Decoding the Stock Market: How 'Mesoscopic' Analysis Can Improve Your Portfolio
"Move Beyond Traditional Metrics to Optimize Investments with Community-Based Portfolio Strategies"
The stock market can often feel like a chaotic arena, with countless factors influencing the performance of your investments. Modern portfolio theory, which began with the pioneering work of Markowitz in 1952, aims to bring order to this chaos by using mean-variance (MV) optimization. This approach seeks to balance risk and return, helping investors construct portfolios that maximize potential gains while minimizing potential losses. However, despite its theoretical appeal, the MV framework often falls short in practice, delivering suboptimal out-of-sample performance.
One major challenge with the traditional MV approach is its sensitivity to estimation errors, especially in the correlation matrix, which captures how different assets move in relation to each other. These errors can lead to skewed portfolio allocations and disappointing results. In fact, some research suggests that a simple 1/N heuristic, where you allocate equal weight to each asset in your portfolio, can often outperform the sophisticated MV optimization due to its lower susceptibility to these errors. This has led researchers to investigate methods to improve the accuracy and reliability of portfolio construction.
Recent research has explored advanced techniques to filter noise and capture the 'real' essence of the correlation matrix, leading to better portfolio outcomes. This article delves into an innovative approach that uses "mesoscopic" analysis, focusing on an intermediate level of market structure to enhance portfolio optimization. By understanding the mesoscopic structure, which lies between the microscopic (individual stock) and macroscopic (overall market) levels, investors can potentially achieve more stable and reliable portfolios.
What is Mesoscopic Structure in the Stock Market?
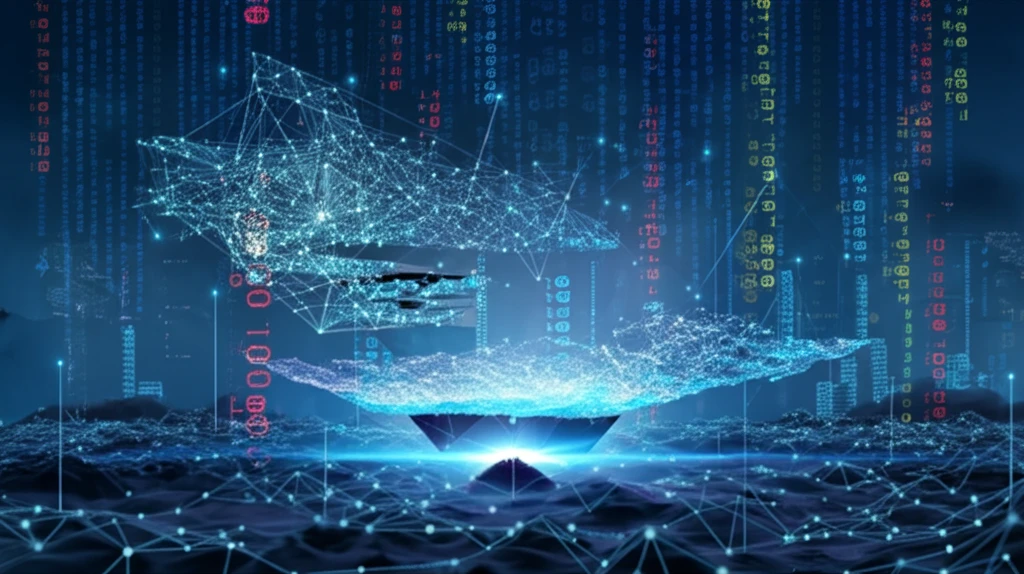
The research paper introduces the concept of mesoscopic structure as an intermediate level of market analysis that bridges the gap between individual stock behavior and overall market trends. This approach aims to identify stable, interconnected groups of stocks (communities) that offer valuable insights for portfolio construction. The process involves:
- Random Matrix Theory (RMT): Filters out random noise in correlation matrices to uncover true relationships.
- Systemic Co-movement Removal: Identifies and removes market-wide trends for clearer insights.
- Community Detection: Groups stocks into interconnected communities for focused analysis.
The Future of Portfolio Management
The research presented offers a compelling case for incorporating mesoscopic analysis into portfolio management strategies. By filtering out noise and identifying stable communities of stocks, investors can build more reliable portfolios that are less susceptible to market fluctuations. This approach not only improves risk management but also provides a framework for understanding the complex interdependencies within the stock market. As the financial landscape continues to evolve, embracing innovative techniques like mesoscopic analysis will be crucial for investors seeking to achieve consistent, long-term success.