Keep Your Machines Running Smoothly: A Guide to Predictive Maintenance
"Leveraging Advanced Analytics for Enhanced Bearing Performance and Equipment Reliability"
In today's fast-paced industrial landscape, keeping machinery running smoothly is more critical than ever. Unexpected breakdowns can lead to significant downtime, impacting productivity and profitability. That's why companies are increasingly turning to condition-based maintenance (CBM), a strategy that relies on real-time monitoring to predict and prevent equipment failures.
Rolling bearings, essential components in rotating machinery, are particularly prone to faults. In fact, bearing-related issues account for a substantial percentage of machinery breakdowns. By identifying potential problems early, businesses can schedule maintenance proactively, minimizing disruptions and maximizing the lifespan of their equipment.
Recent research introduces a novel approach to bearing condition assessment, combining basic scale entropy (BSE) and Gath-Geva fuzzy clustering. This method promises to enhance the accuracy and efficiency of CBM, offering a powerful tool for predictive maintenance strategies.
What is Basic Scale Entropy (BSE) and How Does It Work?
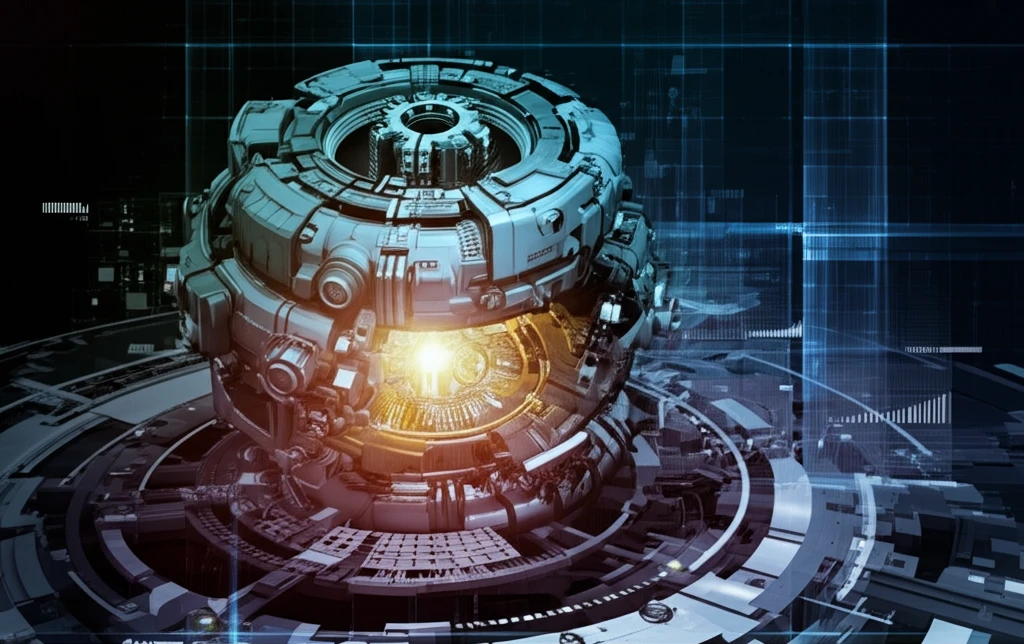
Basic Scale Entropy (BSE) is a sophisticated technique used to analyze the complexity of signals, like those generated by a machine’s vibrations. Imagine listening to the sound your car makes – a smooth, consistent hum is easy to predict, but a series of clanks and rattles indicates something is amiss. BSE works similarly, but on a much more granular level, quantifying the unpredictability in the vibration patterns of a bearing.
- Dimension Transforming: Converts a one-dimensional signal (like a time-series of vibration data) into a multi-dimensional vector. This essentially reorganizes the data to capture its behavior over time.
- Symbol Converting: Transforms the multi-dimensional vectors into a sequence of symbols based on a 'basic scale parameter'. This parameter determines how sensitive the analysis is to variations in the signal.
- Probability Statistics and Information Calculation: Analyzes the frequency of different symbol sequences and calculates the BSE value, which reflects the complexity of the signal. A higher BSE value indicates a more complex, and potentially problematic, vibration pattern.
The Future of Machine Maintenance is Here
By embracing these advanced techniques, businesses can move beyond reactive maintenance and into a new era of predictive, data-driven decision-making. The result? Reduced downtime, extended equipment lifespans, and a more efficient, profitable operation.