Global vs. Individual? How to Get the Best of Both Worlds in Statistical Testing
"Researchers often face the dilemma of choosing between global and individual hypothesis testing. Discover how a new combined approach can optimize your statistical power and ensure robust results."
In the realm of economic research, studies often involve testing multiple hypotheses simultaneously. Researchers aim to evaluate both the collective and individual evidence supporting or refuting these hypotheses. This dual objective requires a delicate balance in choosing the appropriate statistical methods.
Traditionally, practitioners have relied on two main classes of tests: those using quadratic test statistics (QF tests), such as F-tests and Wald tests, and those based on minimum/maximum type test statistics. Each has its strengths and weaknesses. QF tests are powerful for detecting overall effects but may not pinpoint specific individual effects. Minimum/maximum tests, on the other hand, excel at identifying individual effects while controlling for multiplicity but may lack power in detecting broad, subtle effects.
Recognizing the limitations of each approach, a recent paper introduces a combination test that merges these two classes using the minimum p-value principle. This innovative method capitalizes on the global power advantages of QF tests while retaining the stepdown procedure benefits of minimum/maximum type tests.
Why Choose? Combining Global and Individual Testing for Optimal Results
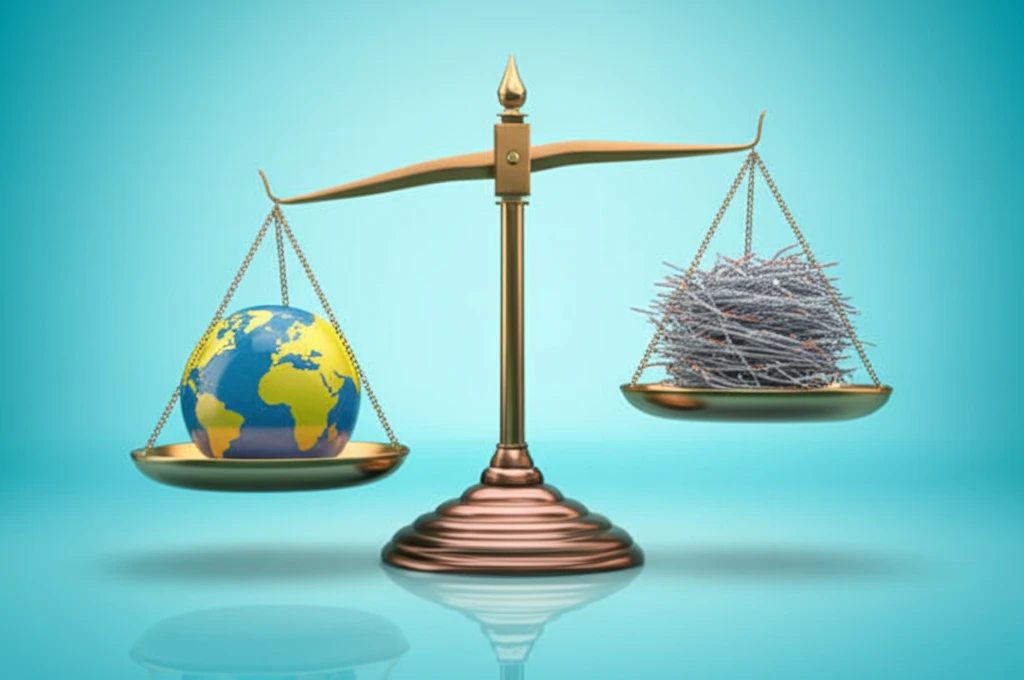
The core challenge in statistical testing lies in balancing the desire to detect any true effects (power) while minimizing the risk of false positives (Type I error). Global tests, like F-tests, are designed to assess the overall significance of a set of hypotheses. They are particularly effective when many small individual effects accumulate to create a significant overall effect. However, a significant global test result doesn't necessarily tell you which specific individual hypotheses are driving the effect.
- Global Power: Detects overall effects arising from the accumulation of small individual effects.
- Individual Specificity: Identifies significant individual treatment effects while controlling Type I errors.
- FWER Control: Preserves the control of familywise error rate (FWER) by the MinP test.
The Best of Both Worlds
The combined test offers a robust and versatile approach to hypothesis testing, suitable for various research settings. By leveraging the strengths of both global and individual tests, researchers can achieve a more comprehensive and reliable understanding of their data, ultimately leading to more informed conclusions and better decision-making.