Decoding the Oocyte: How Advanced Algorithms are Revolutionizing Fertility Treatment
"A look at the cutting-edge image processing techniques that could unlock new insights into oocyte quality and improve IVF success rates."
For those navigating the complexities of assisted reproductive technologies (ART), the journey can feel like piecing together a puzzle with many missing pieces. One of the most critical pieces is the oocyte, or egg, whose quality significantly impacts the success of fertilization and subsequent embryo development. While both male and female gametes contribute to the reproductive process, the oocyte holds a unique position, essentially setting the stage for new life.
Traditionally, assessing oocyte quality has relied on morphological features observed under a microscope. However, this method is subjective and limited by human perception. The rise of image processing techniques offers a more objective and detailed approach, promising to revolutionize how we evaluate oocytes and improve the outcomes of procedures like in vitro fertilization (IVF).
New research explores an advanced algorithm designed to describe and analyze the cytoplasm and polar body of mouse oocytes. While the study focuses on mice, the implications for human fertility treatment are significant. By using sophisticated image processing, researchers aim to identify subtle characteristics that correlate with oocyte health and developmental potential.
What Makes an Oocyte 'Good'? Unveiling the Algorithm's Approach
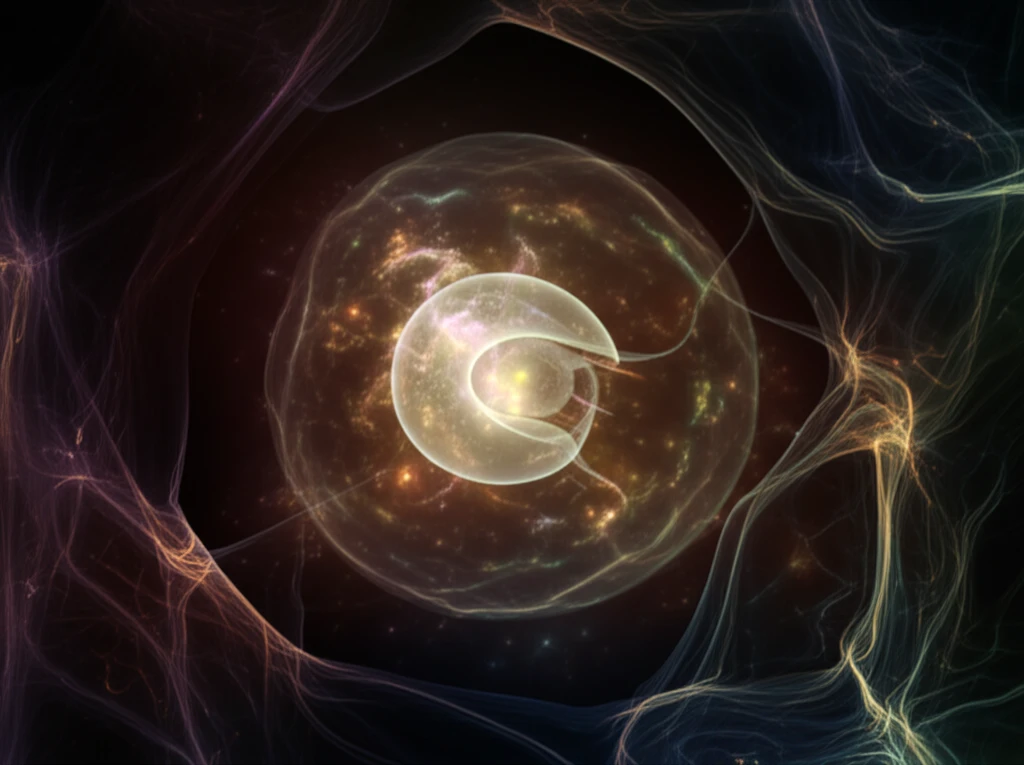
The key to this innovative approach lies in its ability to dissect the oocyte's structure with remarkable precision. The algorithm focuses on several critical areas:
- Segmentation: The algorithm begins by isolating the oocyte area using a technique called Moore neighborhood contour tracking. This method allows for precise detection of the oocyte's boundaries, even when they aren't perfectly circular.
- Feature Extraction: Once segmented, the algorithm analyzes the texture of the cytoplasm using a Gray-Level Co-occurrence Matrix (GLCM). This mathematical tool helps quantify the distribution and relationships of gray levels within the image, revealing subtle textural patterns that indicate oocyte quality.
- Clustering: Finally, the algorithm uses a self-organizing map (SOM) neural network to group oocytes into different clusters based on their textural features. This allows researchers to classify oocytes into categories such as low, medium, or high granularity, which can be indicative of their developmental potential.
The Future of Fertility: Precision and Personalization
This research represents a significant step forward in the quest to improve fertility treatment outcomes. By providing a more objective and detailed assessment of oocyte quality, these algorithms have the potential to personalize IVF treatment, selecting the most viable oocytes for fertilization and increasing the chances of a successful pregnancy. As technology advances, we can expect even more sophisticated image processing techniques to play a central role in the future of reproductive medicine, offering hope and improved outcomes for individuals and couples facing infertility.