Do AI Agents Play Nice? Unveiling the Social Behavior of Language Models
"New research explores whether AI language models exhibit fairness, reciprocity, and cooperation – or just look out for number one."
Large Language Models (LLMs) are rapidly transitioning from behind-the-scenes tech to active participants in our digital lives. They're powering everything from customer service chatbots to AI assistants that help manage our schedules. But as these AI systems take on more autonomous roles, a crucial question arises: how do these AI agents behave in social contexts? Do they exhibit traits like fairness, reciprocity, and cooperation, or are they primarily driven by self-interest?
Understanding the social behavior of LLMs is essential for building trust and ensuring these technologies are used responsibly. If an LLM is deployed in a situation requiring negotiation or resource allocation, will it act fairly? Will it be susceptible to biases that could lead to inequitable outcomes? These are the questions that motivate a growing body of research aimed at uncovering the inner workings of these complex systems.
Now, a groundbreaking study is shedding light on this critical area. By applying concepts from behavioral economics, researchers have developed a novel framework to analyze LLMs' responses in social contexts. The findings reveal surprising insights into the social tendencies of these AI agents, challenging some common assumptions and raising important questions about the future of AI.
Decoding AI's Social Compass: The SUVA Framework
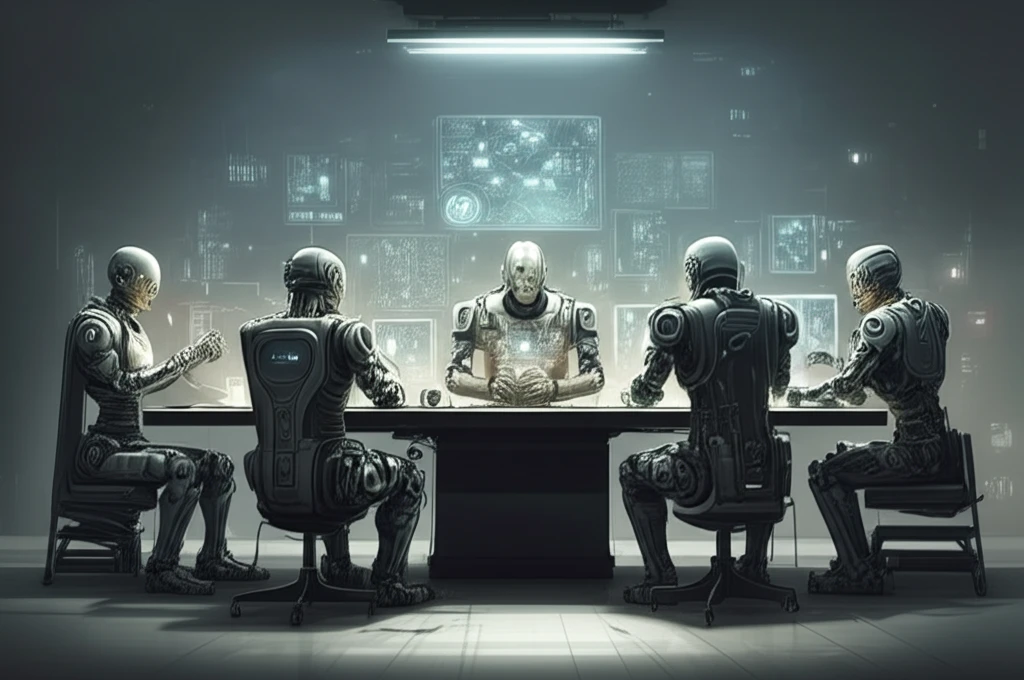
The key to unlocking the social behavior of LLMs lies in a new framework called "State-Understanding-Value-Action" (SUVA). This probabilistic model analyzes LLM responses based on their textual outputs, or utterances, in social contexts. It draws upon established behavioral economics games, such as the dictator game, and social preference concepts relatable to LLM users to assess social behavior through both final decisions and response generation processes.
The Future of AI: Building Ethical and Cooperative Agents
This research marks a significant step towards understanding and shaping the social behavior of AI agents. By providing a framework for analyzing LLMs' responses in social contexts, it opens the door for developing more ethical and cooperative AI systems. As LLMs become increasingly integrated into our lives, these insights will be crucial for ensuring that AI benefits all of humanity.