Decoding RNA Sequencing: A Beginner's Guide to Powerful Study Designs
"Unlock the secrets of RNA-Seq: Learn how to design effective studies, calculate power, and maximize your research budget for optimal results."
In the rapidly evolving world of genomics, RNA sequencing (RNA-Seq) has emerged as a powerful technique for understanding the complexities of gene expression. This technology allows researchers to monitor the global transcriptomic landscape, providing insights into various biological processes and disease mechanisms. While the cost of RNA-Seq experiments has decreased significantly, the financial investment and bioinformatic challenges remain considerable hurdles for many biomedical projects.
Unlike traditional methods like microarrays, RNA-Seq data requires careful consideration of discrete count data and sequencing depth. Designing an effective RNA-Seq study involves more than just determining the sample size; it requires a comprehensive strategy that balances sequencing depth with the overall budget. The key is to maximize the information gained while keeping costs manageable.
This article provides a practical framework for navigating the complexities of RNA-Seq study design. By demystifying the concepts of power calculation, cost-benefit analysis, and optimal resource allocation, we empower researchers to design robust and informative experiments. Whether you're a seasoned genomics expert or just starting out, this guide offers actionable insights to help you unlock the full potential of RNA-Seq.
Why is RNA-Seq Study Design So Complex?
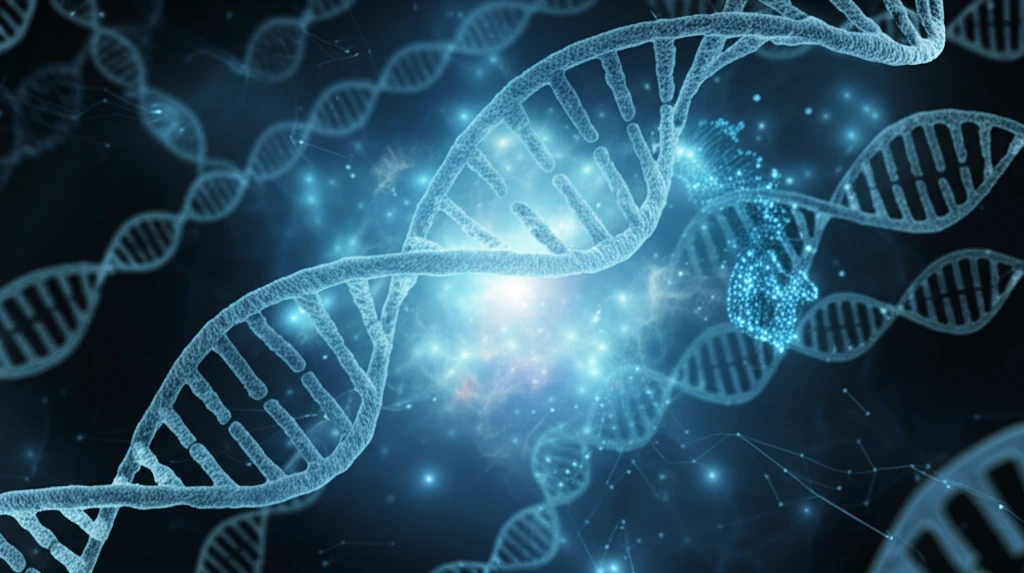
Traditional power calculations typically consider the relationship between effect size, statistical power (1 - type II error), and sample size. However, in RNA-Seq experiments, the sheer volume of data generated introduces unique challenges.
- Multiple Comparisons: RNA-Seq experiments involve testing thousands of hypotheses simultaneously, necessitating stringent control of type I error rates (false positives). Methods like Family-Wise Error Rate (FWER) and False Discovery Rate (FDR) are crucial.
- Sequencing Depth: The depth of sequencing (number of reads per sample) directly impacts the ability to detect differentially expressed genes. Balancing sequencing depth with sample size within a fixed budget is a complex optimization problem.
- Data Distribution: Unlike microarray data, RNA-Seq data consists of discrete counts, requiring statistical models that account for this unique characteristic. The negative binomial model has become a popular choice for analyzing RNA-Seq data.
- Expression Skewness: The distribution of gene expression levels is often skewed, with a small proportion of highly expressed genes dominating the sequencing reads. This can lead to detection bias for genes with low expression levels.
The Future of RNA-Seq Study Design
As sequencing costs continue to decline and RNA-Seq technology becomes increasingly accessible, thoughtful study planning will be more critical than ever. By embracing statistical frameworks like RNASeqDesign and carefully considering the interplay of various factors, researchers can maximize the value of their experiments and gain deeper insights into the complexities of the transcriptome.The landscape of genomic research is evolving, so adapting your approach to study design is essential for success.