Decoding Your Blood: How Machine Learning is Revolutionizing Hemoglobin Measurement
"Discover how cutting-edge 3D photonic structures and AI are making blood analysis faster, more accurate, and more accessible."
Imagine a world where blood tests are not only faster but also significantly more accurate. This future is rapidly becoming a reality thanks to the integration of machine learning into medical diagnostics. One fascinating application involves measuring hemoglobin, a critical component of our blood that carries oxygen. Traditional methods can be time-consuming and sometimes less precise, but new research is harnessing the power of artificial intelligence to revolutionize this process.
Hemoglobin levels are vital for diagnosing a range of conditions, from anemia to polycythemia. Accurate and timely measurement is crucial for effective treatment. Now, scientists are developing innovative techniques that combine 3D photonic structures with machine learning algorithms to achieve unprecedented precision in hemoglobin analysis. This technology promises to make healthcare more efficient and accessible.
This article delves into the groundbreaking research that is transforming hemoglobin measurement. We will explore how machine learning algorithms are trained to interpret data from 3D photonic structures, providing a faster, more accurate, and potentially more cost-effective way to assess blood health. Join us as we uncover the future of medical diagnostics.
The Science Behind the Innovation
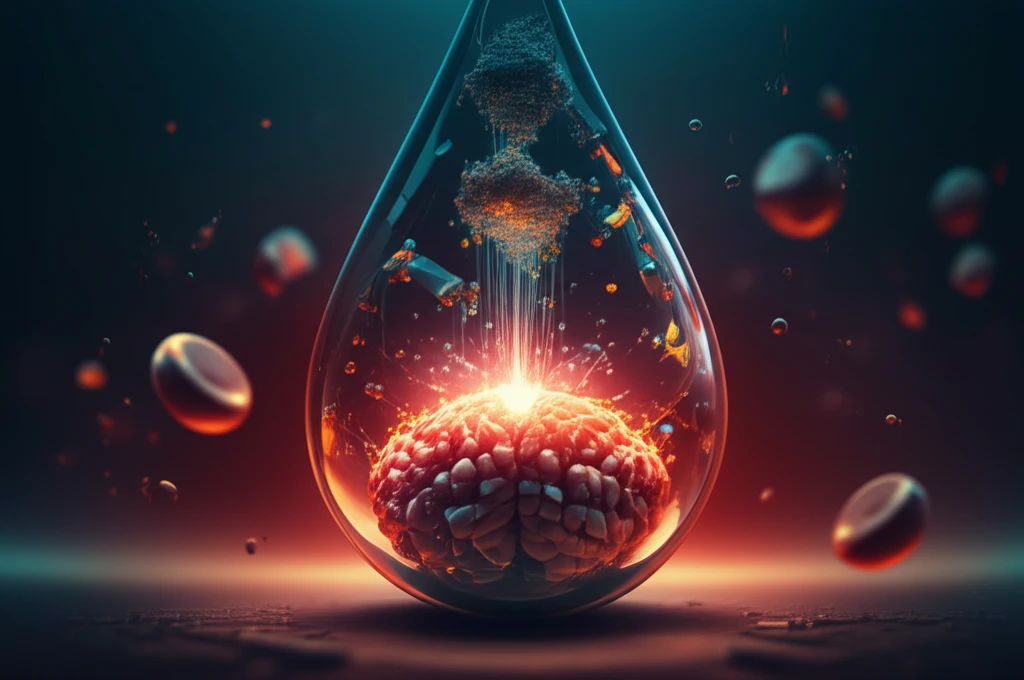
At the heart of this technological leap is the clever combination of photonics and machine learning. Researchers have designed intricate 3D photonic structures – essentially tiny, precisely engineered crystals – that interact with light in specific ways. When a blood sample is introduced to this structure, the way light passes through it changes depending on the hemoglobin concentration. This interaction creates a unique optical signature that can be captured and analyzed.
- 3D Photonic Structures: Precisely engineered crystals that interact with light based on hemoglobin concentration.
- Machine Learning Algorithms: Trained models that interpret optical signatures to predict hemoglobin levels.
- Linear Regression: A specific type of machine learning algorithm used for prediction.
- Data Analysis: The process of converting raw data into meaningful insights.
The Future of Blood Analysis
The integration of machine learning with 3D photonic structures represents a significant step forward in medical diagnostics. This innovative approach not only promises faster and more accurate hemoglobin measurements but also paves the way for a new generation of point-of-care testing devices. As technology continues to advance, we can expect even more sophisticated applications of machine learning in healthcare, leading to earlier diagnoses, more effective treatments, and ultimately, improved patient outcomes.