Decoding Stochastic Partial Differential Equations: A Guide to Particle Representations
"Unlock the secrets of SPDEs with our comprehensive breakdown of particle representations and boundary conditions, making complex math accessible."
Stochastic Partial Differential Equations (SPDEs) are complex mathematical tools used to model phenomena that evolve randomly in space and time. Think of weather patterns, the spread of diseases, or the fluctuating prices in financial markets. These equations are notoriously difficult to solve, often requiring advanced numerical methods and computational power.
One innovative approach to tackling SPDEs is through "particle representations." Instead of directly solving the equation, this method uses a system of interacting particles to approximate the solution. Each particle carries information about the overall system, and their collective behavior mirrors the SPDE's dynamics. This is particularly useful when dealing with SPDEs that have specific boundary conditions—constraints on the solution at the edges of the domain being modeled.
This article explores how particle representations can be used to solve SPDEs, focusing on the challenges and techniques involved in handling boundary conditions. We'll break down the key concepts, explain the underlying math, and discuss how this approach can be applied to real-world problems. The goal is to provide a comprehensive yet accessible guide for anyone interested in understanding this powerful tool.
What are Stochastic Partial Differential Equations (SPDEs)?
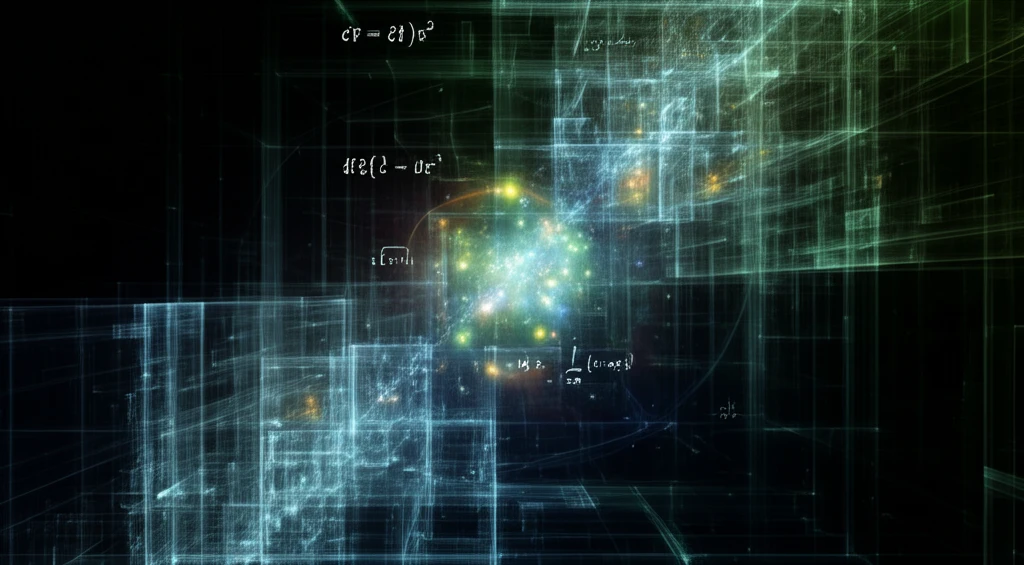
Before diving into particle representations, let's clarify what SPDEs are and why they are important. SPDEs are essentially extensions of ordinary differential equations (ODEs) and partial differential equations (PDEs) that incorporate randomness. In simpler terms, they describe how systems change over time and space when influenced by random noise.
- Differential Equations: Equations that relate a function to its derivatives. They are used to model how things change.
- Partial Differential Equations: Differential equations involving functions of several variables. These are used to model processes evolving in both time and space.
- Stochastic Elements: The inclusion of random noise or stochastic processes within the equation. This makes the equation more realistic for many real-world applications where perfect predictability is impossible.
The Future of SPDEs and Particle Representations
Particle representations offer a promising avenue for solving SPDEs, especially those with complex boundary conditions. Ongoing research continues to refine these methods, making them more efficient and applicable to a wider range of problems. As computational power increases, the use of particle representations is likely to become even more prevalent, providing valuable insights into the behavior of complex systems across various scientific and engineering disciplines.