Decoding the Economic Tea Leaves: Can AI Predict the Next Market Move?
"New research unveils a data-driven approach to mastering high-dimensional vector autoregressions, potentially revolutionizing economic forecasting and investment strategies."
In today's fast-paced economic landscape, predicting market fluctuations feels less like science and more like guesswork. Traditional methods often fall short when dealing with the vast amount of data that influences market behavior, leaving investors and economists struggling to make informed decisions. This is especially true with high-dimensional time series data, which involves analyzing numerous variables over extended periods – a task that can quickly overwhelm conventional statistical models.
However, a new wave of research is emerging that combines the power of data science with sophisticated machine-learning techniques to tackle this challenge head-on. One particularly promising area focuses on improving vector autoregressions (VAR), a statistical method used to capture the relationships between multiple time series. By enhancing VAR models with data-driven tuning parameter selection, researchers aim to unlock more accurate and reliable economic forecasts.
This article delves into a groundbreaking study that introduces a novel approach to tuning parameter selection for high-dimensional VAR models. We'll explore how this method, leveraging estimators like Lasso, post-Lasso, and square-root Lasso, offers a fully data-driven way to navigate complex economic data, potentially transforming how we understand and predict market trends.
The Quest for a Better Crystal Ball: How Data-Driven Techniques Enhance Economic Forecasting
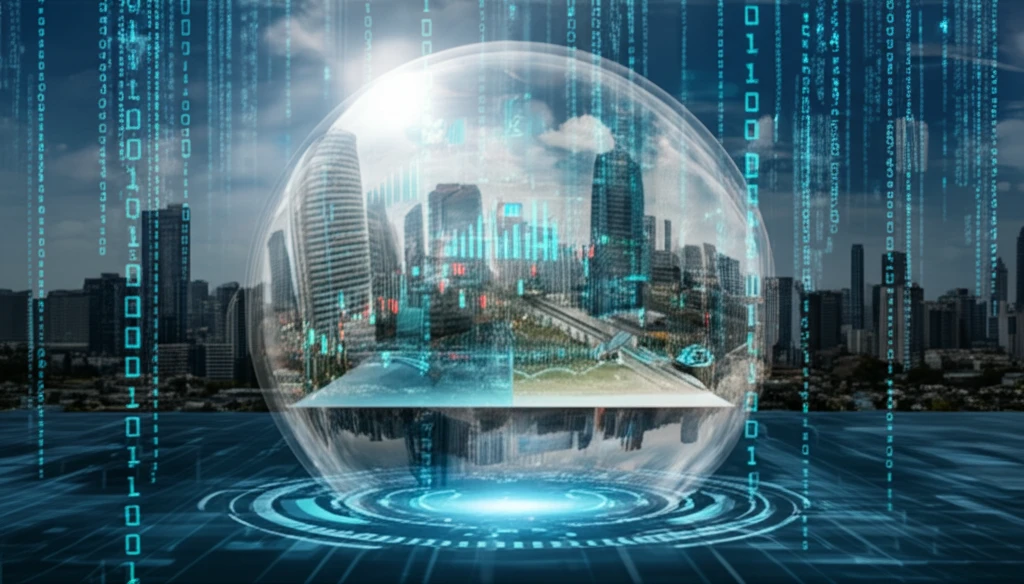
The core challenge in economic forecasting lies in the sheer volume and complexity of the data. High-dimensional vector autoregressions (VAR) offer a way to model the intricate relationships between multiple economic time series. However, these models often require careful tuning to avoid overfitting or underfitting the data. Overfitting leads to models that perform well on historical data but fail to predict future trends, while underfitting results in models that miss important patterns and relationships.
- Lasso Estimators: Automatically selects relevant variables and shrinks the coefficients of less important ones, preventing overfitting.
- Post-Lasso Estimators: Refines the Lasso estimates by re-fitting a least squares model using only the variables selected by the Lasso.
- Square-Root Lasso Estimators: An alternative to the Lasso that is less sensitive to the scale of the data.
The Future of Economic Prediction: A Glimpse into Tomorrow's Toolkit
This study represents a significant step forward in the quest for more accurate and reliable economic forecasts. By providing a fully data-driven approach to tuning parameter selection, it addresses a critical challenge in the application of high-dimensional VAR models. The theoretical guarantees established for the resulting estimation and prediction errors match those currently available for methods based on infeasible choices of penalization, paving the way for more robust and reliable economic predictions. As AI and machine learning continue to evolve, we can expect even more sophisticated tools to emerge, transforming how we understand and navigate the complexities of the global economy. This new generation of techniques promises to empower investors, policymakers, and economists with the insights they need to make informed decisions in an ever-changing world.