P-Hacking: Are Your Research Results Just a Statistical Illusion?
"Uncover the truth behind p-hacking, its impact on research integrity, and how to detect it in the age of information overload."
In an era defined by data, the integrity of research findings is paramount. Yet, a subtle menace known as 'p-hacking' threatens to undermine the very foundations of empirical studies. Imagine making critical decisions based on research that, unbeknownst to you, has been manipulated to achieve statistically significant results. This isn't just a hypothetical scenario; it’s a growing concern across numerous disciplines.
P-hacking, in essence, involves tweaking research methods or data analysis until a desired p-value—typically p < 0.05, the conventional threshold for statistical significance—is achieved. While not always intentional, this practice can lead to false positives and skewed results, eroding the reliability of published research. Recognizing and combating p-hacking is crucial for anyone who relies on data-driven insights, from policymakers to business leaders and academics.
This article explores the pervasive issue of p-hacking, dissecting its various forms and revealing its potential impact on research validity. We’ll delve into practical methods for detecting p-hacking, empowering you to critically assess the research you encounter and ensuring that your decisions are grounded in trustworthy evidence.
What is P-Hacking and Why Should You Care?
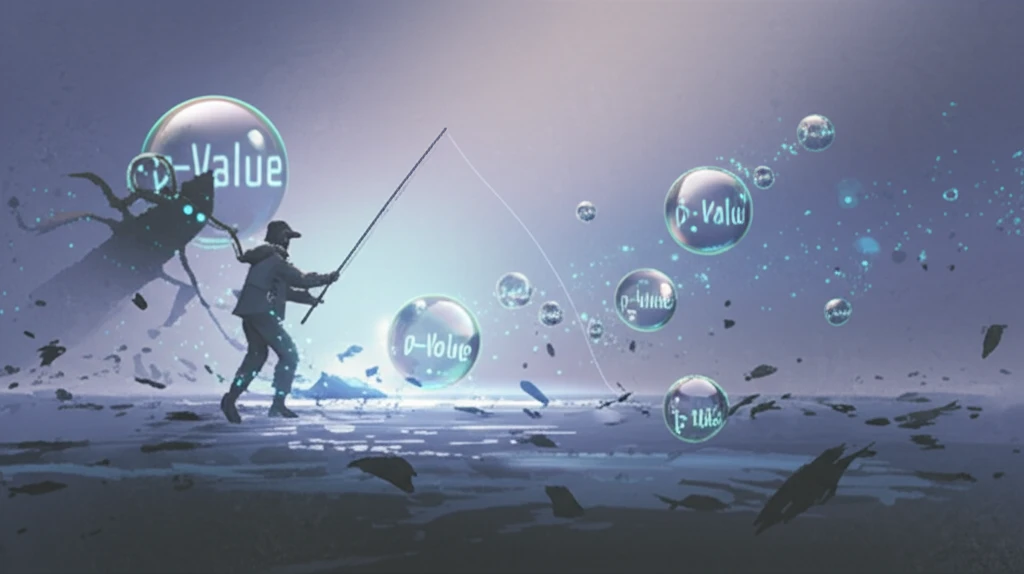
P-hacking, also known as data dredging, data fishing, or selective reporting, is a practice where researchers consciously or unconsciously manipulate their data analysis to achieve statistically significant results. This often involves:
- Selecting favorable data subsets: Focusing on specific segments of data that support the hypothesis while ignoring contradictory data.
- Adding or removing variables: Including or excluding variables in a regression model until a significant p-value is obtained.
- Altering statistical tests: Switching between different statistical tests to find one that yields the desired outcome.
- Stopping data collection prematurely: Ending the experiment once the results align with the hypothesis, rather than continuing until a predetermined sample size is reached.
The Future of Research Integrity: Staying Vigilant Against P-Hacking
P-hacking poses a significant threat to the reliability of research across many fields. By understanding the different forms of p-hacking and applying the methods described in this article, you can critically evaluate research findings and promote greater integrity in the scientific process. Staying informed and proactive is essential for safeguarding the trustworthiness of research and ensuring that evidence-based decisions are based on solid foundations.