Decoding the Secrets of Single-Cell Data: How Interpretable Clustering Can Revolutionize Research
"DendroSplit offers a new way to cluster single-cell RNA-Seq datasets, emphasizing interpretability and addressing the subjective nature of cell type definitions."
Single-cell RNA sequencing (scRNA-seq) has become a cornerstone of modern biological research, allowing scientists to delve into the intricate world of individual cells. This powerful technology is used to study cellular differentiation, explore known cell types, and even discover entirely new cell populations and their unique gene expression patterns. The result is a vast amount of data that holds the key to understanding complex biological processes.
However, analyzing this data presents significant challenges. scRNA-seq datasets are characterized by high dimensionality, missing data (drop-out events), and sheer size – often encompassing tens of thousands, or even millions, of cells. After scientists gather this data, their goal is to group individual cells based on their gene expression profiles, identifying known cell populations and uncovering novel or rare cell types. The catch is that there are a ton of different ways to do this.
Enter DendroSplit, a novel framework designed to tackle these challenges head-on. Unlike many existing methods that rely on complex parameters and lack clear interpretability, DendroSplit offers a more intuitive and transparent approach to clustering scRNA-seq data. It directly addresses the inherent subjectivity in defining “cell types” and provides researchers with valuable insights into the biological meaning of their clusters.
Why is Interpretable Clustering a Game Changer?
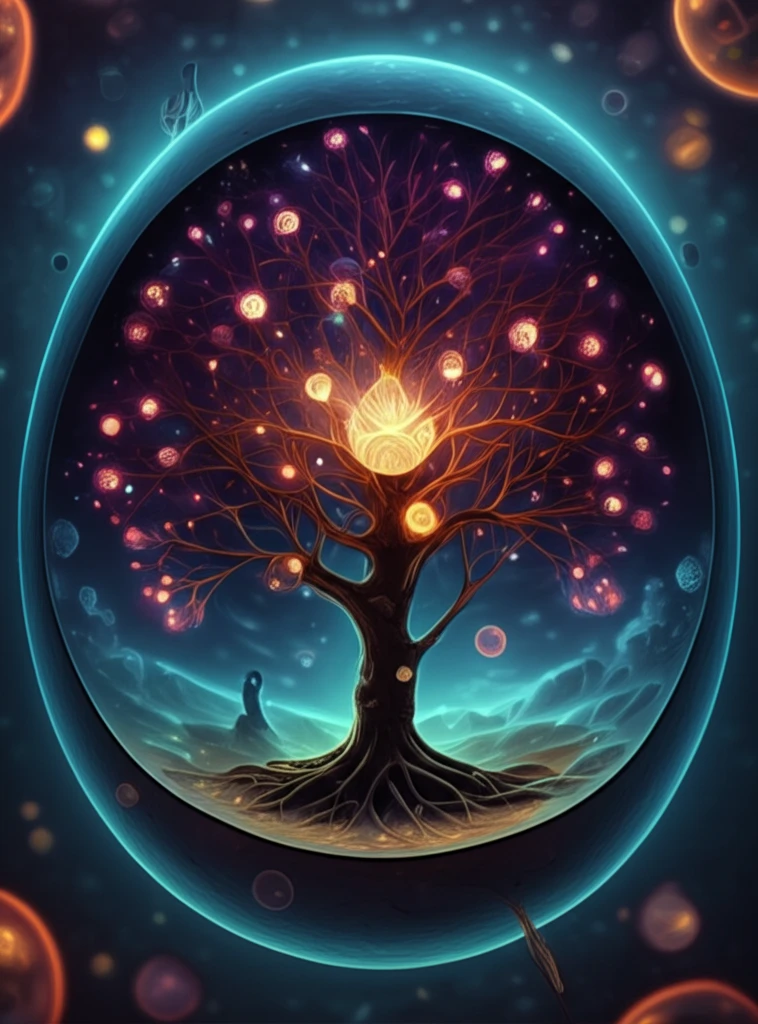
Traditional clustering methods often require significant preprocessing and fine-tuning of non-intuitive parameters. Algorithms like K-means or spectral clustering depend on knowing the number of clusters beforehand, while others, such as DBSCAN or affinity propagation, involve parameters that are difficult to interpret biologically. Finding the right combination of algorithm and parameters can be time-consuming and frustrating, without even fully solving the problem.
- Gene-Based Justification: Every decision made during cluster generation is grounded in gene expression data, providing biological context for each split and merge.
- Interpretable Parameters: DendroSplit uses intuitive parameters, making it easier to understand and control the clustering process.
- Multiple Clusterings: The framework allows for the cheap generation of multiple clusterings from the same dataset, enabling exploration of different perspectives.
- Workflow Integration: DendroSplit can be easily incorporated into existing scRNA-seq analysis pipelines.
Looking Ahead: The Future of Single-Cell Data Analysis
DendroSplit represents a significant step forward in making single-cell data analysis more accessible and interpretable. As scRNA-seq technology continues to advance, with increased cell throughput and larger datasets, frameworks like DendroSplit will be essential for extracting meaningful insights and driving biological discoveries. By emphasizing interpretability and providing a flexible platform for exploration, DendroSplit empowers researchers to unlock the full potential of single-cell data and gain a deeper understanding of the cellular world.