Decoding Skin Cancer: How AI and TensorFlow Are Changing Detection
"A look at how deep learning is improving skin cancer detection accuracy and potentially saving lives."
Skin cancer is a growing global health concern, demanding accurate and timely detection. While the human eye can easily distinguish between similar-looking images, classifying skin images affected by cancer requires specialized expertise. The rising incidence of skin cancer necessitates more trained professionals, which poses a significant challenge.
To address this issue, researchers are increasingly focused on developing machine learning classifiers capable of automatically detecting skin cancer through image analysis. This paper delves into an innovative approach for predicting skin cancer by classifying images using deep convolutional neural networks (DCNN).
The study leverages TensorFlow and the inception v3 model, testing its performance on standard cancer datasets. The results demonstrate a promising accuracy rate exceeding 85%, highlighting the potential of AI in revolutionizing skin cancer diagnostics.
The Power of Deep Convolutional Neural Networks (DCNNs) in Skin Cancer Detection
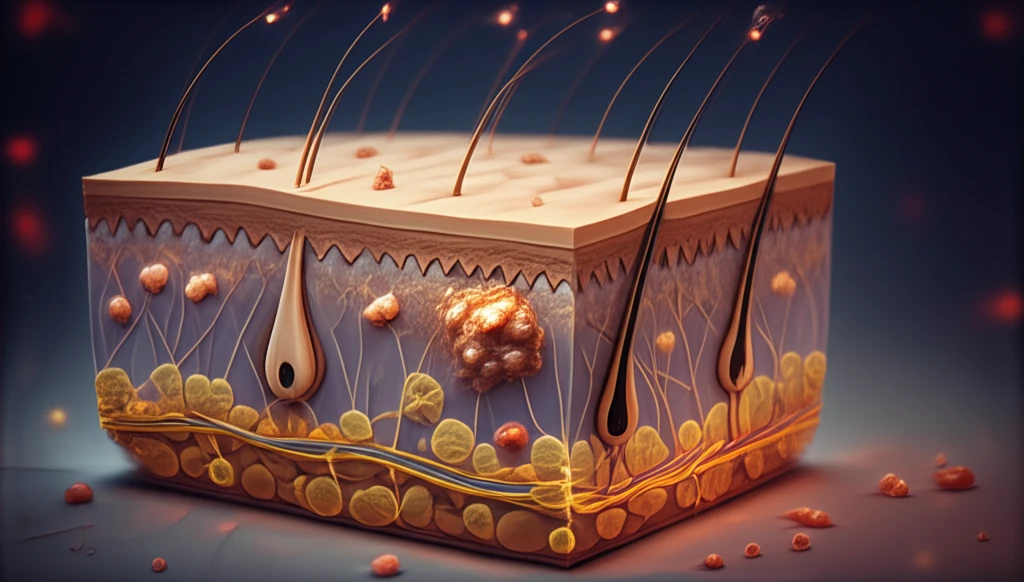
Deep Convolutional Neural Networks (DCNNs) offer a promising avenue for improving skin cancer detection. DCNNs, a machine learning classification technique, excel in classifying skin cancer images with enhanced precision. By training the network with a vast number of images and increasing the number of iterations, the accuracy of DCNNs can be significantly enhanced. The key is enabling the network to learn intricate patterns and features indicative of cancerous formations.
- DCNN Advantages: DCNNs automatically learn hierarchical features from images, reducing the need for manual feature extraction.
- TensorFlow Benefits: TensorFlow supports distributed computing, enabling faster training on large datasets.
- Inception V3 Architecture: Inception V3 employs a modular architecture that optimizes both speed and accuracy.
The Future of AI in Early Skin Cancer Detection
The application of DCNNs using TensorFlow and inception V3 presents a significant advancement in skin cancer detection. Achieving over 85% accuracy on standard datasets demonstrates the potential of AI to enhance diagnostic precision and efficiency. The ongoing development and refinement of these technologies promise a future where early and accurate skin cancer detection becomes more accessible, ultimately improving patient outcomes and saving lives.