Decoding Stock Market Predictability: Can Quantile Regressions Help You?
"A Deep Dive into Predictive Models and Their Implications for Investors"
Predicting stock market movements has long been a holy grail for investors. While numerous studies have focused on forecasting mean stock returns, a more comprehensive approach considers the entire return distribution. This is where predictive quantile regressions come into play, offering a way to understand how different factors influence various points, or quantiles, of the return distribution, from the median to the extremes.
Traditional methods often struggle with issues like non-standard distributions and the persistence of predictor variables. Financial data, such as dividend yields or earning price ratios, tend to be highly autocorrelated and not strictly exogenous, leading to unreliable test results. This article delves into how a novel approach, the switching-fully modified (FM) test, addresses these challenges to provide more robust and accurate predictions.
This article will discuss the nuances of predictive quantile regressions, the difficulties in achieving reliable inference, and how the switching-FM test can overcome these obstacles. It highlights the practical implications for investors looking to make informed decisions in an uncertain market environment.
What are Predictive Quantile Regressions?
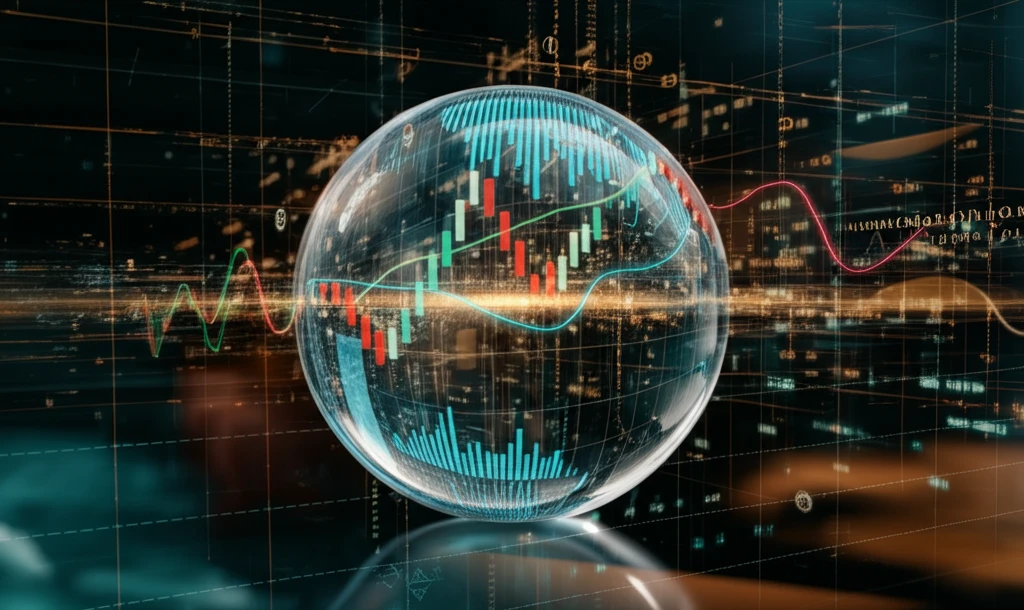
Predictive quantile regressions extend the standard regression framework to estimate the conditional quantiles of a dependent variable based on one or more predictors. Unlike ordinary least squares (OLS) regression, which focuses on the conditional mean, quantile regression can estimate the conditional median, quartiles, or any other quantile of interest. This is particularly useful in finance, where understanding the tails of a return distribution is crucial for risk management.
- Flexibility: Captures effects beyond the mean.
- Robustness: Less sensitive to outliers.
- Detailed Insights: Provides a more complete picture of how predictors affect returns.
Final Thoughts: Navigating Uncertainty with Quantile Regressions
Predictive quantile regressions, particularly when enhanced with methods like the switching-FM test, offer a robust framework for understanding and navigating the complexities of stock market prediction. While no model can guarantee foolproof forecasts, these advanced techniques provide investors with a more nuanced and reliable perspective on potential risks and rewards. As financial markets continue to evolve, embracing such sophisticated analytical tools can be a key to making more informed and resilient investment decisions.