Mastering the Market: How Reinforcement Learning is Revolutionizing Financial Index Tracking
"Discover how advanced AI strategies, including deep reinforcement learning, are enhancing index tracking and potentially unlocking extra profits."
In today's fast-paced financial world, the ability to accurately track market indices while minimizing costs is crucial. Traditionally, financial index tracking—replicating the performance of a specific market index like the S&P 500—has relied on static models that often fall short in capturing market dynamics. However, a new approach is emerging that promises to revolutionize this field: reinforcement learning (RL).
Reinforcement learning, a type of artificial intelligence, allows financial models to learn from experience, adapting dynamically to changing market conditions. Unlike static models, RL can incorporate a multitude of market variables, accurately calculate transaction costs, and even make strategic decisions about when to inject or withdraw cash from a tracking portfolio. This leads to more precise tracking and opportunities for additional profit.
This article explores how reinforcement learning is being applied to financial index tracking, offering a breakthrough solution that addresses the limitations of existing models. We’ll delve into the specifics of how RL works in this context, its advantages, and the potential it holds for the future of financial management. Whether you're a seasoned investor or simply curious about the intersection of AI and finance, this is an area worth watching.
Why Traditional Index Tracking Models Fall Short
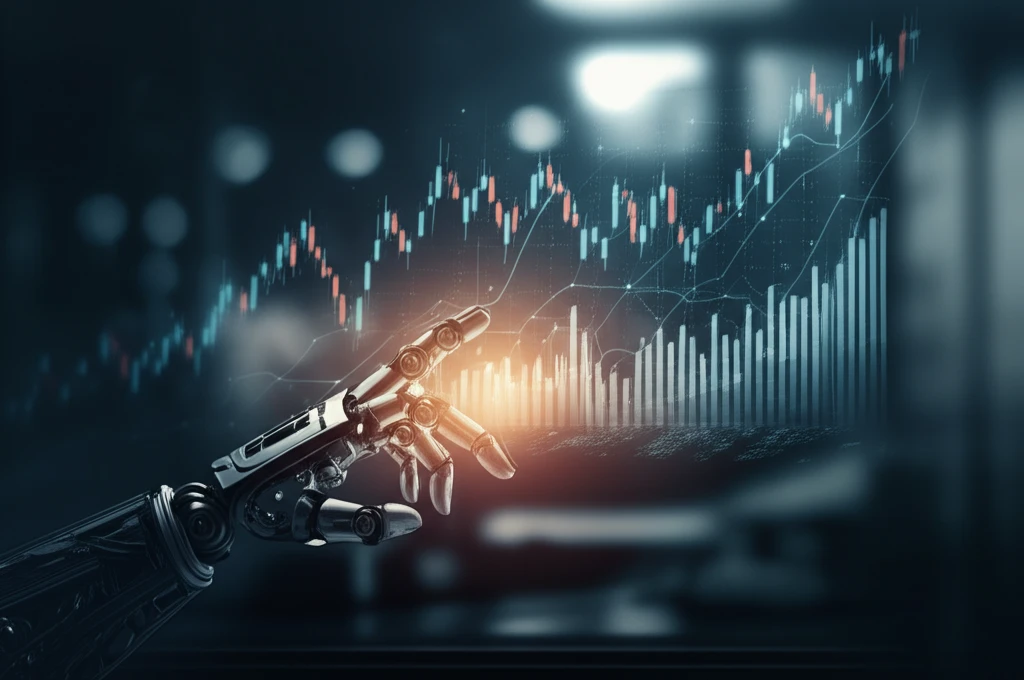
Before diving into the specifics of reinforcement learning, it’s essential to understand the challenges faced by traditional index tracking methods. Static models, which have dominated the field, operate under several assumptions that can limit their effectiveness:
- Limited Data Use: Static models typically use only short historical data periods, which can lead to a waste of valuable information.
- Lack of Responsiveness: Portfolio weights are fixed based on past data and are not responsive to current market changes.
- Inaccurate Cost Calculation: Most models either ignore transaction costs or only account for them at the beginning of a period, failing to capture the ongoing impact.
- Inability to Balance Trade-offs: Static models struggle to balance the trade-off between tracking error and transaction costs over extended periods.
- Ignoring Market Information: These models often fail to incorporate diverse market information beyond prices and returns, such as trading volume or market sentiment.
The Future of Financial Index Tracking
Reinforcement learning is poised to play a transformative role in financial index tracking. By overcoming the limitations of traditional static models, RL offers a dynamic, data-driven approach that can enhance portfolio management, reduce tracking errors, and potentially unlock extra profits. As AI technology continues to evolve, we can expect even more sophisticated applications of RL in finance, shaping the future of investment strategies.