Is Your Data Telling the Truth? How to Know If Your Statistical Tests Are Accurate
"A Deep Dive into Exponentiality Testing Using Renyi Distance: Ensuring Reliability in Your Data Analysis"
In our increasingly data-driven world, making informed decisions hinges on the reliability of the statistical tests we employ. Imagine making critical business decisions, developing healthcare strategies, or formulating public policies based on flawed data analysis. The consequences can be severe, leading to wasted resources, ineffective interventions, and even harmful outcomes. That's why it's crucial to ensure the statistical methods we use are accurate and trustworthy.
One common task in data analysis is assessing whether a dataset follows a specific probability distribution. The exponential distribution, in particular, pops up everywhere – from predicting the lifespan of electronic devices to modeling customer waiting times and understanding financial risk. But how can you be sure your data truly fits an exponential pattern? The answer lies in the power of goodness-of-fit tests, which act like quality control checks for your data.
This article explores a fascinating approach to goodness-of-fit testing for the exponential distribution, leveraging something called 'Renyi distance'. We'll break down the key concepts, explain how this method works, and discuss its advantages in ensuring the reliability of your statistical analyses. Whether you're a data scientist, a business analyst, or just someone curious about the world of statistics, this guide will provide valuable insights into the importance of accurate data validation.
The Renyi Distance Test: A New Way to Check Your Data
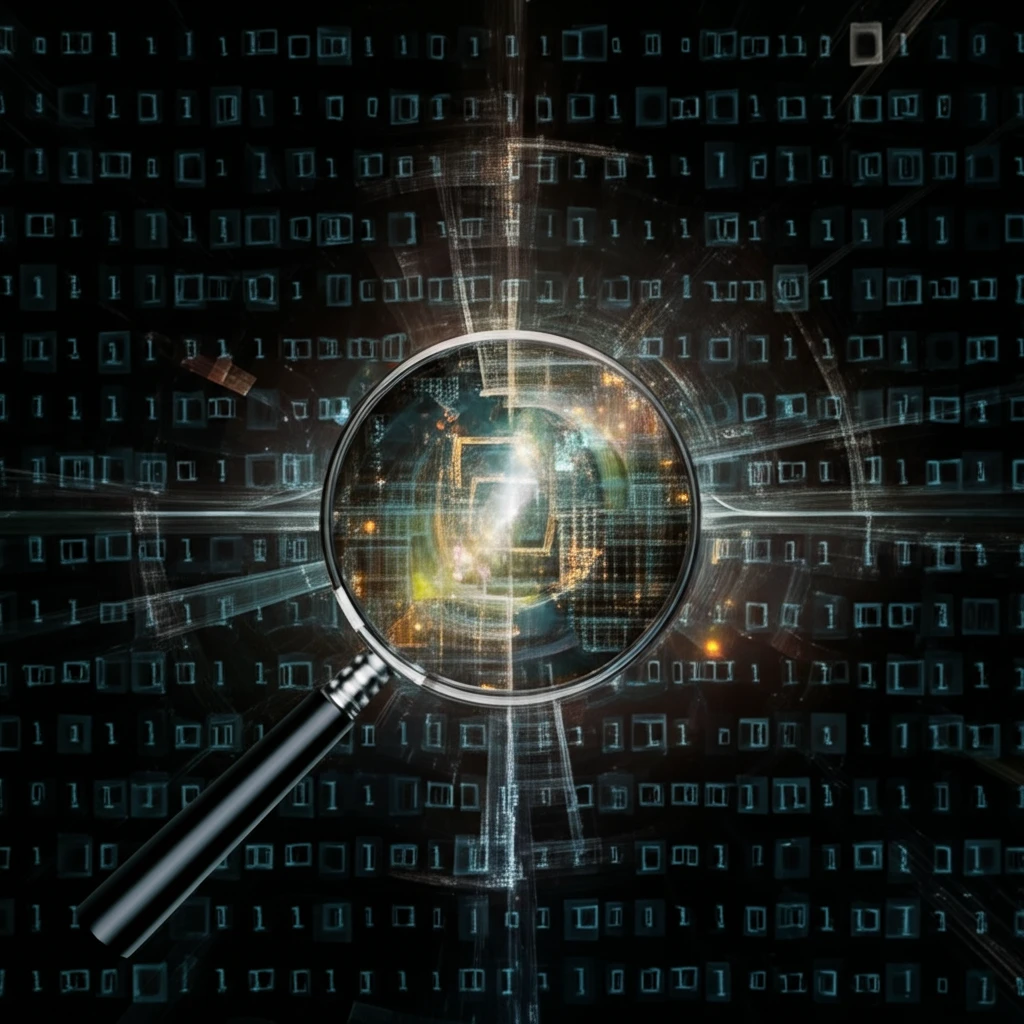
At its heart, this method is all about measuring the 'distance' between two probability distributions. In our case, we're interested in the distance between the empirical distribution of your data (what your data actually looks like) and the theoretical exponential distribution (what it should look like if it truly is exponential). The smaller the distance, the better the fit.
- Power: The ability of the test to correctly reject the null hypothesis when it is false.
- Sensitivity: How well the test performs under different conditions.
- Computational Complexity: The resources required to perform the test.
The Bottom Line: Why Accurate Data Validation Matters
In conclusion, the Renyi distance test provides a valuable tool for assessing whether your data truly follows an exponential distribution. By offering improved power compared to other methods, particularly when dealing with decreasing hazard rates, this test can help you make more confident data-driven decisions. As data continues to grow in importance across all fields, embracing robust and accurate validation techniques like the Renyi distance test will become increasingly essential for ensuring the reliability of our insights and actions.