Decoding the Financial Web: How Partial Mutual Information Can Revolutionize Market Analysis
"Move beyond traditional correlation methods and discover how advanced network analysis reveals hidden connections in financial markets, offering a fresh perspective for investors and analysts."
Financial markets are increasingly viewed as complex systems, where the interactions between various instruments are far from simple. Traditional methods of analysis often rely on linear correlations to understand these interactions. However, this approach overlooks the inherent nonlinearities that characterize market behavior. This is akin to trying to understand a symphony by only listening to the individual notes, missing the rich harmonies and dissonances that give it depth.
Enter the field of econophysics, which applies physics-based theories and tools to analyze economic phenomena. A central idea is that financial networks—representations of how different financial instruments relate to each other—can be better understood using concepts beyond simple correlation. One promising technique is partial mutual information (PMI), an advanced measure that captures both linear and nonlinear dependencies while controlling for the influence of other factors.
This article explores how PMI is transforming the analysis of financial networks, offering a more nuanced and potentially more accurate view of market dynamics. We'll break down the key concepts, discuss the advantages of this approach, and consider its practical implications for investors and analysts seeking an edge in today's complex markets.
Why Traditional Correlation Analysis Falls Short?
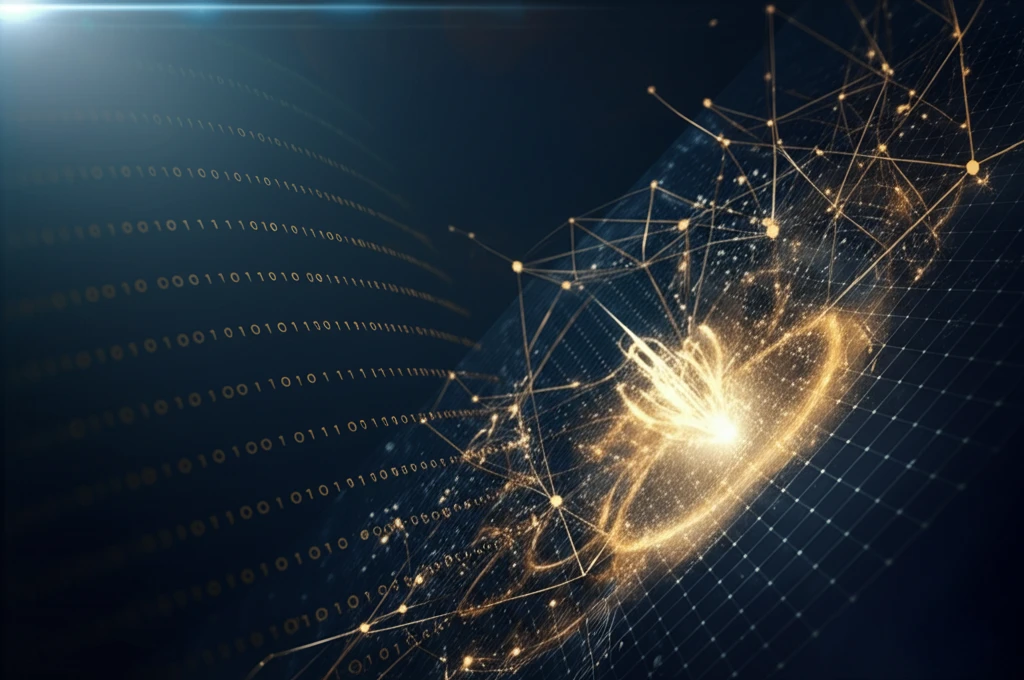
For years, financial analysts have relied on correlation to build network models of the stock market, foreign exchange markets, and more. Correlation measures the degree to which two variables move together. If stock A goes up when stock B goes up, they are positively correlated. If stock A goes up when stock B goes down, they are negatively correlated. While this provides a basic understanding of relationships, it has significant limitations:
- Ignores Complex Dependencies: Correlation only captures pairwise relationships and fails to account for how multiple variables interact. The influence of a third factor can mediate the apparent connection between two instruments.
- Limited Practical Usefulness: While correlation analysis can identify sectors within a market, this is often already known. True insight lies in understanding causal relationships and predicting market movements.
The Future of Financial Network Analysis
Partial mutual information is not a magic bullet, but it represents a significant step forward in understanding financial markets. By incorporating nonlinearity and controlling for mediating influences, PMI offers a more accurate and insightful view of market dynamics. As research continues, expect to see even more sophisticated techniques emerge, further refining our ability to navigate the complexities of the financial world.