Game Theory on the Grid: How Spatial Dynamics Change the Rules of Evolution
"Uncover how spatial arrangement affects strategic interactions, impacting everything from ecology to economics."
Evolutionary game theory, traditionally examining strategy selection in populations, has largely overlooked the impact of spatial arrangement. Classic models often assume a well-mixed population, where every player interacts equally with all others. However, real-world interactions rarely occur in such idealized settings. Individuals are often situated in specific locations, interacting primarily with their neighbors. Think of animals in an ecosystem, businesses in a market, or even individuals in a social network—all influenced more by their immediate surroundings than by the entire population.
A study published in the journal Electronic Journal of Probability delves into this spatial dimension, exploring how local interactions on a lattice structure—a grid-like network—alter the outcomes of evolutionary games. This research uses a 'best-response dynamic,' where players adjust their strategies to maximize their individual payoff, considering only the strategies of their immediate neighbors. This approach contrasts sharply with nonspatial models where decisions are based on the overall population strategy frequencies.
The implications of this spatial perspective are significant. The study reveals that what makes a strategy 'stable' in a well-mixed population is fundamentally different from what ensures its survival in a spatially structured environment. This divergence has profound implications for understanding phenomena ranging from the evolution of cooperation to competitive strategies in business.
Why Does Space Matter in Evolutionary Games?
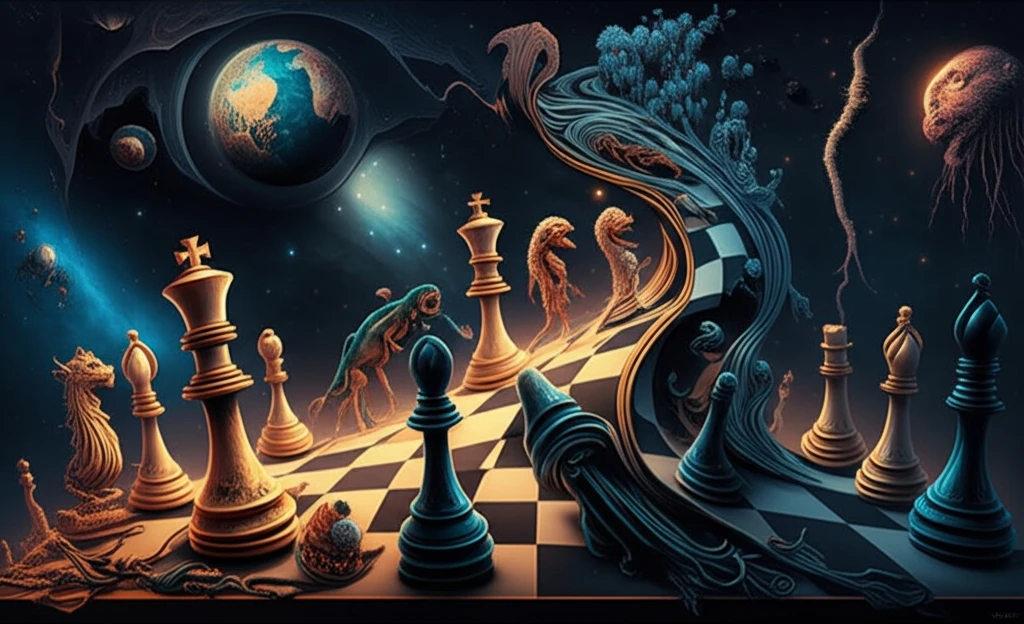
In classic evolutionary game theory, the concept of an 'evolutionary stable strategy' (ESS) is central. An ESS is a strategy that, if adopted by a population, cannot be invaded by any alternative strategy. This means that even if a few individuals start using a different strategy, the original ESS will persist. However, this concept is largely based on the assumption of a well-mixed population. In reality, spatial constraints introduce critical nuances. For instance, a cooperative strategy might be vulnerable in a well-mixed population because defectors can exploit the cooperators. But in a spatial setting, clusters of cooperators can form and protect each other, making cooperation a viable strategy.
- Mean-Field Approximation: The study first examines a nonspatial, or mean-field, approximation of the game. This involves assuming that the population is well-mixed, allowing researchers to derive deterministic equations for the frequencies of each strategy.
- Spatial Model: The core of the research involves a spatial model, where players are located on an infinite square lattice and interact only with their immediate neighbors. This introduces a stochastic element, as individual decisions are influenced by local interactions.
- Selfish vs. Altruistic Strategies: The study classifies strategies as 'selfish' or 'altruistic' based on how they affect the payoffs of neighboring players. This classification plays a critical role in determining the stability of different strategies in both spatial and nonspatial models.
Beyond the Lattice: Real-World Implications
While this study focuses on a lattice structure, the principles extend to any networked population where interactions are localized. The key takeaway is that the architecture of interactions profoundly affects strategic outcomes. Businesses competing in a local market, individuals spreading ideas through a social network, or even immune cells interacting within a tissue all operate under spatial constraints that shape their strategic choices and ultimate success. Recognizing the importance of spatial dynamics offers a more nuanced and realistic understanding of competition and cooperation in complex systems.