Is Your Investment Strategy Future-Proof? How AI is Changing the Game
"Discover how Generative Adversarial Networks (GANs) are revolutionizing robust utility optimization, offering a versatile approach to navigate market uncertainties and trading costs."
In today's volatile financial landscape, uncertainty is the only constant. For risk managers and traders, effectively modeling and managing this uncertainty is paramount to making informed financial decisions. Robust utility optimization stands out as a crucial tool for rational decision-making, enabling a delicate balance between maximizing returns and managing potential risks while aligning with individual preferences and long-term financial goals.
But what happens when you're unsure about the behavior of the market itself? That's where robust utility optimization truly shines. It allows investors to prepare for a range of possible market scenarios, ensuring their portfolios can weather various economic storms. The goal is to build resilient strategies that perform well, even in the face of adverse market conditions.
Now, a groundbreaking approach is emerging, promising to reshape how we tackle robust utility optimization: Generative Adversarial Networks, or GANs. These AI-powered tools are demonstrating remarkable potential in solving complex investment problems, offering new avenues for navigating uncertainty and optimizing portfolios in realistic market settings. Get ready to explore how GANs are poised to revolutionize the world of finance.
GANs: The AI Game-Changers for Investment
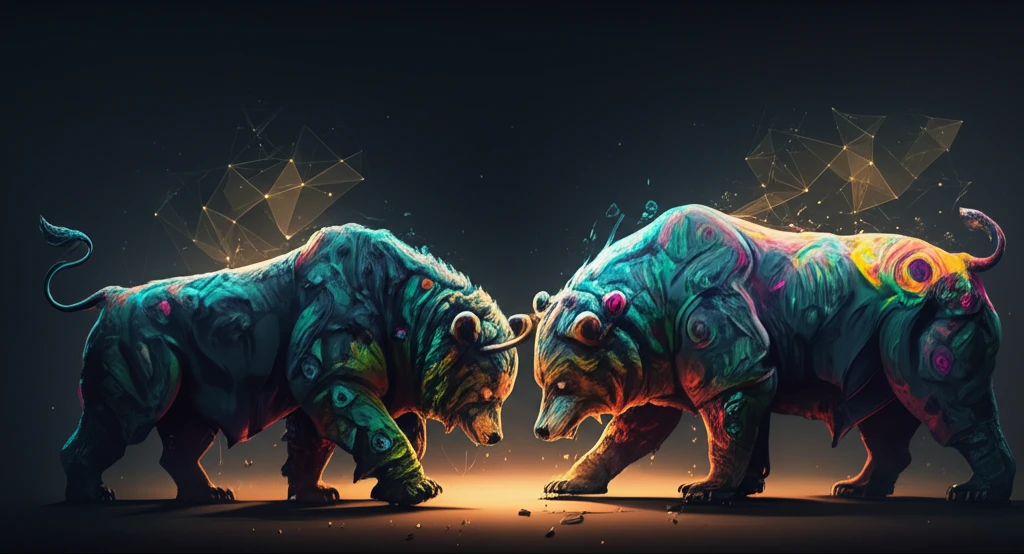
Imagine a constant tug-of-war, but instead of a rope, it's data, and instead of people, it's two competing AI systems. That's essentially how GANs work. GANs consist of two neural networks: a generator and a discriminator. These networks are trained together in a game-like setup, constantly challenging each other to improve. In a typical application, the generator creates new data, while the discriminator tries to distinguish between the generated data and real, existing data.
- Image and Text Generation: GANs can create realistic images from scratch or generate text with specific styles.
- Video Synthesis: They can be used to create new video content or modify existing videos.
- Drug Discovery: GANs can even aid in the discovery of new drug candidates by generating novel molecular structures.
The Future of Investing is Intelligent
The rise of GANs in robust utility optimization signifies a major leap forward in financial modeling and investment strategy. By providing a versatile and data-driven approach to navigating market uncertainties and trading costs, GANs are empowering investors to make more informed and resilient decisions. As AI continues to evolve, expect even more sophisticated applications to emerge, further transforming the financial landscape.