Is Your City Growing Old? Unveiling the Secrets of Age Distribution and Spatial Influence
"Explore how functional spatial autoregressive models reveal the hidden connections shaping age demographics in urban landscapes."
The makeup of a city's population, particularly its age distribution, is a critical factor in understanding its economic vitality, social dynamics, and future prospects. Cities across the globe are experiencing significant shifts in their age demographics, driven by factors ranging from economic opportunities and healthcare access to social policies and cultural attractions. Understanding these shifts is crucial for policymakers, urban planners, and community leaders seeking to create vibrant, sustainable, and equitable urban environments.
Traditional methods of demographic analysis often fall short when capturing the complex interplay of factors that influence age distribution. These methods typically focus on isolated variables, neglecting the spatial interdependence between neighboring regions. A city's population isn't just a product of its internal characteristics; it's also shaped by the flows of people, resources, and ideas across geographic boundaries.
Enter functional spatial autoregressive models: a cutting-edge approach that combines the strengths of functional data analysis and spatial econometrics to provide a more holistic view of urban demographics. By considering age distribution as a function and accounting for spatial autocorrelation, these models offer valuable insights into the intricate forces shaping our cities.
What is Functional Spatial Autoregressive Modeling?
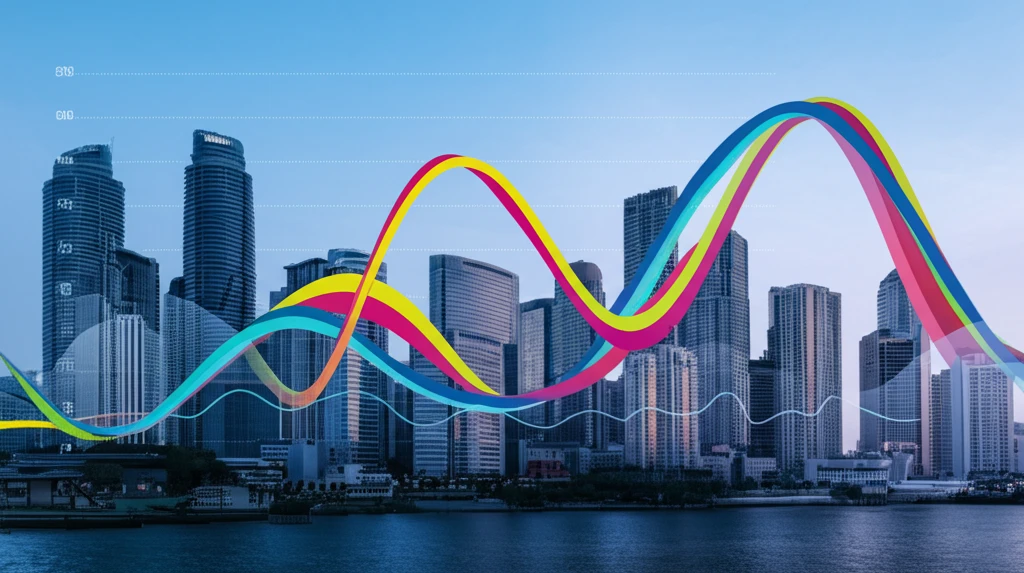
Functional spatial autoregressive (SAR) models represent a significant advancement in how we analyze data that varies across both space and a continuous function – in this case, a city's age distribution. Imagine each city not just as a single point on a map, but as a curve representing the proportion of residents at every age. Functional SAR models allow us to examine how these age distribution curves are influenced by the curves of neighboring cities, while also accounting for local socioeconomic factors.
- Simultaneous Integral Equation System: The model is characterized as a simultaneous integral equation system, which requires careful consideration to ensure a unique and stable solution.
- Regularized Two-Stage Least Squares (2SLS) Estimator: To address endogeneity issues arising from spatial interactions, the model employs a regularized two-stage least squares estimator, based on a basis approximation for the functional parameter.
- Wald-Type Test: A simple Wald-type test is proposed for detecting the presence of spatial effects, adding a statistical tool to validate the model's findings.
Implications for Urban Planning and Policy
The findings from functional spatial autoregressive models offer valuable insights for urban planning and policy. By understanding the factors that drive age distribution and the spatial connections between cities, policymakers can develop targeted interventions to address demographic challenges. For example, cities facing an aging population can implement policies to attract younger residents, such as affordable housing initiatives or investments in education and job training. Similarly, understanding the spatial spillover effects of economic growth can help policymakers coordinate regional development strategies to promote balanced and sustainable growth.