GDP Under the Microscope: Are Our Economic Models Missing the Mark?
"New research challenges the traditional ways we measure economic growth, suggesting our current models may be too simplistic."
For decades, economists have relied on aggregate production functions (APF) to link a nation's output to its physical capital and labor inputs. These functions, which treat capital and labor as the primary drivers of economic production, have become a cornerstone of macroeconomic theory and economic growth literature. However, this approach is not without its critics. Many economists argue that the very concept of aggregate production functions is flawed, leading to a re-evaluation of how we model and interpret economic growth.
The Cobb-Douglas functional form has persisted in theoretical and empirical models of economic growth due to its historical roots, simplicity, and perceived data fit. A more general and popular form is the translog function, a second-order local approximation. Today, APF is used in international growth comparisons and decomposing economic growth into its sources. These analyses primarily address modelling and statistical inference rather than the theoretical underpinnings of empirical models.
Recent research introduces a purely Bayesian vector autoregression (VAR) framework to formulate and compare models. This approach formulates tri-variate models for economy-wide output and inputs, rooted in aggregate production function theory while accounting for macroeconomic data's dynamic properties. This methodology pits theoretical time-series models against those based on aggregate production function-type relations, using common knowledge about capital and labor elasticities to inform prior distributions. Despite favoring linearly homogenous Cobb-Douglas production functions, the study found that production function-based co-integration models do not outperform simple VAR structures.
Why Traditional Production Models Might Be Misleading Us
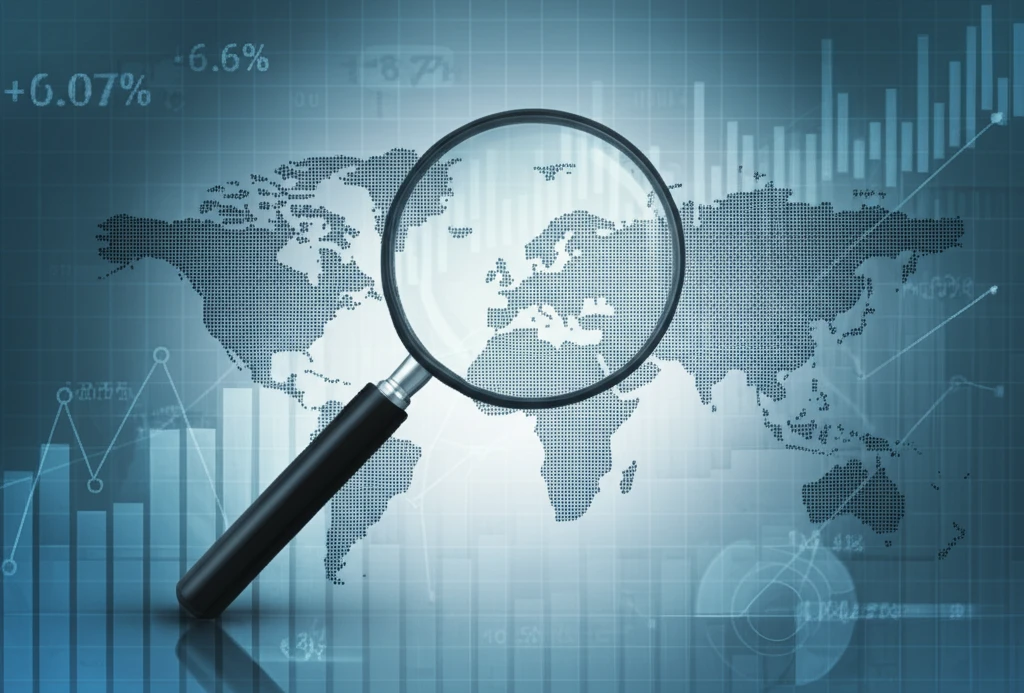
While microeconomic production functions are effective models for describing the technology of individual producers, aggregating physical capital, labor, and output into meaningful measures poses significant challenges. The relationship between these aggregates can appear suspicious, leading some to question the validity of simplistic models. Attempts to derive aggregate production functions from micro-foundations often rely on extremely specific assumptions.
- Data and Methodology: Annual data from Penn World Tables was used, focusing on GDP, capital stock, and total hours worked. The study employed a Bayesian vector autoregression (VAR) framework to compare models.
- Model Comparison: Theoretical time-series models were compared against those grounded in aggregate production function-type relations. Prior distributions were informed by knowledge of capital and labor elasticities.
- Key Finding: Production function-based co-integration models were outperformed by simpler VAR structures, suggesting that traditional production models may not accurately capture economic dynamics.
The Future of Economic Modeling: Embracing Simplicity?
The study underscores the need for economists and policymakers to critically evaluate the models they use to understand economic growth. While aggregate production functions have been a mainstay of economic theory, empirical evidence suggests that simpler, time-series-based models may provide a more accurate representation of economic dynamics. As the quest for better economic models continues, embracing parsimony and focusing on empirical fit may lead to more reliable insights into the forces driving economic growth.