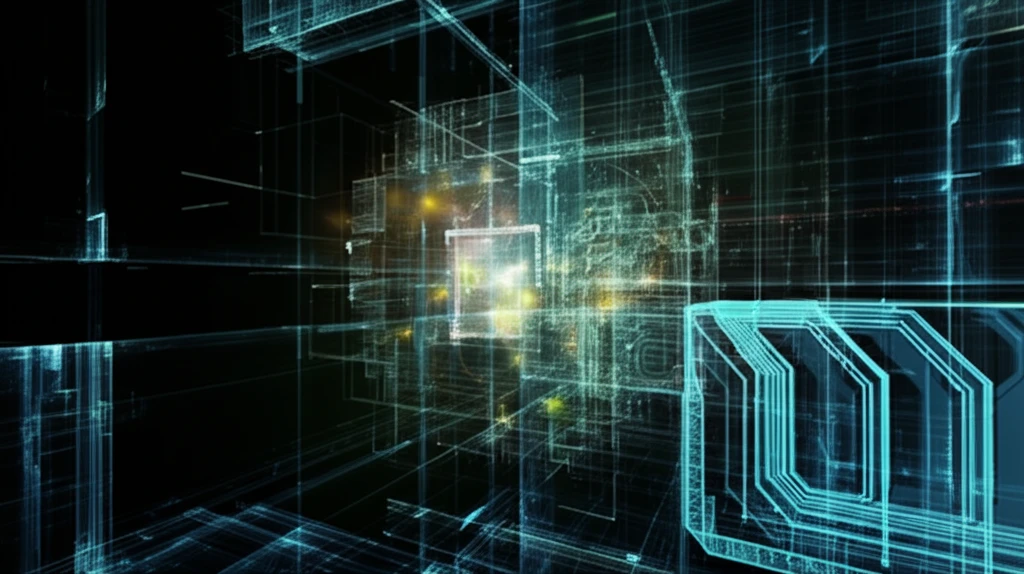
Trans-Layer Model Learning (TLML) is an innovative approach to system modeling that focuses on integrating empirical rules with real-time condition monitoring data. The primary goal of TLML is to enhance the observability of system components, particularly in complex systems where direct observation is limited. By combining data-driven techniques with established knowledge, TLML provides a more accurate and reliable assessment of system health.
- Enhanced Observability: TLML improves the ability to understand the internal states of system components.
- Data Integration: Combines real-time sensor data with empirical rules.
- Improved Accuracy: Offers more precise predictions of component behavior and RUL.
- Hierarchical Modeling: Builds models that mirror real-world system topologies.
The Future of Reliability: Why TLML Matters
Trans-Layer Model Learning (TLML) represents a significant step forward in the field of system reliability. By integrating real-time data with empirical knowledge, TLML offers a more comprehensive and accurate approach to predictive maintenance. As industries continue to rely on increasingly complex systems, the ability to ensure their reliability and safety will become even more critical. TLML provides a powerful tool for achieving this goal, paving the way for safer, more efficient, and more sustainable operations.