Hidden Truths: Are Underreported Benefits Distorting Economic Research?
"Unveiling the Impact of Data Imperfections on Program Effectiveness Studies"
Economic research heavily relies on government benefit data to assess program impacts, often employing instrumental variable (IV) methods to address inherent participation biases. However, the accuracy of this data is increasingly compromised by underreporting and imputation, where benefit amounts are either understated or estimated due to missing information. This poses a significant challenge to the validity of research findings, potentially leading to flawed conclusions about program effectiveness.
The problem of inaccurate benefit data is two-fold. First, households may underreport the benefits they receive, either intentionally or due to recall errors. Second, when data is missing, researchers often use imputed values, which are estimates based on statistical models. While imputation aims to fill the gaps, it introduces its own set of biases, especially if the imputation methods don't fully capture the complexities of benefit distribution.
This article delves into how these data imperfections can distort research outcomes, particularly when using IV methods. We'll explore how underreporting and imputation can lead to overstated program impacts, examine real-world examples from Social Security and child benefit programs, and provide practical guidance for researchers aiming to mitigate these biases.
Why Underreporting and Imputation Matter for Accurate Analysis
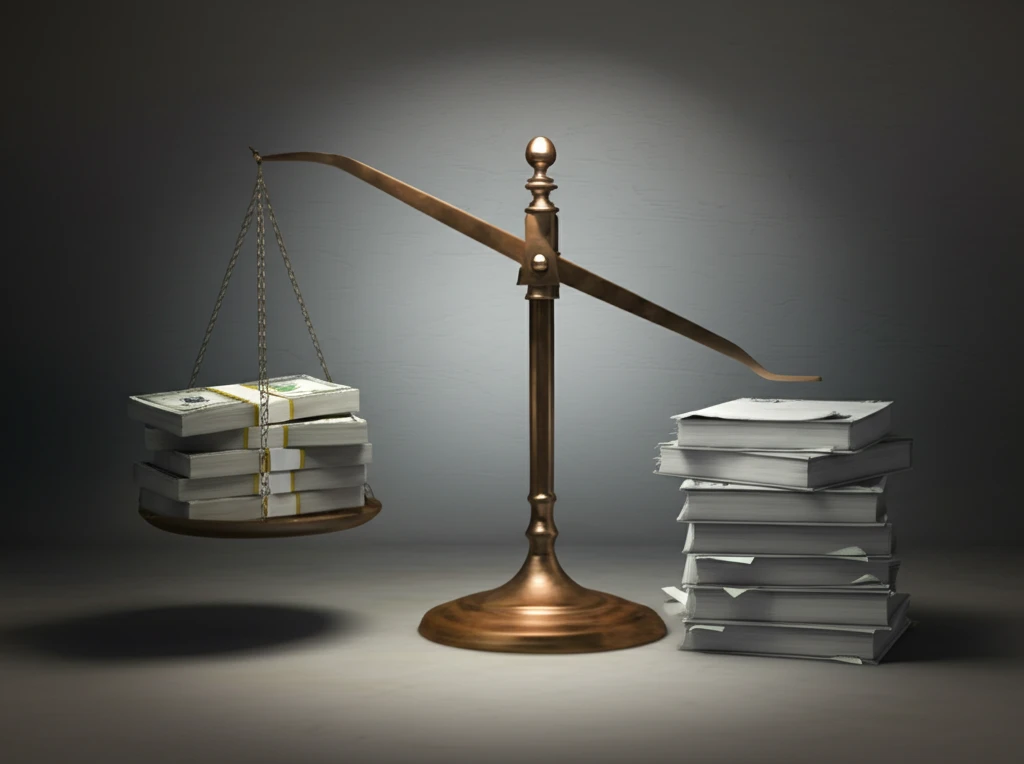
When instrumental variables are used to estimate the impact of government benefits, they rely on the assumption that the instrument is correlated with the actual benefit amount. However, when benefits are underreported or imputed, this correlation weakens, leading to biased estimates. Imagine trying to measure the effectiveness of a job training program using attendance records that are only partially filled in—the results would likely be misleading.
- Attenuation Bias: Underreporting reduces the observed variation in benefit amounts, weakening the relationship between the instrument and the benefit.
- Imputation Bias: If the imputation model doesn't fully capture the factors influencing benefit receipt, the imputed values will be systematically different from the true values.
- Ratio Bias: IV estimates are calculated as the ratio of two coefficients. If the denominator (the effect of the instrument on the benefit) is biased downwards due to underreporting, the overall IV estimate will be biased upwards.
Mitigating Bias: A Call for Rigorous Data Handling
The prevalence of underreported and imputed data poses a serious challenge to economic research. Researchers must be vigilant in identifying and addressing these biases to ensure the accuracy of their findings. This requires a combination of methodological rigor, careful data handling, and a thorough understanding of the underlying data-generating processes. By acknowledging and mitigating these biases, we can move towards more informed and effective policy decisions.