Decoding the Data: How Confidence Sets Can Reveal Hidden Market Realities
"Navigate Uncertainty in Complex Economic Models with Confidence Sets for Identified Sets"
In the realm of economic modeling, pinpointing the exact values of parameters is often a challenge. Unlike simpler scenarios where data directly translates to clear-cut conclusions, many real-world situations involve inherent uncertainties and complexities. This is especially true in nonlinear econometric models, where traditional methods may fall short. In these models, it's tough to say whether the parameters are point-identified. In plain language, point-identified parameters are those where the data gives us a clear, single value.
Economists and researchers need new tools to help them make sense of these situations. One such tool is the confidence set, which is a range of likely values for a parameter, rather than a single 'best guess.' Confidence sets acknowledge the uncertainty and provide a more realistic assessment of what the data is telling us.
This article aims to explain how confidence sets can be constructed and interpreted, even when the underlying economic models are complex and fraught with uncertainty. Recent research provides some helpful computationally attractive procedures to construct confidence sets (CSs) for identified sets of the full parameter vector and of subvectors in models defined through a likelihood or a vector of moment equalities or inequalities.
What are Confidence Sets and Why are They Important?
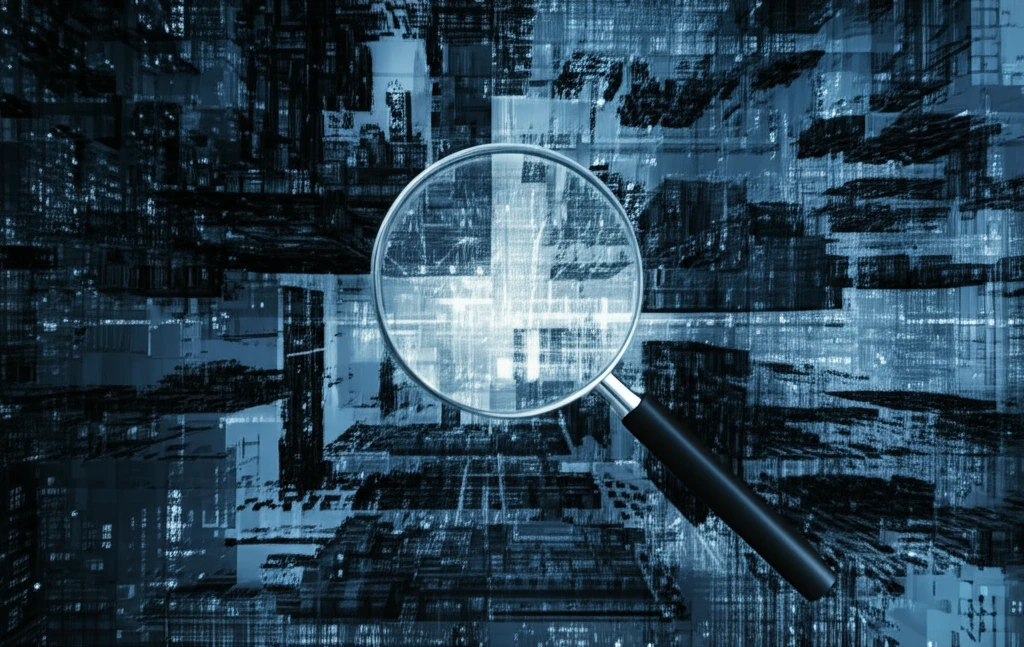
Confidence sets are, at their core, a way of acknowledging and quantifying uncertainty in statistical estimations. They are different from point estimates, which give a single value as the 'best' guess for a parameter. Instead, confidence sets provide a range of values within which the true parameter is likely to fall, given a certain level of confidence.
- Accounting for Uncertainty: Confidence sets explicitly acknowledge that economic data and models are subject to errors and limitations.
- Robustness Checks: They allow economists to assess how sensitive their conclusions are to different assumptions and data specifications.
- Informed Decision-Making: By providing a range of plausible values, confidence sets help policymakers and businesses make more informed decisions.
- Avoiding Overconfidence: They prevent the illusion of certainty that can arise from relying solely on point estimates.
The Future of Economic Analysis
As economic models continue to evolve in complexity, the need for robust and reliable methods of statistical inference will only grow. Confidence sets, with their ability to handle uncertainty and provide a more nuanced view of economic reality, are poised to play an increasingly important role in the field. By embracing these techniques, economists can gain a deeper understanding of the forces shaping our world and make more informed decisions for the future.