Decoding Texture: How AI is Revolutionizing Color Image Analysis
"Unlock the secrets of AI-driven color texture classification and discover the innovative techniques shaping the future of image understanding"
In an era dominated by visual data, the ability to accurately and efficiently analyze images is more crucial than ever. From medical diagnostics to environmental monitoring, texture analysis plays a vital role in numerous applications. But as image datasets grow in size and complexity, traditional methods struggle to keep pace. This is where artificial intelligence (AI) steps in, offering a transformative approach to color texture classification.
AI techniques, particularly those involving machine learning and sparse representation, are revolutionizing how we extract meaningful information from images. These advanced methods not only reduce the computational burden but also enhance the accuracy of identifying and categorizing textures. Imagine a world where subtle anomalies in medical scans are instantly detected, or where satellite images are automatically analyzed to track deforestation with unprecedented precision. This is the promise of AI-driven texture analysis.
This article delves into the groundbreaking research that is making this vision a reality. We'll explore the innovative algorithms and methodologies that are enabling AI to decipher the intricate details of color textures, opening up new possibilities for image understanding and analysis across a wide range of industries.
The Evolution of LBP and the Rise of Sparse Representation
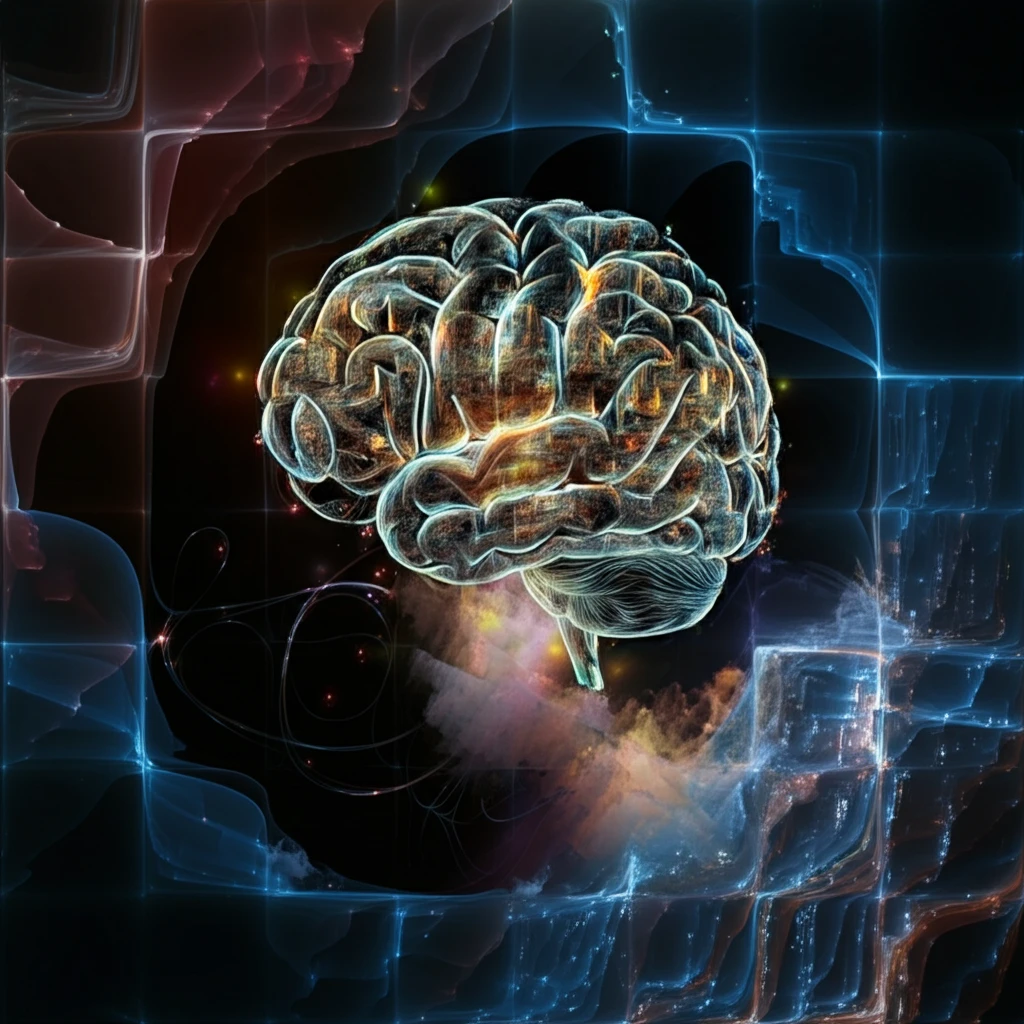
Local Binary Patterns (LBP) have long been a cornerstone of texture analysis due to their simplicity and effectiveness in capturing local image features. However, the traditional LBP operator often generates high-dimensional feature vectors, especially when dealing with color images or complex textures. This dimensionality challenge can lead to increased computational costs and decreased performance. To address this, researchers have been exploring dimensionality reduction techniques that preserve the discriminative power of LBP while minimizing its computational burden.
- Reduced dimensionality: Simplifies computations.
- Enhanced accuracy: Identifies the most important features.
- Robustness: Less susceptible to noise and irrelevant data.
The Future of AI-Driven Texture Analysis
The convergence of AI and texture analysis is poised to transform a wide range of industries, from medical imaging to remote sensing. As AI algorithms become more sophisticated and computational resources continue to expand, we can expect to see even more innovative applications of AI-driven texture analysis in the years to come. Embracing these advancements will be key to unlocking new insights and driving progress across diverse fields.