Decoding the Overtime Puzzle: What Bunching Designs Reveal About Work Hours
"A Fresh Look at Mandatory Overtime and Its Real-World Impact on Labor Markets"
Microeconometrics constantly grapples with separating causal relationships from the tangled web of individual differences. This is particularly true when examining choices individuals make, like how many hours to work. It's tough to isolate how much of a work decision is due to genuine economic factors versus personal preferences. Economists frequently use methods like instrumental variables or studying policy changes over time. However, in many real-world scenarios, these tools aren't applicable, making it crucial to develop alternative methods for understanding how people respond to incentives.
One increasingly popular approach is the "bunching design" method. This technique focuses on situations where a large number of decision-makers face a sudden change in incentives at a specific threshold, such as overtime pay kicking in after 40 hours. By analyzing the distribution of choices around this threshold—specifically, how many people "bunch" up right before or at it—researchers infer how sensitive these individuals are to those incentives. This approach hinges on the idea that rational people will adjust their behavior in response to changes in costs or benefits, leading to observable patterns in their decisions.
However, the bunching design isn't without its critics. The method relies on combining a specific model of how people make choices with assumptions about how different individuals' preferences are distributed. Recent studies highlight that identifying causal effects using bunching hinges on assumptions about the distribution of preferences that can't be directly checked with real-world data. This creates a challenge: Can we really trust the conclusions drawn from bunching designs if the underlying assumptions are unverifiable?
Beyond Standard Models: A New Approach to Bunching
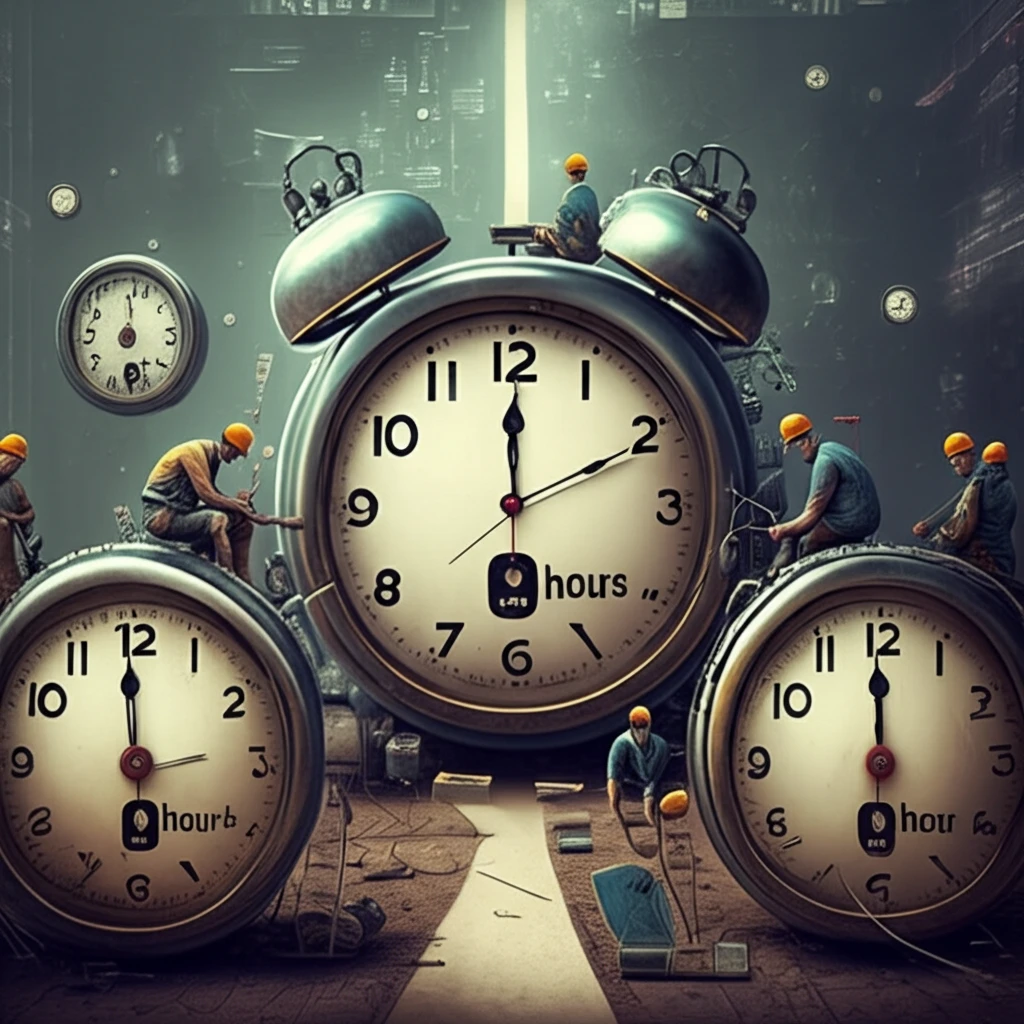
This study introduces a novel framework to tackle the identification challenges within bunching designs. Instead of relying on specific models of choice, it uses a more general approach that requires less restrictive assumptions. The key innovation involves reframing the problem as one of extrapolation: What would individuals' choices look like in counterfactual scenarios that we can't directly observe? In the context of overtime pay, this means estimating how many hours people would work if overtime regulations didn't exist.
- Shape Constraints: The research uses "bi-log-concavity" which imposes a weak shape constraint on each potential income distribution.
- Testable regions: Bi-log-concavity encompasses previous distributional assumptions and can be tested in observed outcome regions.
- Narrowed results: The resulting bounds provide significantly narrow average treatment effect (ATE) than conventional techniques by addressing each counterfactual individually.
The Broader Implications
This research offers a new perspective on the bunching design method, demonstrating its adaptability across diverse economic models. By emphasizing the importance of reduced-form average treatment effects and non-parametric assumptions, the study provides a robust framework for program evaluation. These insights are particularly valuable in contexts where traditional methods struggle, equipping researchers with the tools to analyze a wide range of policy questions and understand the intricate dynamics of economic decision-making.