Decoding Stock Market Smiles: Can AI Fix Volatility's Calibration Conundrum?
"A Deep Dive into Reproducing Kernel Hilbert Spaces and Their Potential to Revolutionize Financial Modeling"
The world of finance is constantly seeking more accurate and reliable models to predict market behavior and price financial instruments. One of the most persistent challenges lies in calibrating these models to reflect real-world market conditions, especially when dealing with the inherent uncertainties of volatility. Traditional models often fall short, struggling to capture the nuances of market "smiles" and other complex patterns.
A recent research paper delves into an innovative approach using Reproducing Kernel Hilbert Spaces (RKHS) to address the limitations of existing financial models. This method focuses on singular local stochastic volatility McKean-Vlasov models, which are known for their ability to replicate option prices but also for their mathematical complexity and potential instability.
This article breaks down the key concepts of the research, explaining how RKHS techniques can be used to regularize these complex models, ensuring they remain stable and well-behaved. We will explore the potential of this approach to improve the accuracy and reliability of financial modeling, offering new insights into market dynamics and volatility.
What are Singular Local Stochastic Volatility McKean-Vlasov Models?
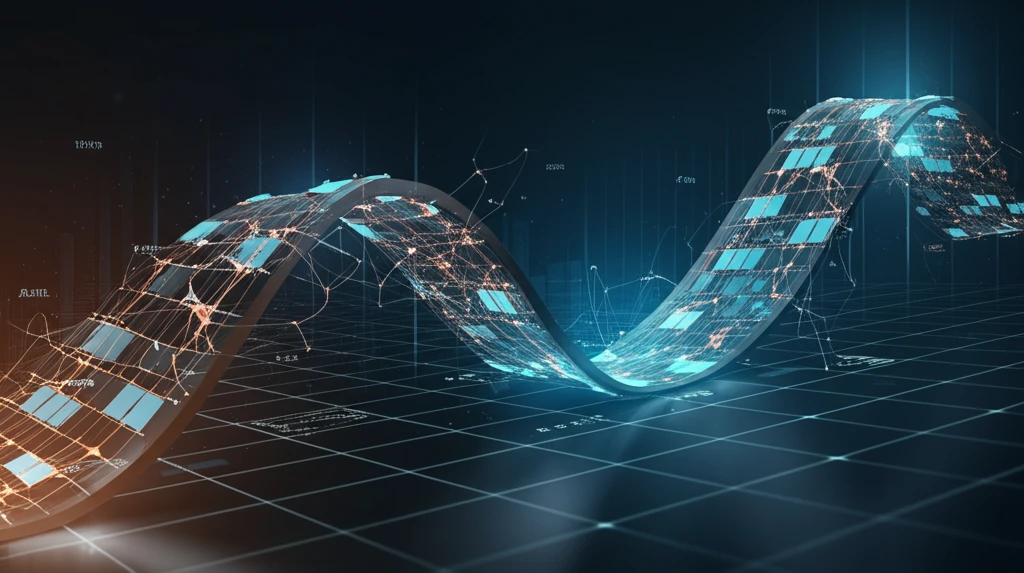
To understand the significance of the RKHS approach, it's important to first grasp the nature of the models it aims to improve. Singular local stochastic volatility McKean-Vlasov models are a type of financial model used to describe the behavior of asset prices, particularly in the options market. These models combine elements of both local volatility and stochastic volatility models, aiming to capture the best of both worlds.
- The Challenge of Calibration: Financial models need to accurately reflect real-world market conditions to be useful for pricing and risk management.
- The Complexity of Volatility: Volatility is a key factor in financial modeling, but it is also inherently uncertain and difficult to predict.
- The Need for Stability: Models must be stable and well-behaved to avoid producing unrealistic or unreliable results.
The Future of Financial Modeling: Embracing AI and Advanced Techniques
The research discussed in this article highlights the ongoing efforts to improve the accuracy and reliability of financial models. By leveraging advanced mathematical techniques like Reproducing Kernel Hilbert Spaces and incorporating AI-driven approaches, researchers are pushing the boundaries of what's possible in financial forecasting and risk management. While challenges remain, the potential benefits of these innovations are significant, promising more stable, accurate, and insightful tools for navigating the complexities of the modern financial landscape.