Is Your Data Misleading You? The Hidden Dangers of Measurement Error in Network Analysis
"Uncover how seemingly small data inaccuracies can drastically distort your understanding of social trends and economic forecasts."
In today's data-driven world, network analysis has become a cornerstone of understanding complex systems. From tracking disease outbreaks to predicting technology adoption and analyzing social influence, diffusion models based on network data provide invaluable insights. However, what happens when the data we rely on isn't as accurate as we think?
A groundbreaking study highlights a critical issue: even minor inaccuracies in network data can lead to major distortions in the results of diffusion models. These seemingly small measurement errors can significantly alter predictions, causing policymakers and businesses to make flawed decisions. This article will unpack the complexities of this problem and show how to navigate the hidden dangers of data mismeasurement.
Imagine relying on a weather forecast that's slightly off – it could mean packing the wrong clothes for a trip. Now, amplify that risk to the scale of a public health crisis or a multi-million dollar marketing campaign. The stakes are high, and understanding the limitations of our data is more important than ever.
Why Small Errors Matter: The Butterfly Effect in Network Data
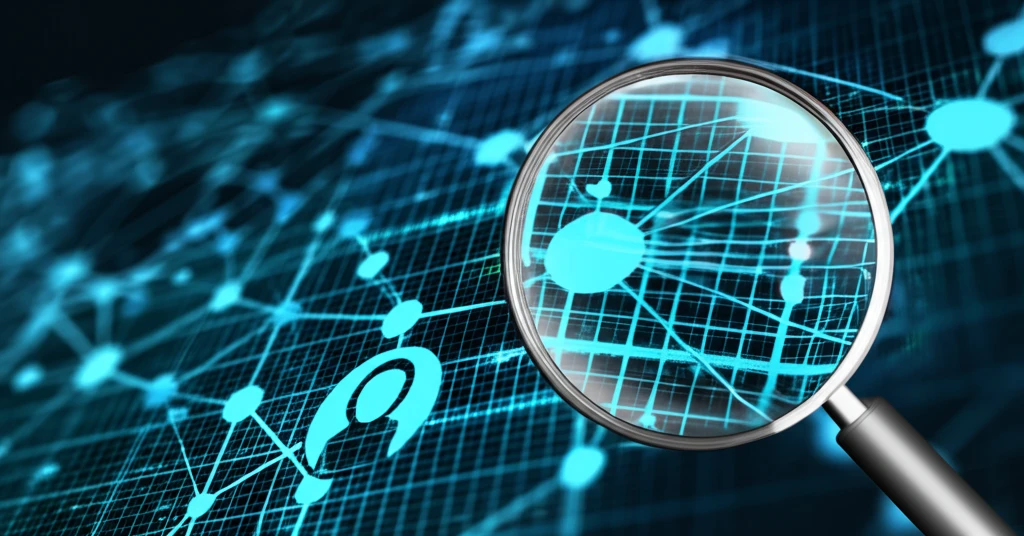
The core issue lies in the non-robustness of diffusion estimates. Diffusion models are designed to simulate how information, behaviors, or diseases spread through a network. These models rely on accurately mapping the connections between individuals or entities within the network. However, when those connections are misreported or missing, the model's predictions can go awry.
- Inaccurate Seed Data: Even if the network structure is perfectly mapped, slight errors in identifying the initial source of a diffusion (e.g., the first person infected in a disease outbreak) can drastically change the predicted spread. It's like a ripple effect – a small change at the beginning leads to large deviations later on.
- Missing Connections: If the initial source is known, but some connections within the network are missed, the diffusion process can be significantly underestimated. These missed links act as unseen pathways, allowing the diffusion to spread further and faster than the model predicts.
The Path Forward: Navigating Uncertainty in Network Analysis
While the challenges posed by measurement error are significant, they aren't insurmountable. By acknowledging the limitations of our data and employing strategies to mitigate the impact of inaccuracies, we can harness the power of network analysis to make more informed decisions. Widespread early detection strategies can help, and further research into new methods will help.