Decoding Stochastic Modeling: How Randomness Shapes Our World
"From finance to ecology, stochastic models help us understand uncertainty. Discover practical applications and insights into this fascinating field of statistical modeling."
In our daily lives, we encounter a world brimming with uncertainty. From the fluctuations of the stock market to the unpredictable nature of weather patterns, randomness is an inherent aspect of many systems. To navigate and comprehend these complexities, scientists and researchers turn to stochastic modeling.
Stochastic modeling, at its core, is a mathematical approach designed to simulate systems influenced by randomness. Unlike deterministic models that predict outcomes with certainty, stochastic models incorporate probabilities and random variables to better reflect real-world uncertainty. This allows for a more nuanced and realistic analysis of various phenomena.
This article explores the world of stochastic modeling, focusing on its definition, diverse applications across multiple fields, and insights it offers into understanding and predicting complex systems. Whether you're a student, a professional, or simply someone curious about the power of statistical analysis, this guide will provide you with a comprehensive overview of how randomness shapes our world.
What is Stochastic Modeling?
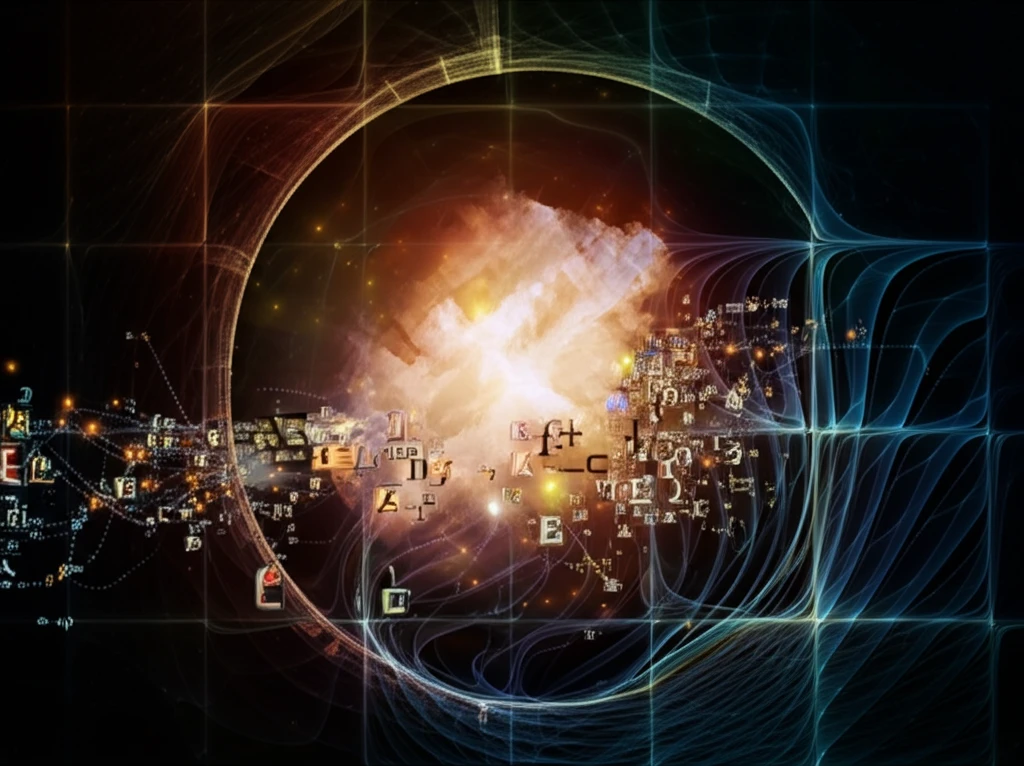
Stochastic modeling is a mathematical framework used to represent and analyze systems where randomness is a key factor. Traditional deterministic models assume that outcomes are predictable based on fixed inputs, stochastic models acknowledge that randomness plays a role in many real-world scenarios. The primary difference lies in how uncertainty is handled. Deterministic models ignore or simplify randomness, while stochastic models embrace it.
- Probabilities: Represent the likelihood of different events occurring within the system.
- Random Variables: Variables whose values are determined by a random process.
- Simulations: Running multiple simulations helps assess the range of potential outcomes and their likelihoods.
The Future of Stochastic Modeling
Stochastic modeling is continually evolving. As computational power increases and data becomes more readily available, so too does its relevance in addressing complex problems. Future research will likely focus on developing more sophisticated algorithms, integrating machine learning techniques, and expanding applications across emerging fields. Embracing uncertainty and harnessing the power of randomness will be crucial for navigating an increasingly complex and interconnected world. By understanding and applying stochastic modeling, we can gain valuable insights, make informed decisions, and better prepare for whatever the future may hold.