Decoding the Cosmos: How Cluster Radius Reveals Stellar Secrets
"Explore how astronomers use cutting-edge techniques to determine cluster radius and sampling radius in the determination of cluster membership probabilities of star clusters, refining our understanding of the universe."
Imagine sifting through grains of sand on a vast beach, each grain representing a star in the night sky. How do you identify which grains belong to a specific pile, a star cluster bound together by gravity and shared history? This is the challenge astronomers face when studying open clusters, groups of stars born from the same molecular cloud.
Modern astronomy relies on large-scale surveys that catalog millions of stars, making it possible to search for these stellar groupings with unprecedented detail. Identifying cluster members, however, isn't as simple as spotting a dense patch of stars. Stars within a cluster share similar motions and positions, but these characteristics can be muddied by observational errors and the presence of unrelated stars along the same line of sight.
To tackle this problem, astronomers use sophisticated algorithms like the one developed by Sanders in 1971. This method estimates the probability that a star belongs to a cluster based on its motion, comparing it to the overall motion of the cluster and the surrounding field stars. But even the best algorithms have limitations. One critical factor is the size of the area surveyed around the cluster, known as the sampling radius. Choosing the right sampling radius can make or break the accuracy of membership determinations. Let’s explore how astronomers optimize this crucial parameter to uncover the hidden secrets of star clusters.
The Delicate Balance: Cluster Radius and Sampling Radius
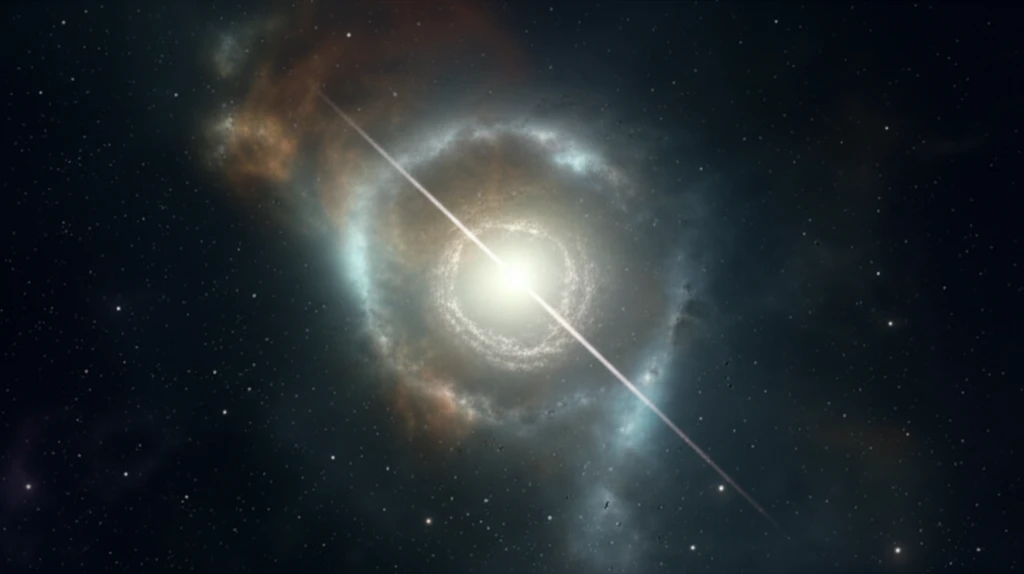
The core idea behind membership determination is to distinguish between cluster members and field stars based on their proper motions—how stars appear to move across the sky over time. Cluster members tend to share a common motion, while field stars exhibit a more random distribution. The Sanders algorithm uses bivariate normal distributions to model these motions, one circular distribution for the cluster and one elliptical for the field. The algorithm then calculates the probability that each star belongs to either distribution, assigning membership probabilities accordingly.
Unlocking the Secrets of the Cosmos
By carefully considering the interplay between cluster radius and sampling radius, astronomers can refine their techniques for identifying true cluster members. This improved accuracy allows for more reliable studies of stellar evolution, cluster dynamics, and the overall structure of our galaxy. Just as a skilled detective carefully examines a crime scene, astronomers must meticulously analyze star clusters to reveal their hidden stories.