Decoding Uncertainty: How Confidence Sets Can Guide You Through Complex Economic Models
"Navigate the gray areas of economic parameters with confidence. Discover how Monte Carlo methods create reliable guides in uncertain landscapes."
In the realm of economics, it's rarely possible to know anything with absolute certainty. Economic models, particularly nonlinear ones, are often shrouded in doubt. This is where the concept of "partial identification" comes into play, acknowledging that we can't always pinpoint exact values for parameters but can instead define a range of plausible values. This uncertainty stems from various factors, including limitations in data, the complexity of economic systems, and the assumptions we make when building our models.
Think of it like trying to understand the inner workings of a complicated machine without a complete instruction manual. You might be able to observe how certain parts interact, but you won't necessarily know the precise role of each component or how they all fit together. Similarly, in economics, we often have to grapple with incomplete information and rely on statistical techniques to estimate the parameters that govern economic behavior.
One powerful tool for dealing with this uncertainty is the construction of "confidence sets." Instead of providing a single, definitive estimate for a parameter, a confidence set gives us a range of values that are likely to contain the true value. But how do we build these confidence sets, and how much can we trust them, especially when dealing with complex, nonlinear models? That's where Monte Carlo methods come in, offering a computationally intensive but remarkably effective approach to constructing reliable confidence sets for identified sets.
Monte Carlo Confidence Sets: A Roadmap Through Uncertainty
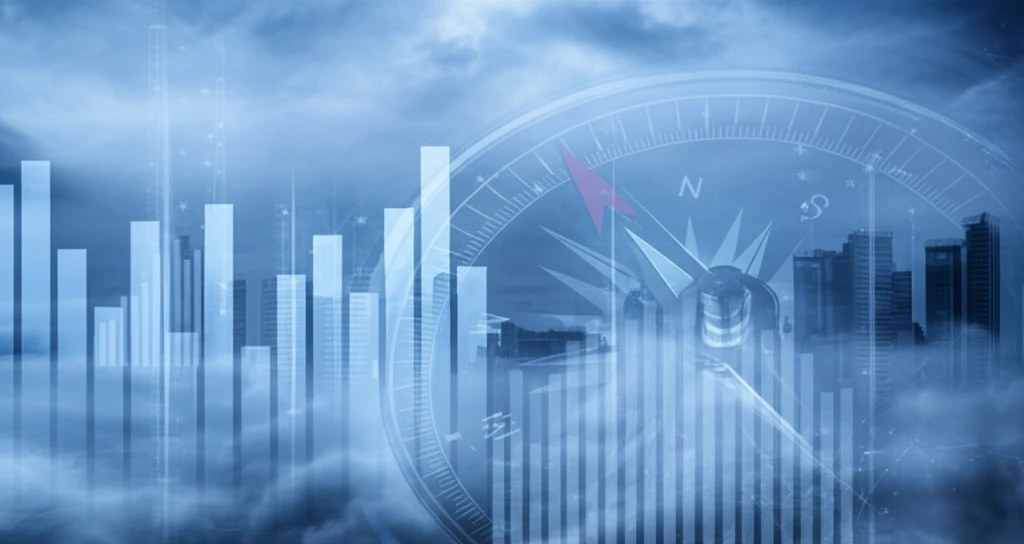
A recent research paper published in Econometrica sheds light on innovative procedures for building confidence sets for partially identified parameters. The method uses computationally attractive procedures to construct confidence sets (CSs) for identified sets of the full parameter vector and of subvectors in models defined through a likelihood or a vector of moment equalities or inequalities. The core innovation lies in using Monte Carlo (MC) simulations to construct these confidence sets, drawing on the "quasi-posterior" distribution of the criterion function.
- Computational Efficiency: The Monte Carlo approach is computationally feasible, even for complex models.
- Accuracy: The resulting confidence sets have accurate coverage probabilities, meaning that they contain the true parameter values with the stated level of confidence.
- Flexibility: The method can be applied to a wide range of models, including those defined through likelihood functions or moment conditions.
- Robustness: The confidence sets are valid even when the model is only partially identified.
Navigating the Landscape of Economic Uncertainty
The rise of Monte Carlo Confidence Sets provides a more robust and reliable way to assess the range of plausible outcomes in complex economic situations. Whether you're an economist, a policymaker, or simply someone trying to understand the forces shaping the economy, embracing these tools can lead to more informed decisions and a greater appreciation for the inherent uncertainty of the world around us.