Decoding Stock Market Volatility: Factor Models to Navigate High-Dimension Challenges
"Unlock the secrets to forecasting market trends using advanced volatility models that simplify complex financial landscapes."
In today's fast-paced and ever-changing economic climate, understanding and predicting market volatility is more critical than ever. Both economists and investors rely on sophisticated tools to analyze the uncertainty inherent in financial time series. Among these tools, two classes of models stand out: the generalized autoregressive conditional heteroskedasticity (GARCH) model and the stochastic volatility (SV) model.
Multivariate GARCH (MGARCH) and Multivariate SV (MSV) models are particularly valuable as they capture time-varying covariance structures, which are essential for forecasting variance-covariance matrices. However, these models often struggle with the 'curse of dimensionality' – the explosive increase in the number of parameters as the data's complexity grows. This issue hampers estimation and worsens prediction accuracy due to overfitting.
To combat this challenge, researchers have turned to factor-based structures that incorporate a factor decomposition. This approach reduces dimensionality while maintaining the flexibility needed to capture essential features in financial data. This article explores the innovative factor Multivariate Stochastic Volatility (fMSV) framework, which offers a promising solution for high-dimensional volatility modeling.
How Do Factor Models Break Down Volatility?
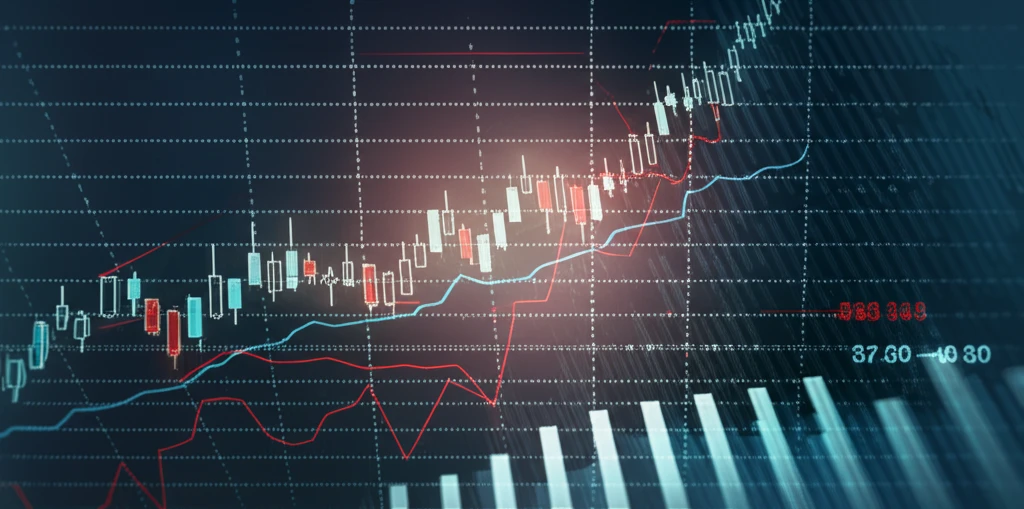
The fMSV framework builds on the idea of factor decomposition to address the challenges of high dimensionality. It uses two key viewpoints: the sparse approximate factor model and the sparse factor loading matrix. This dual approach allows for a more streamlined and efficient analysis of market volatility.
- Stage One: Estimators of the factor model are derived.
- Stage Two: The MSV component is estimated using the common factor variables identified in the first stage.
Why is Understanding fMSV Important?
The fMSV model represents a significant advancement in financial econometrics, offering a practical solution to the challenges of high-dimensional data. By combining factor models with stochastic volatility techniques, it provides a robust framework for forecasting market trends and managing risk. As financial markets continue to evolve, models like fMSV will become increasingly essential for investors and economists alike. This article aimed to translate highly technical findings for everyone to understand. Remember, keep a balanced view, always re-evaluate risk and reward and understand how a strategy or model actually works.