Leaf Identification: How AI is Revolutionizing Plant Species Classification
"Discover how Integral Contour Angle (ICA), an innovative shape descriptor, is enhancing the accuracy of leaf image classification and retrieval in computer vision."
Leaf identification has long been a significant yet challenging task in computer vision. The difficulty arises from the vast variations within the same plant species and the subtle differences between different species. Traditional methods often struggle to accurately classify and retrieve leaf images due to these complexities.
However, recent advancements in artificial intelligence are offering new solutions. One promising approach is the use of novel shape descriptors that can effectively capture the unique characteristics of leaf shapes. Among these, the Integral Contour Angle (ICA) stands out as a particularly effective tool.
This article delves into how the ICA descriptor works, its advantages over traditional methods, and its potential impact on various applications, from botany to environmental conservation. Discover how AI is revolutionizing the way we identify and classify plant species.
The Integral Contour Angle (ICA): A Novel Approach
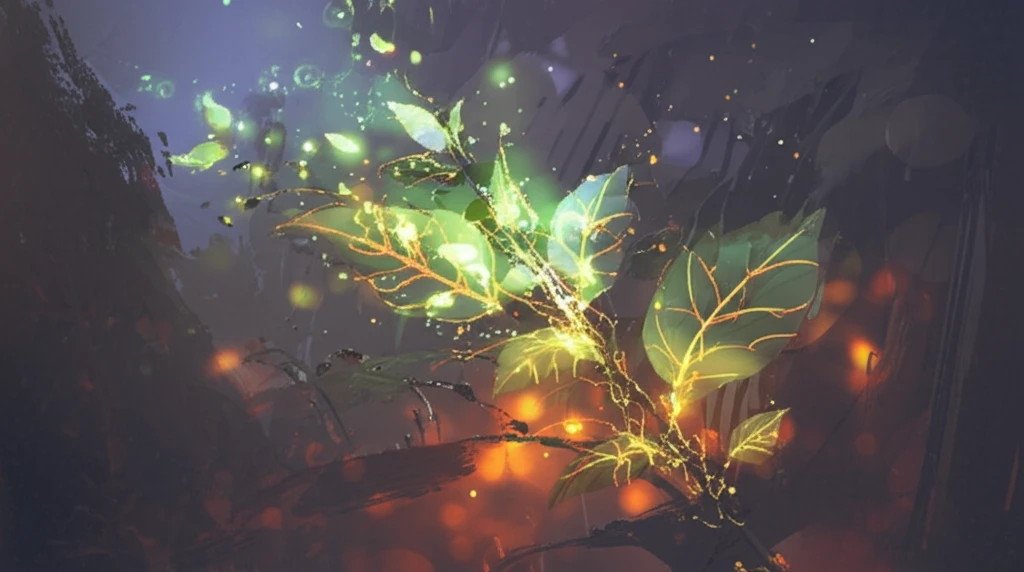
The Integral Contour Angle (ICA) is a shape descriptor designed for accurate leaf image classification and retrieval. Unlike traditional methods that rely on direct curvature calculations (often sensitive to noise), ICA uses a more robust approach. For any given point on a leaf's outline, ICA considers two sets of vectors extending to neighboring points on either side. The average direction of these vectors forms an angle – the Integral Contour Angle.
- Translation Invariance: ICA remains consistent regardless of the leaf's position in the image.
- Rotation Invariance: ICA is unaffected by the leaf's orientation.
- Scale Invariance: ICA works equally well for leaves of different sizes.
- Multi-Scale Analysis: Captures both coarse and fine details of the leaf shape.
The Future of Leaf Identification
The development of ICA represents a significant step forward in leaf identification and shape description. Its inherent invariance to transformations, robustness to noise, and ability to capture multi-scale features make it a powerful tool for accurate classification and retrieval. As AI technology continues to advance, methods like ICA will play an increasingly important role in various fields, from botany and agriculture to environmental conservation and biodiversity research.